Lecture Note 11 - Critical Thinking | Trường Đại học Quốc tế, Đại học Quốc gia Thành phố HCM
Lecture Note 11 - Critical Thinking | Trường Đại học Quốc tế, Đại học Quốc gia Thành phố HCM được sưu tầm và soạn thảo dưới dạng file PDF để gửi tới các bạn sinh viên cùng tham khảo, ôn tập đầy đủ kiến thức, chuẩn bị cho các buổi học thật tốt. Mời bạn đọc đón xem!
Môn: Critical thinking
Trường: Trường Đại học Quốc tế, Đại học Quốc gia Thành phố Hồ Chí Minh
Thông tin:
Tác giả:
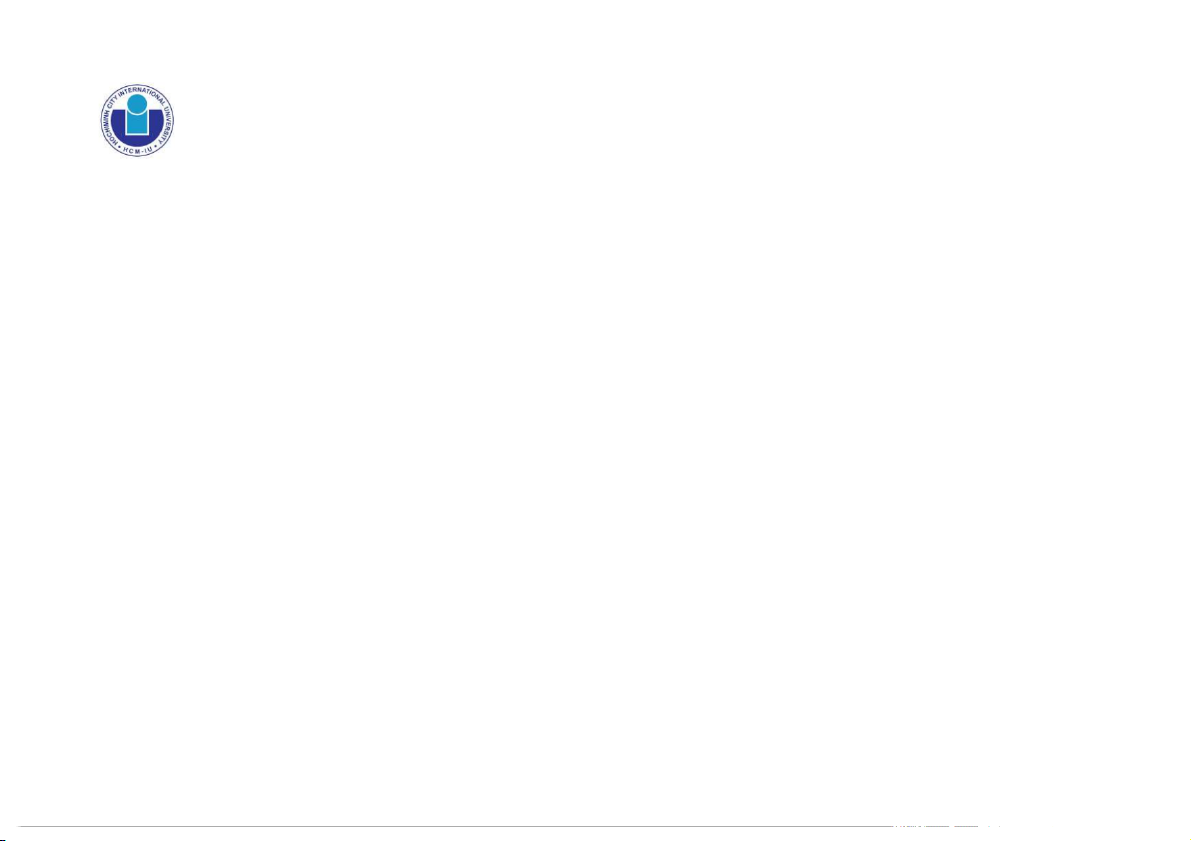
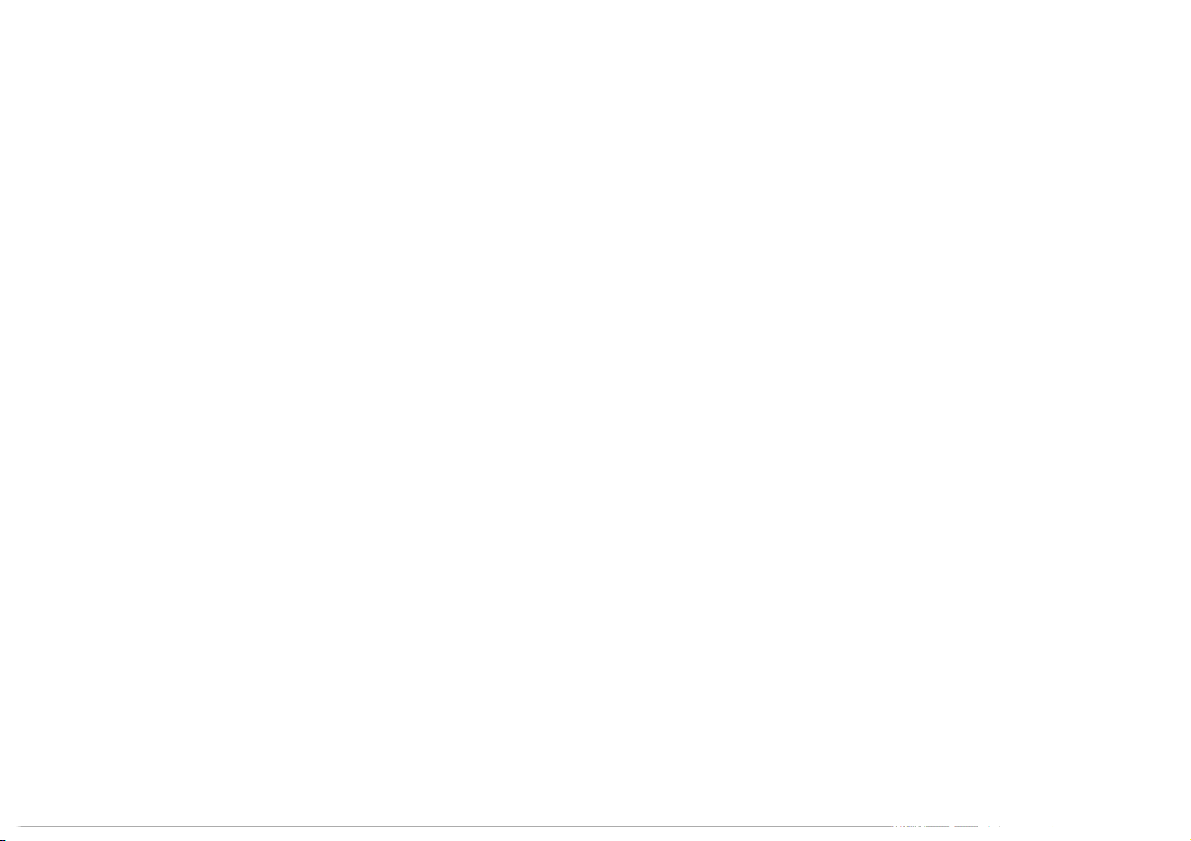
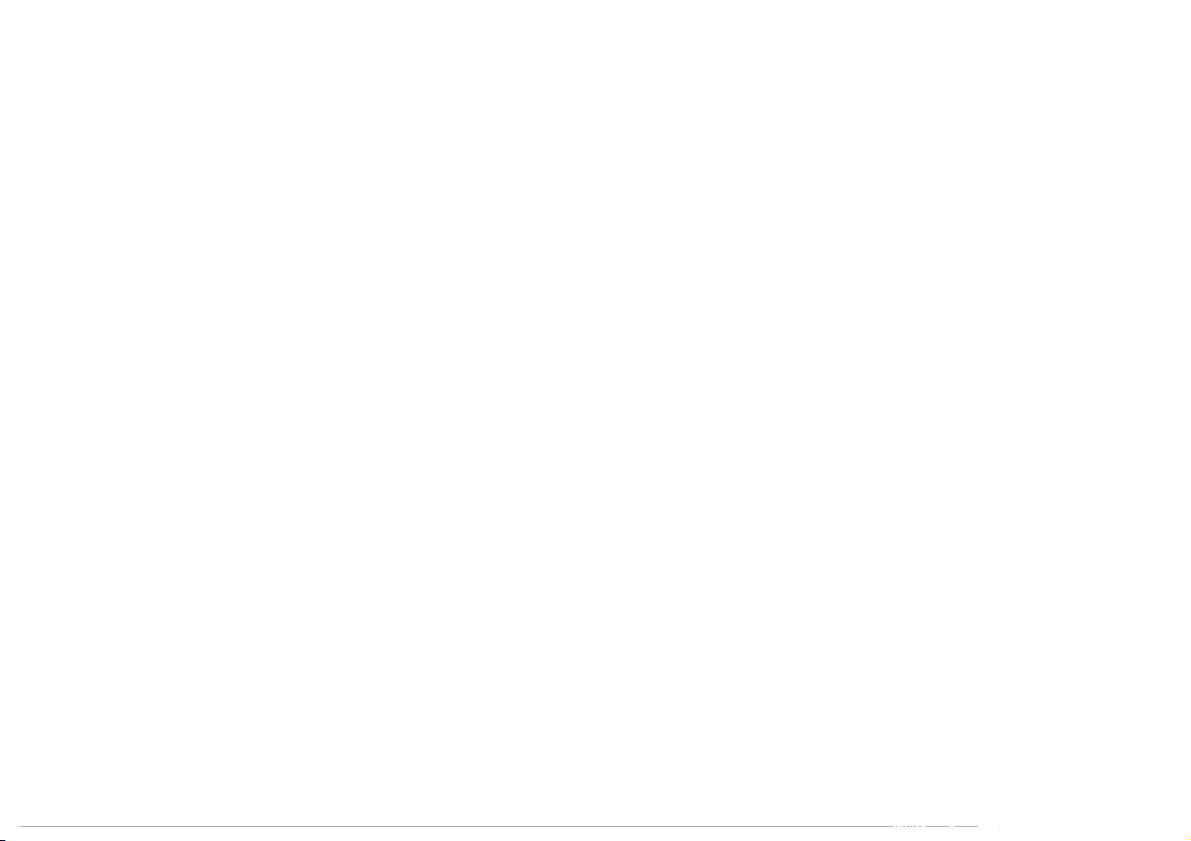
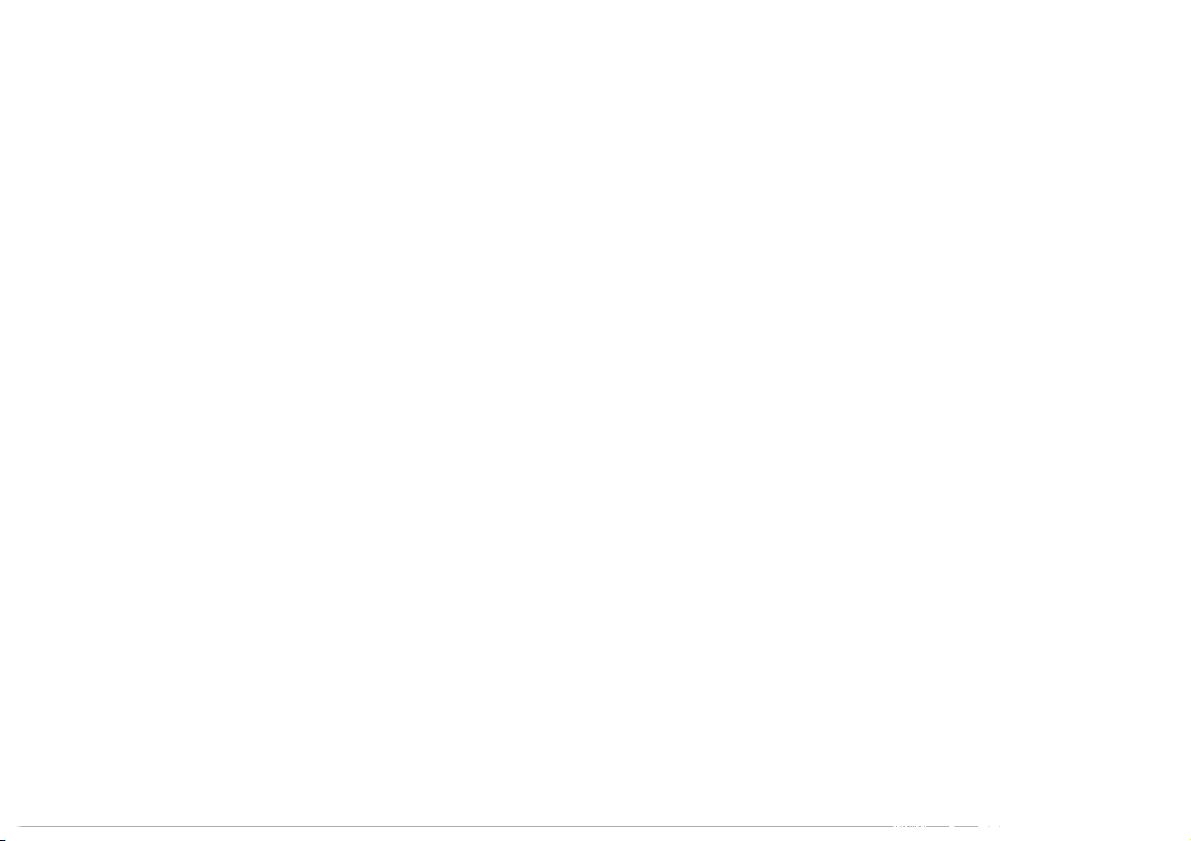
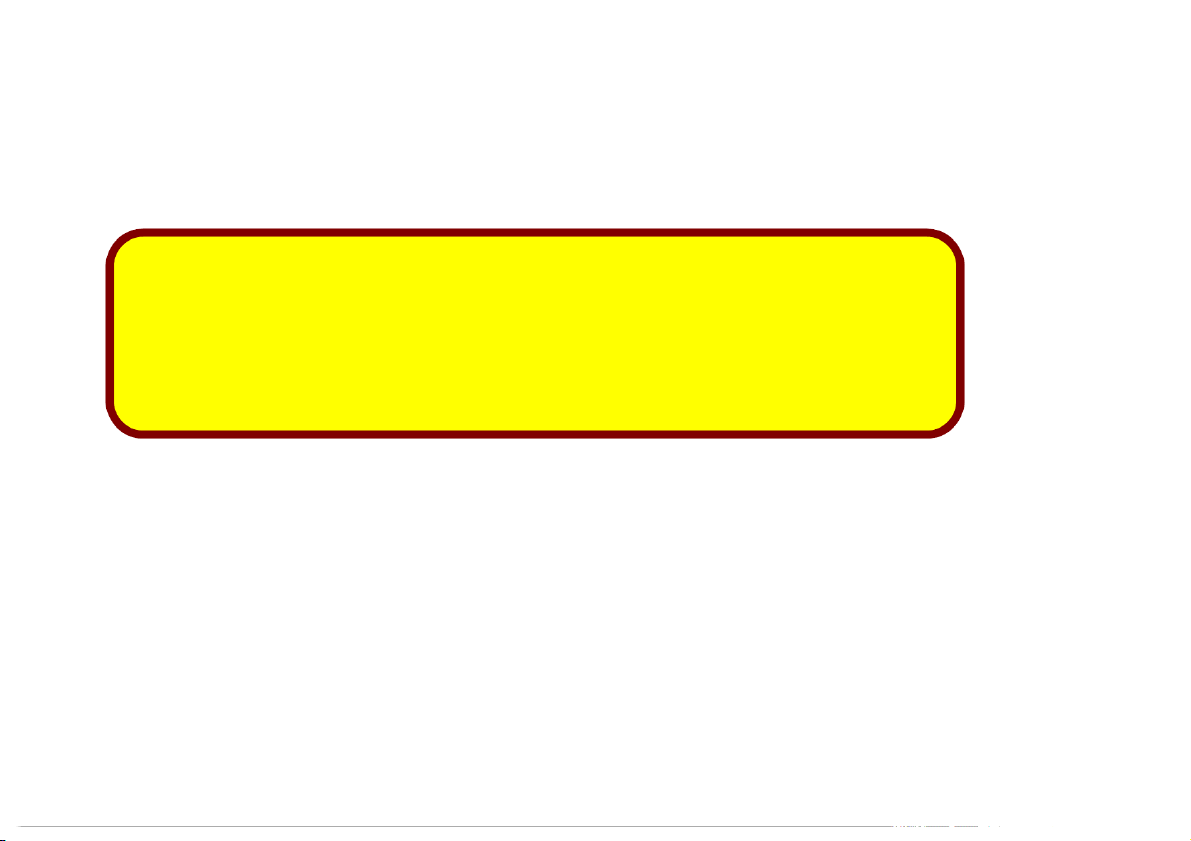
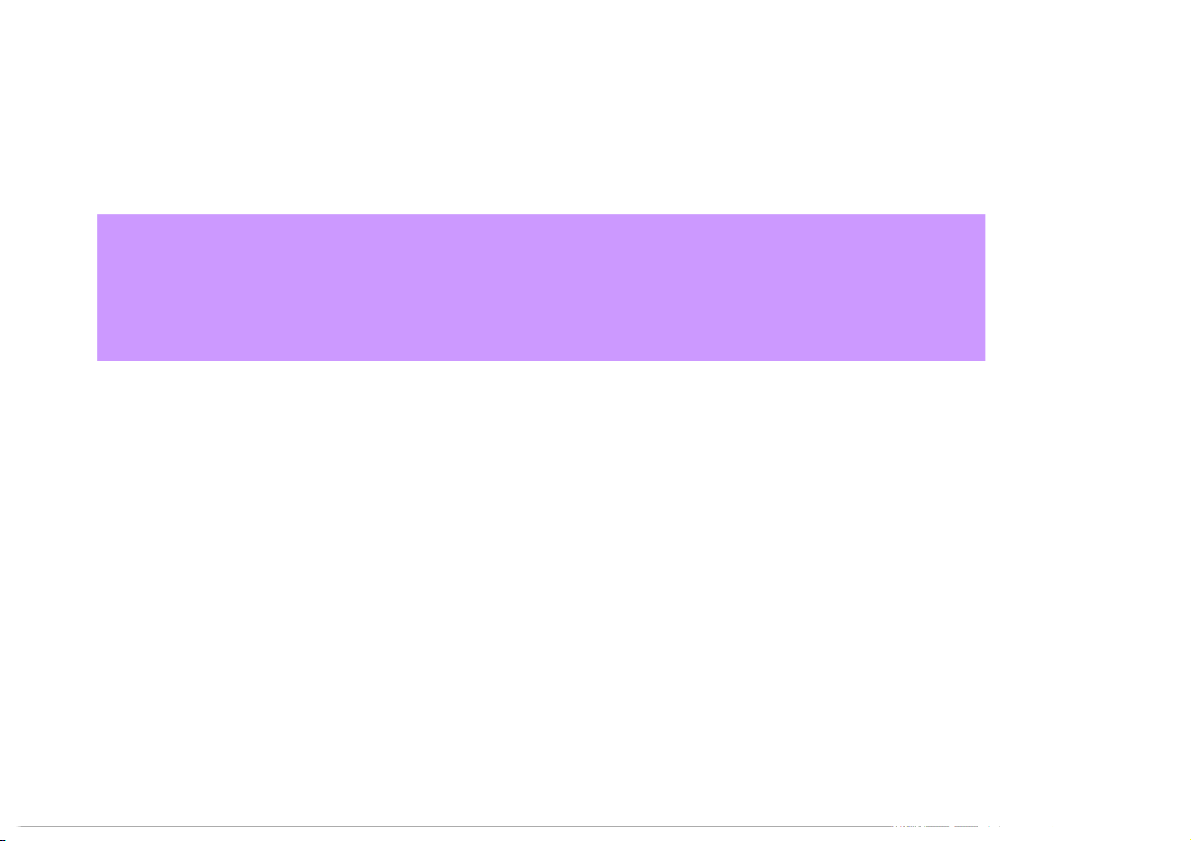
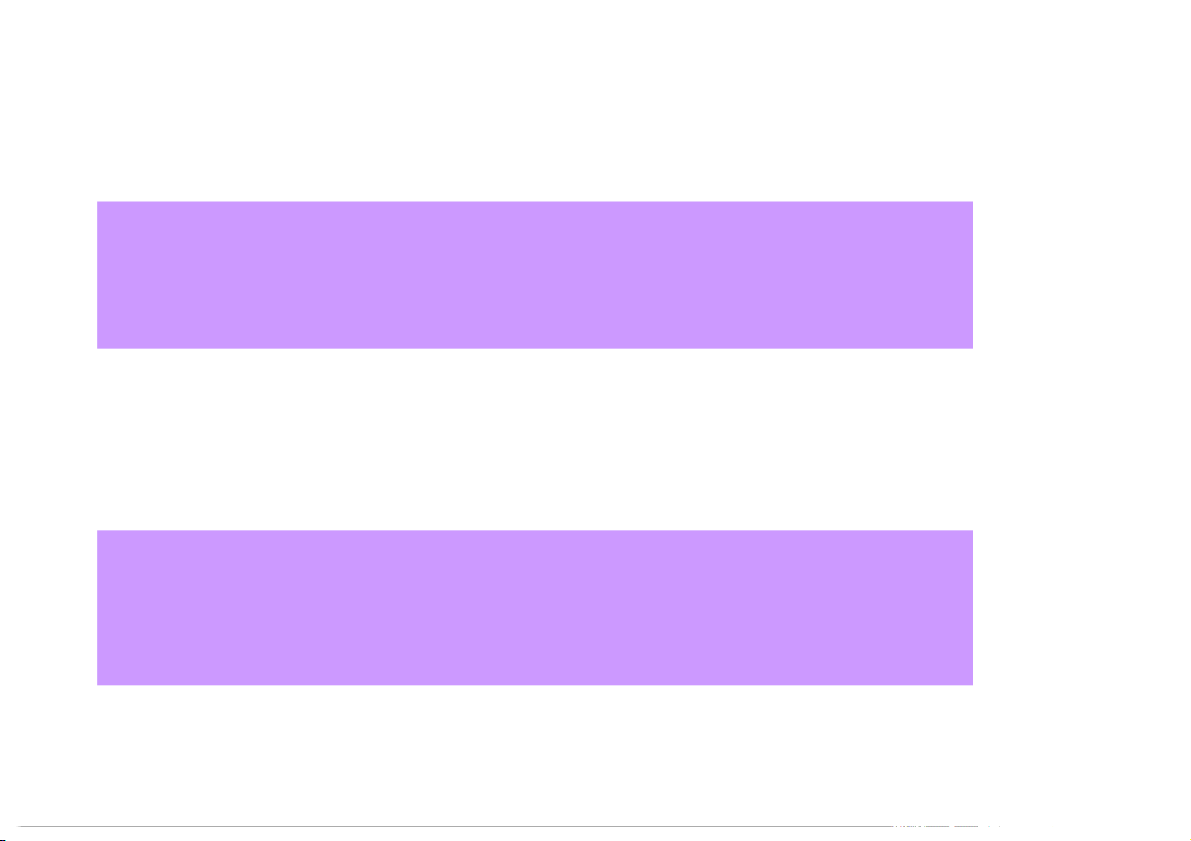
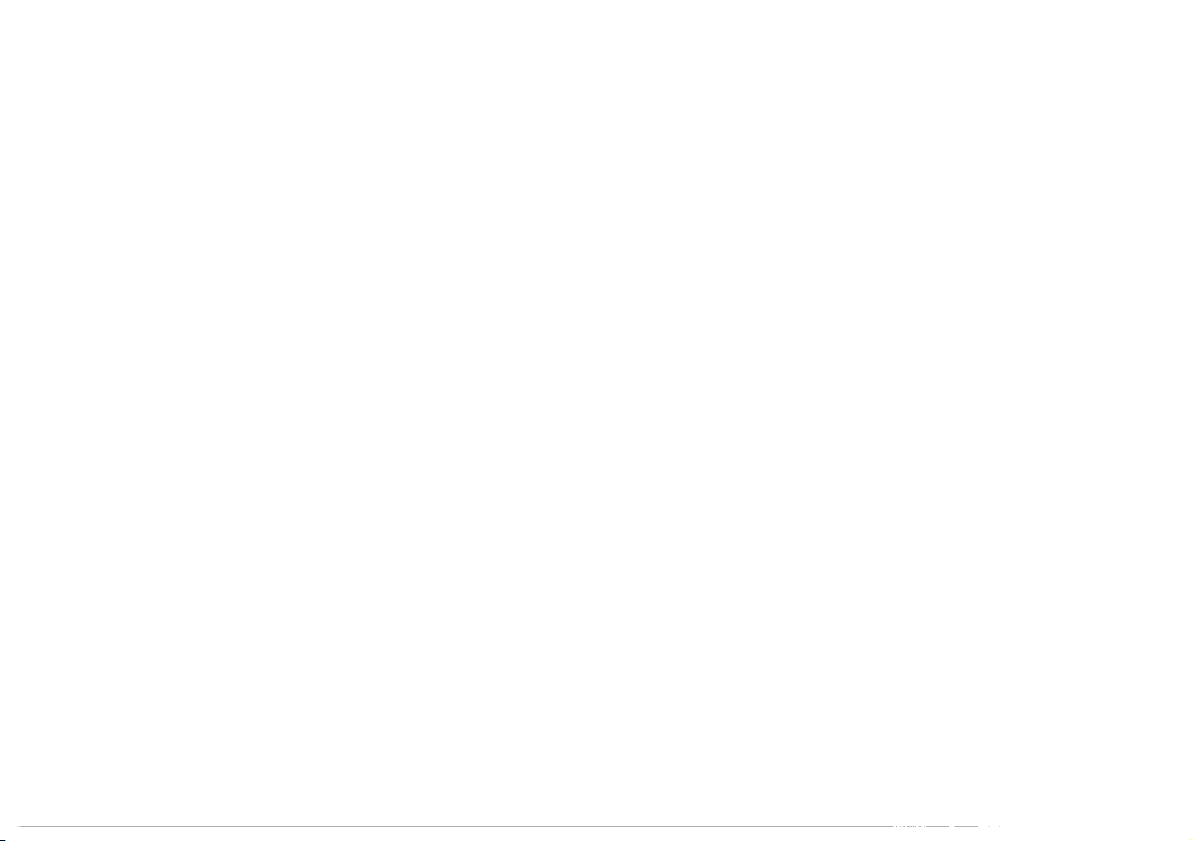
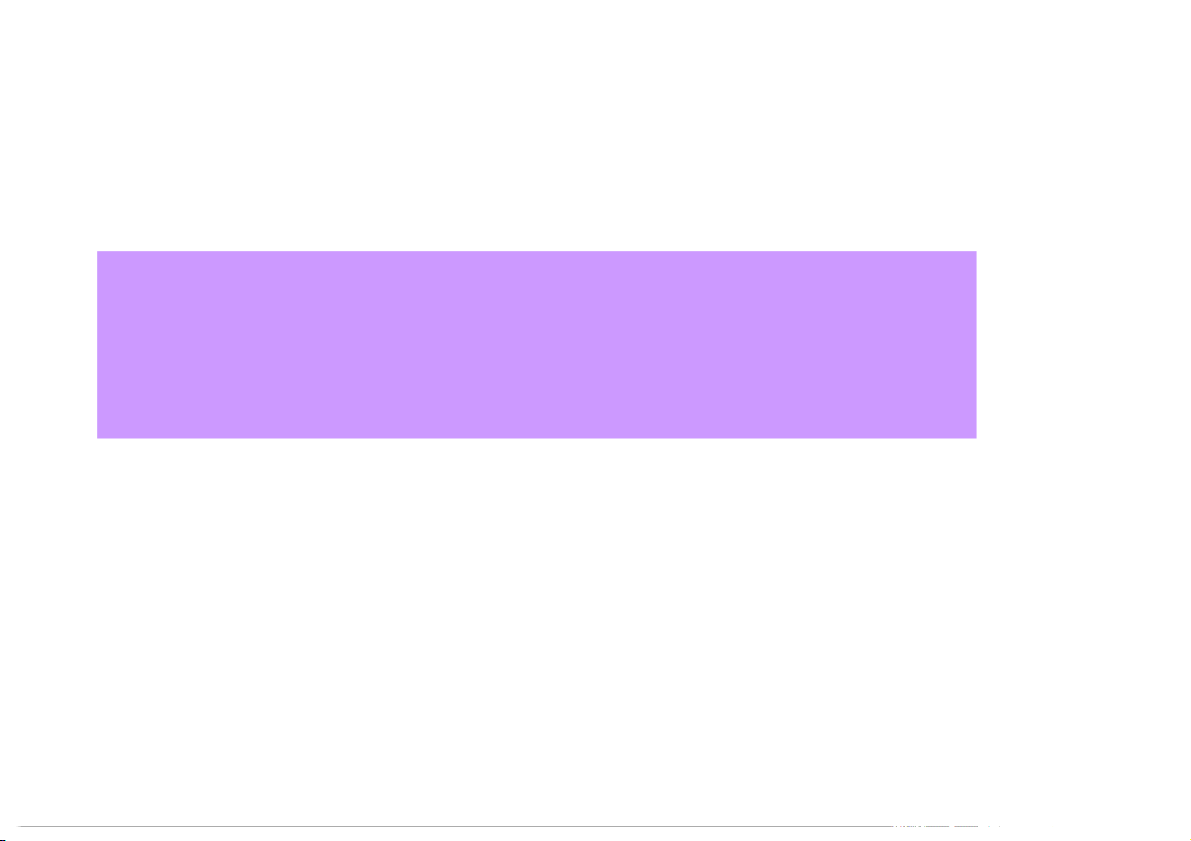
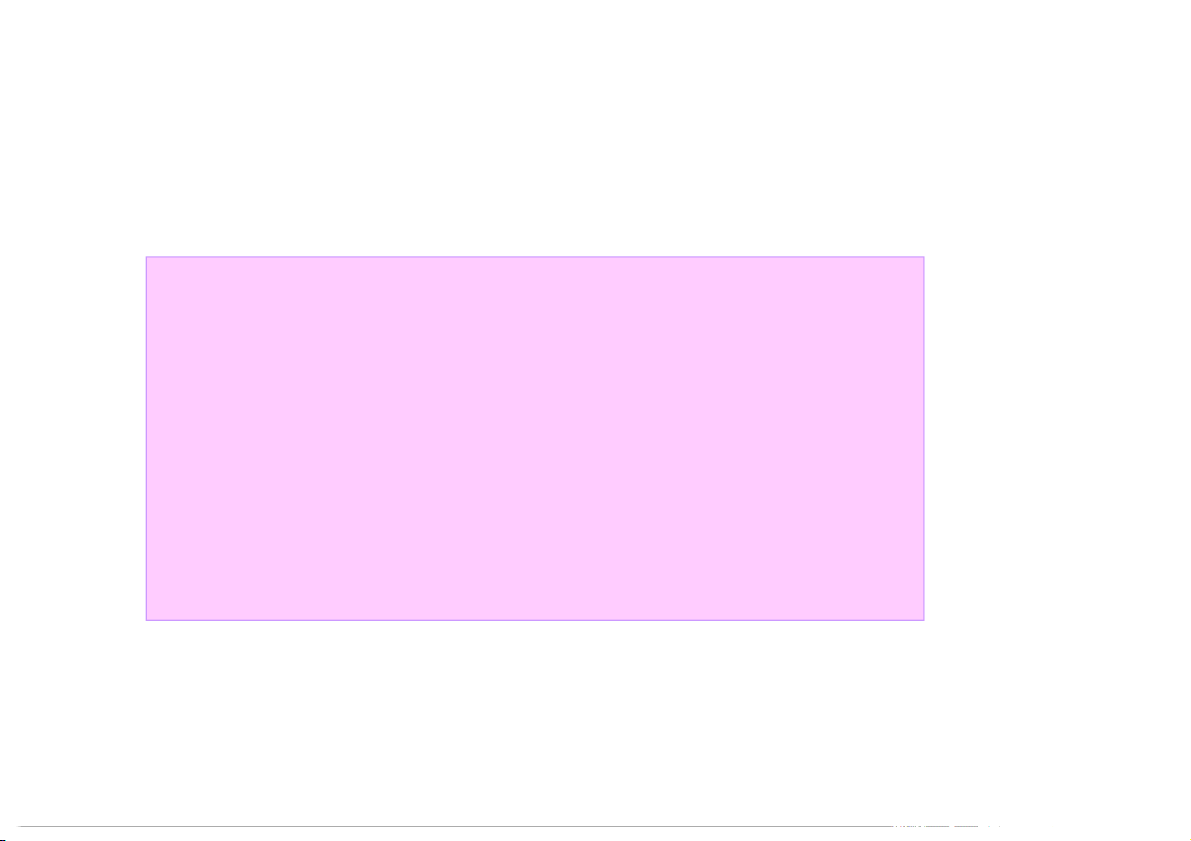
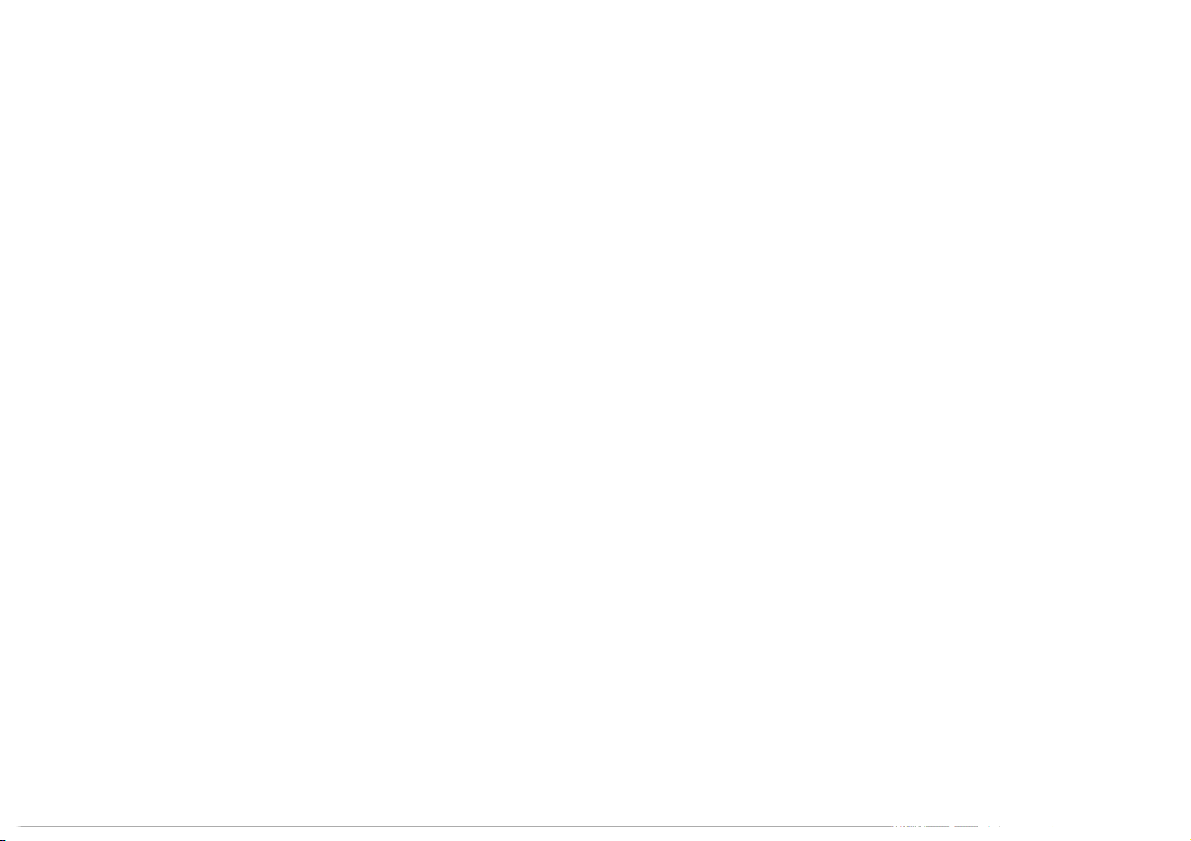
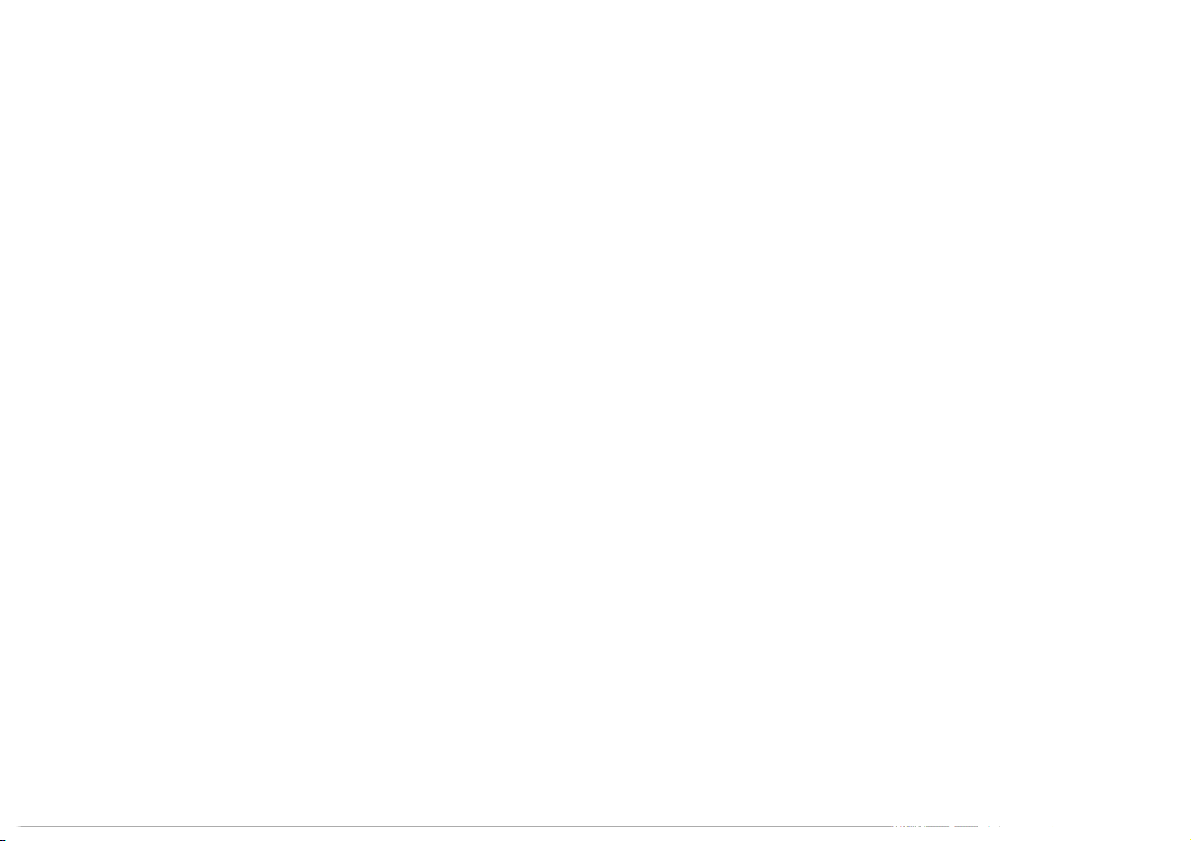
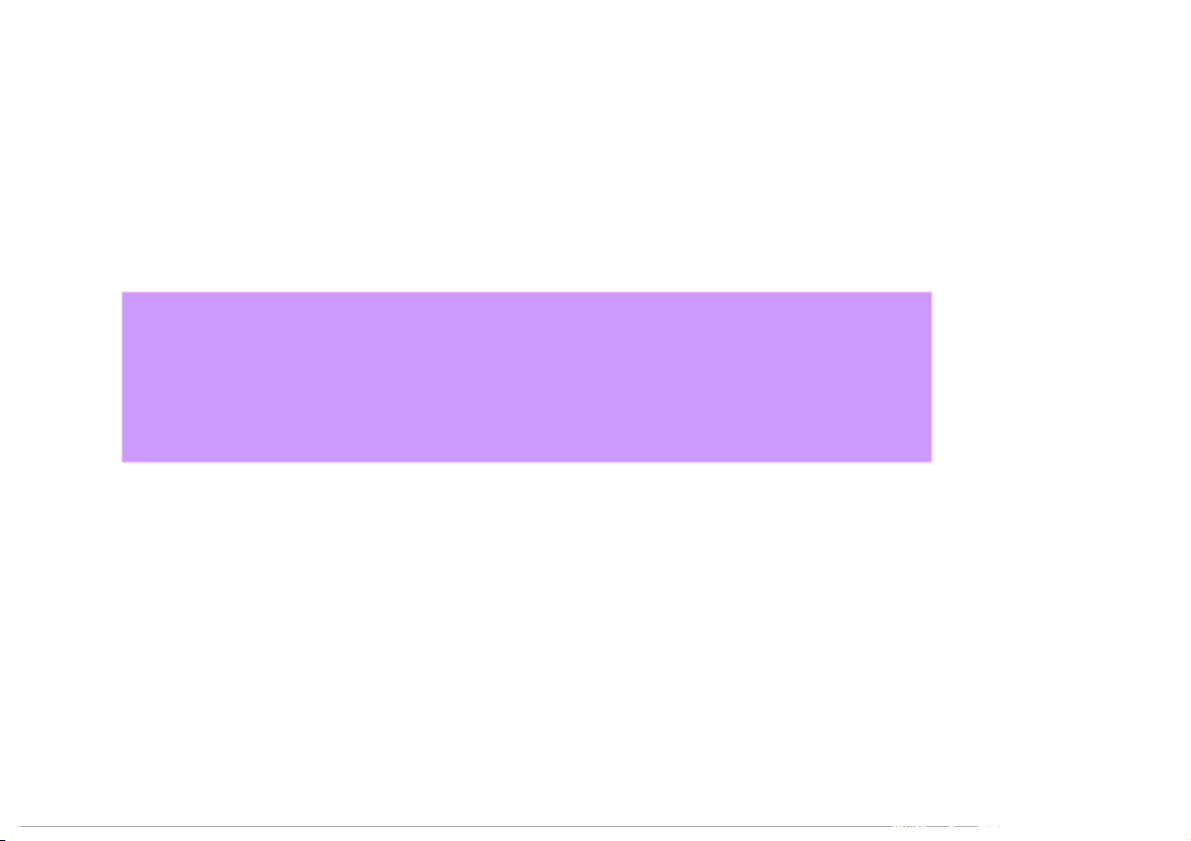
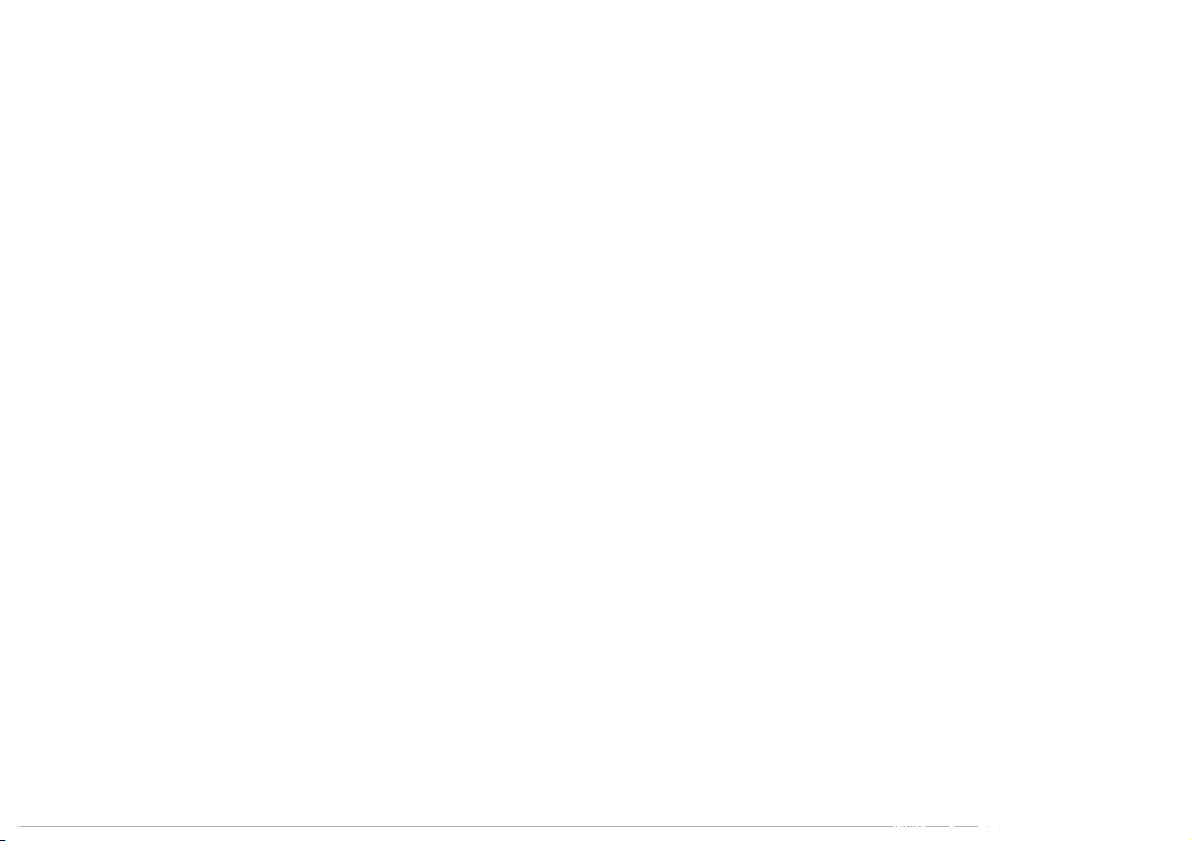
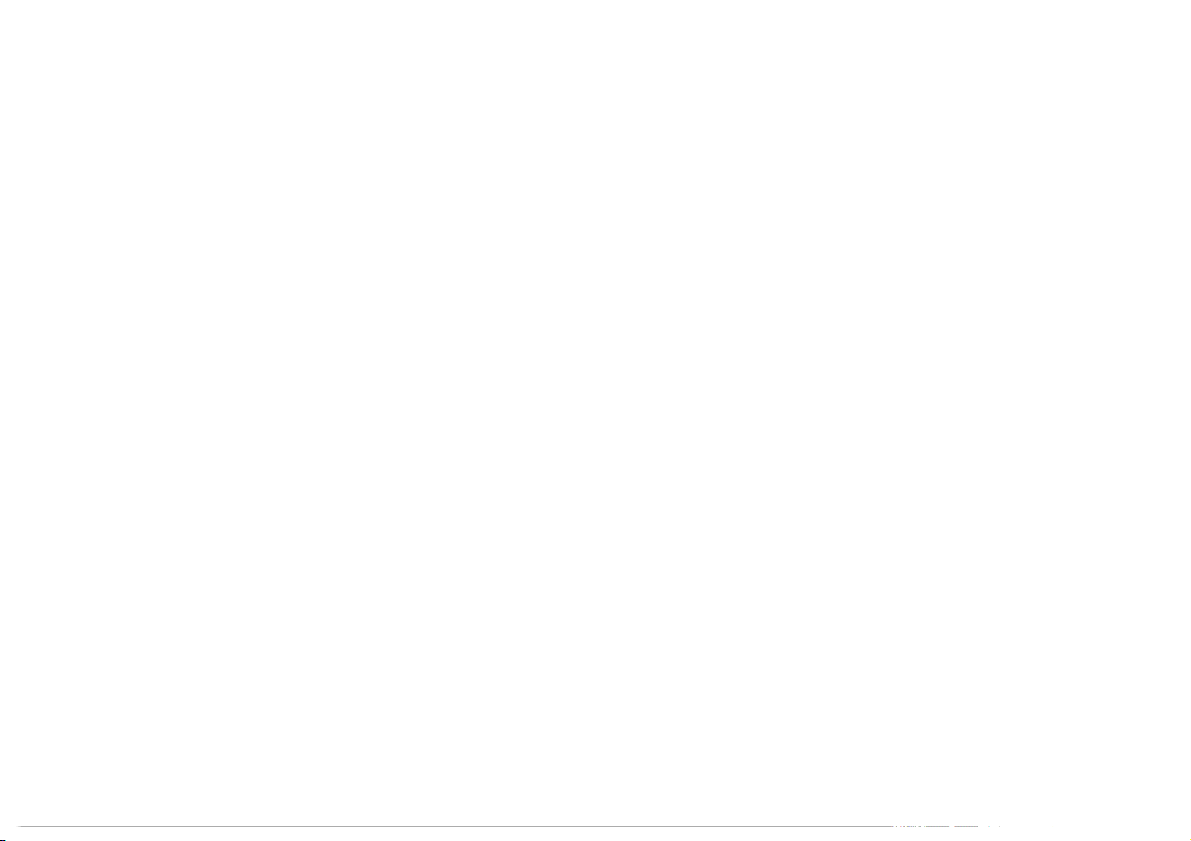
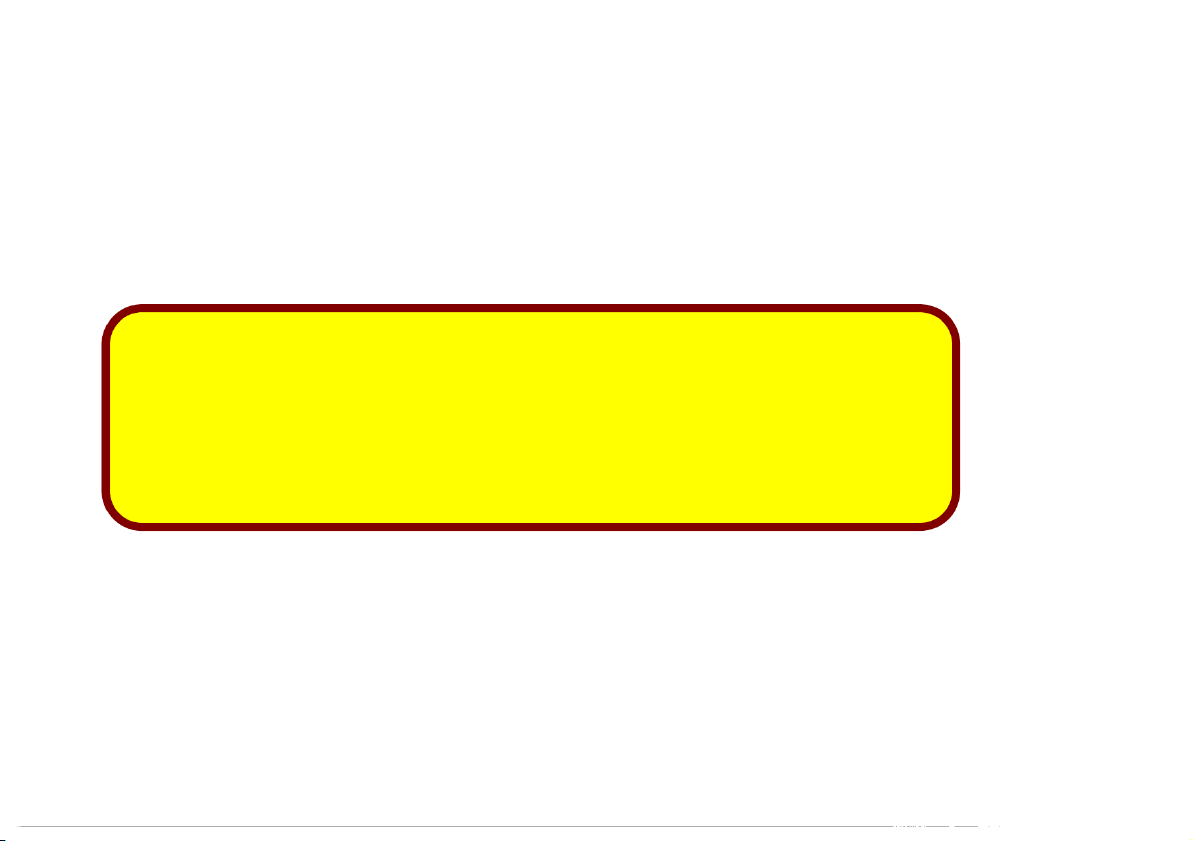
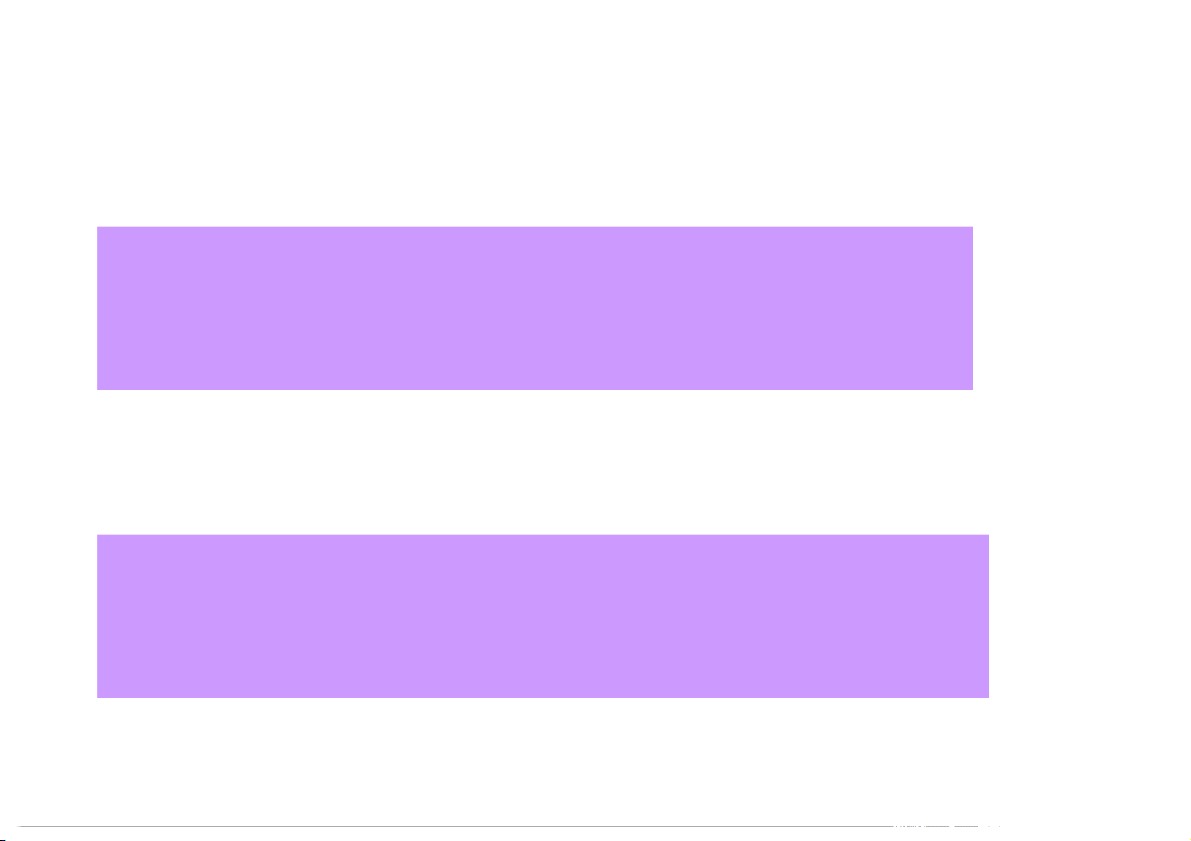
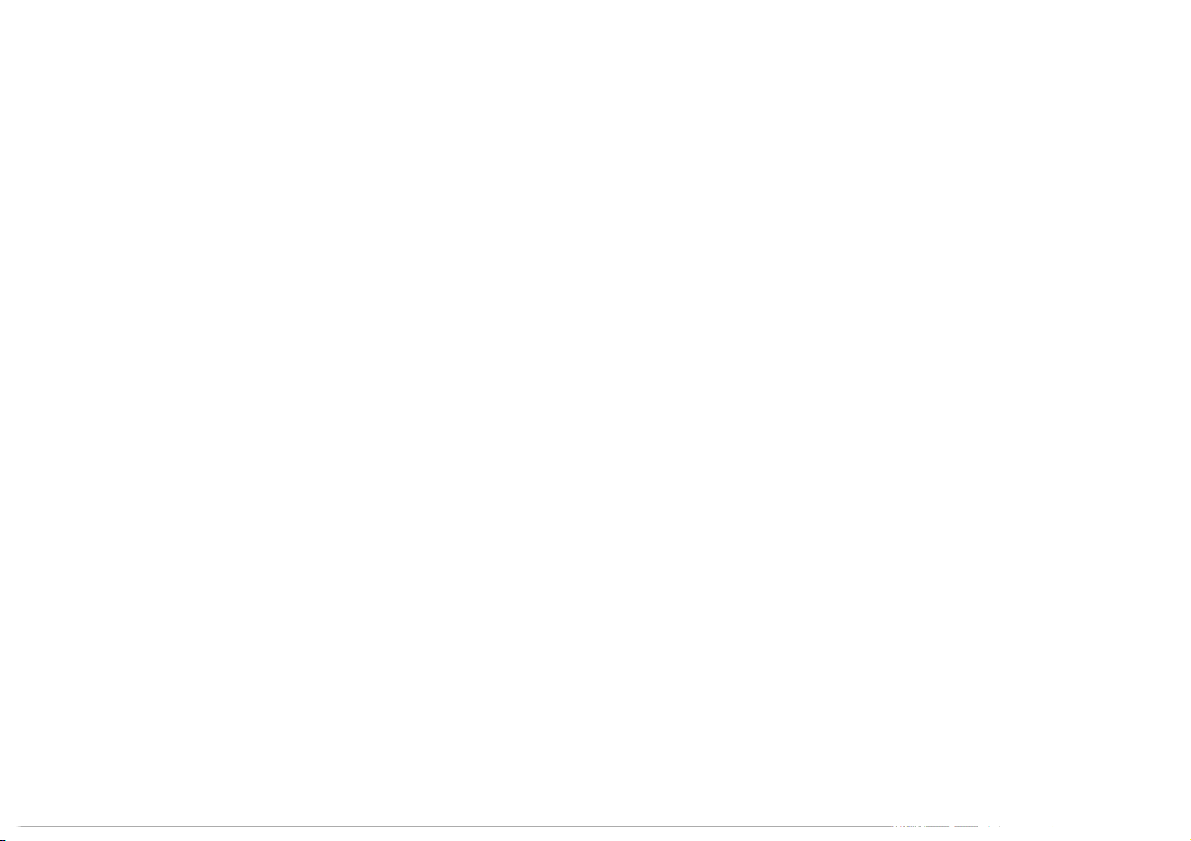
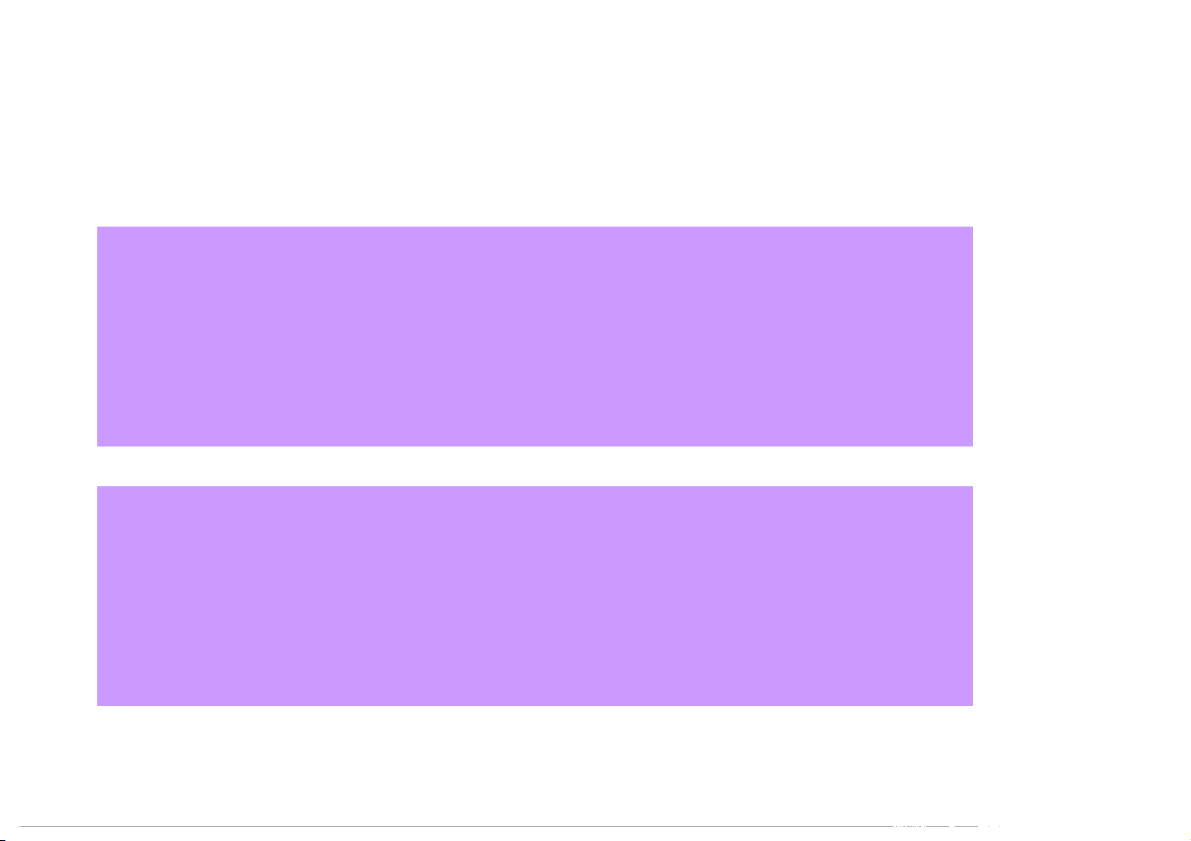
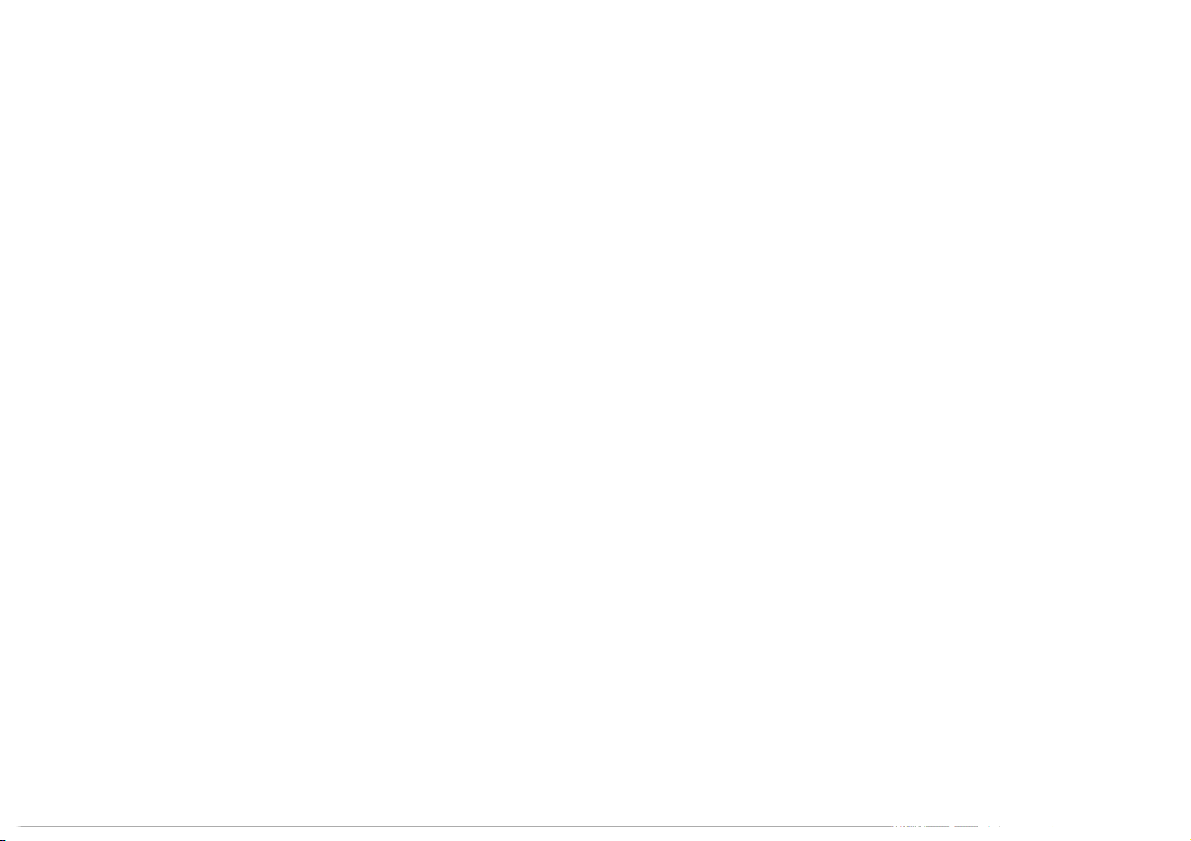
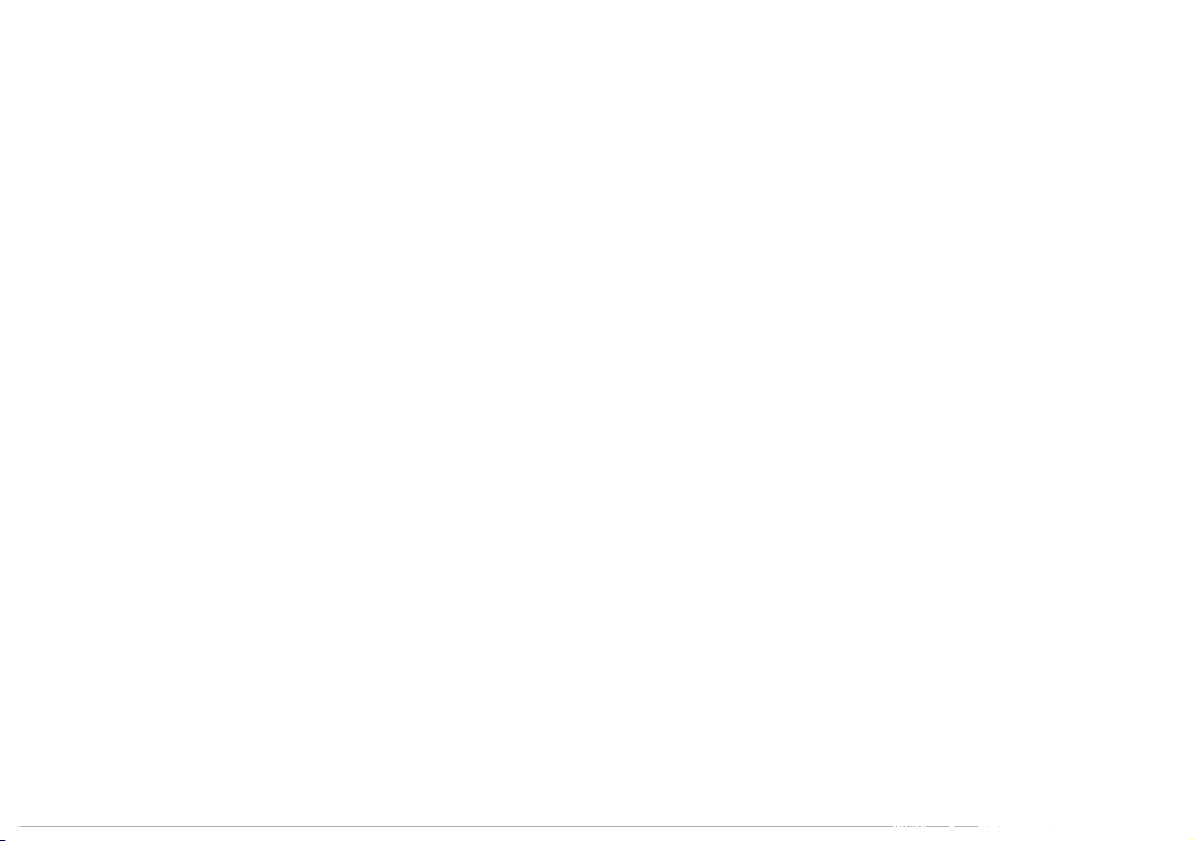
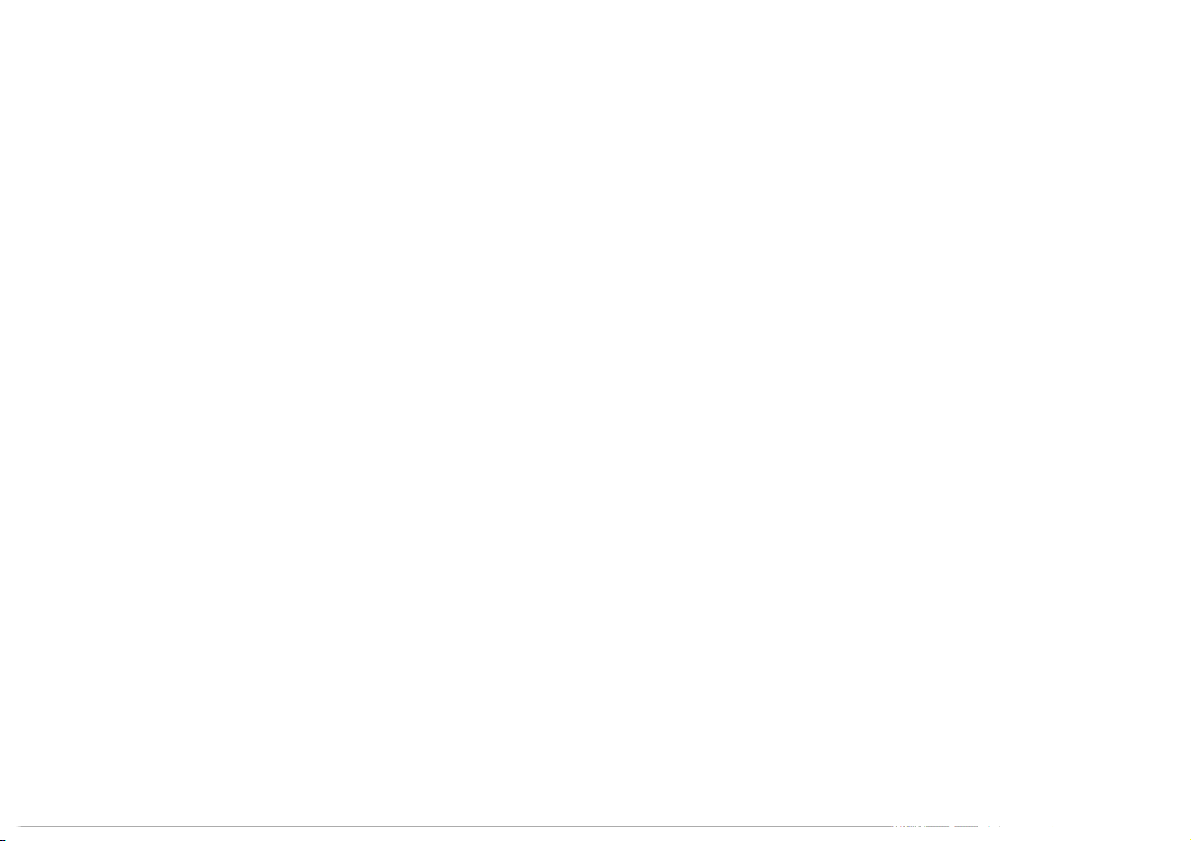
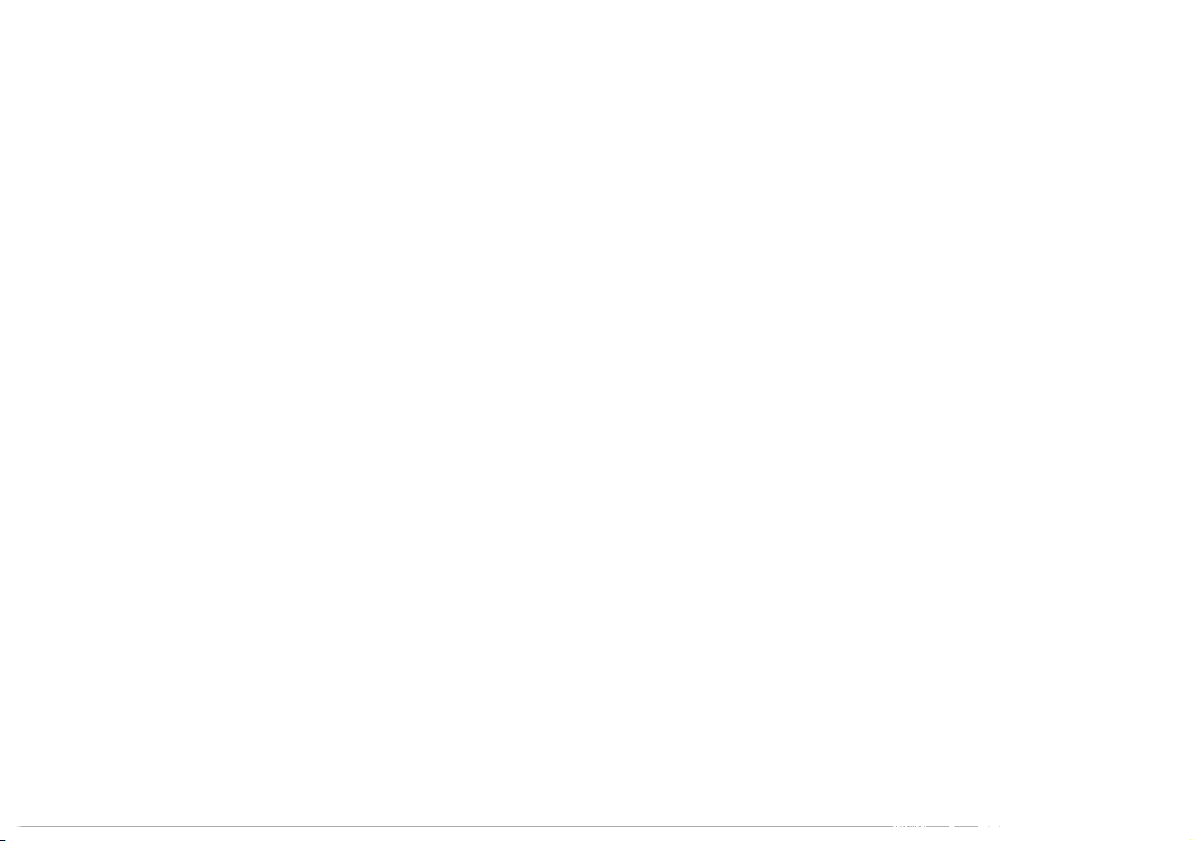
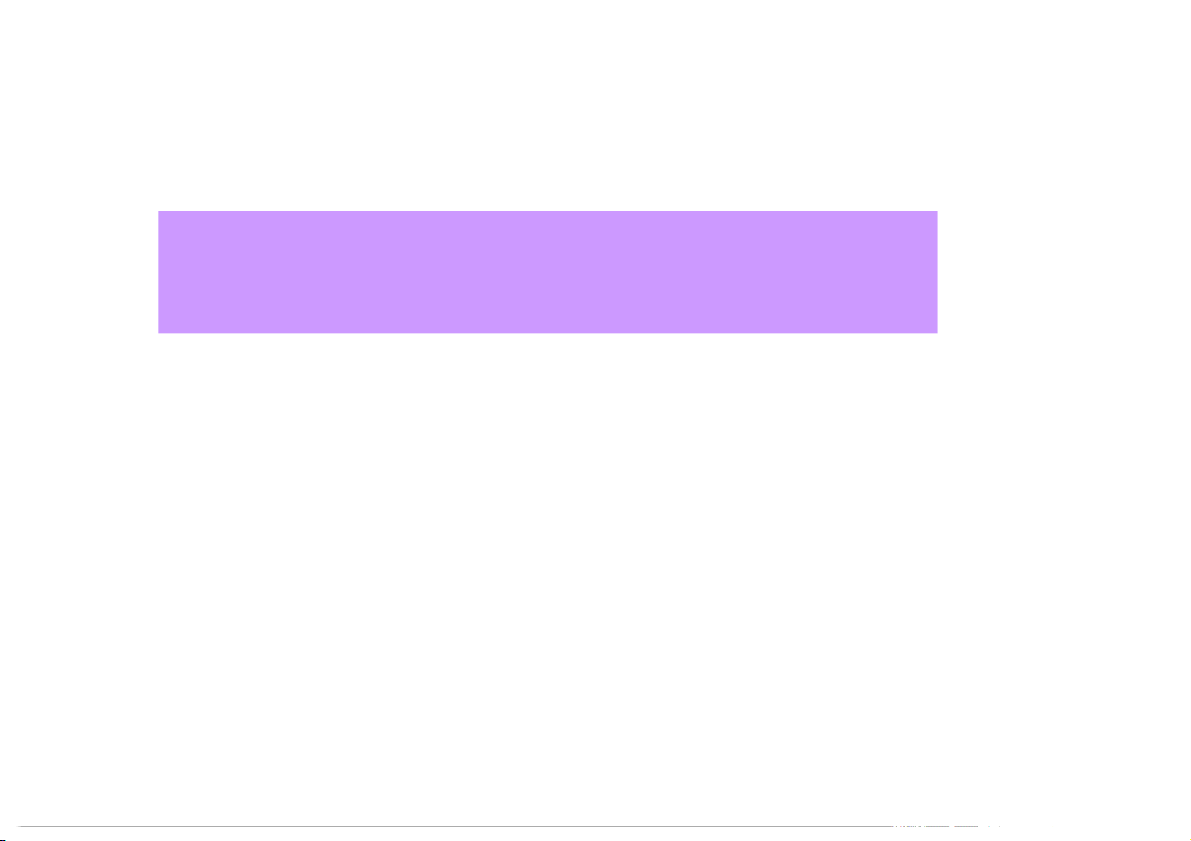
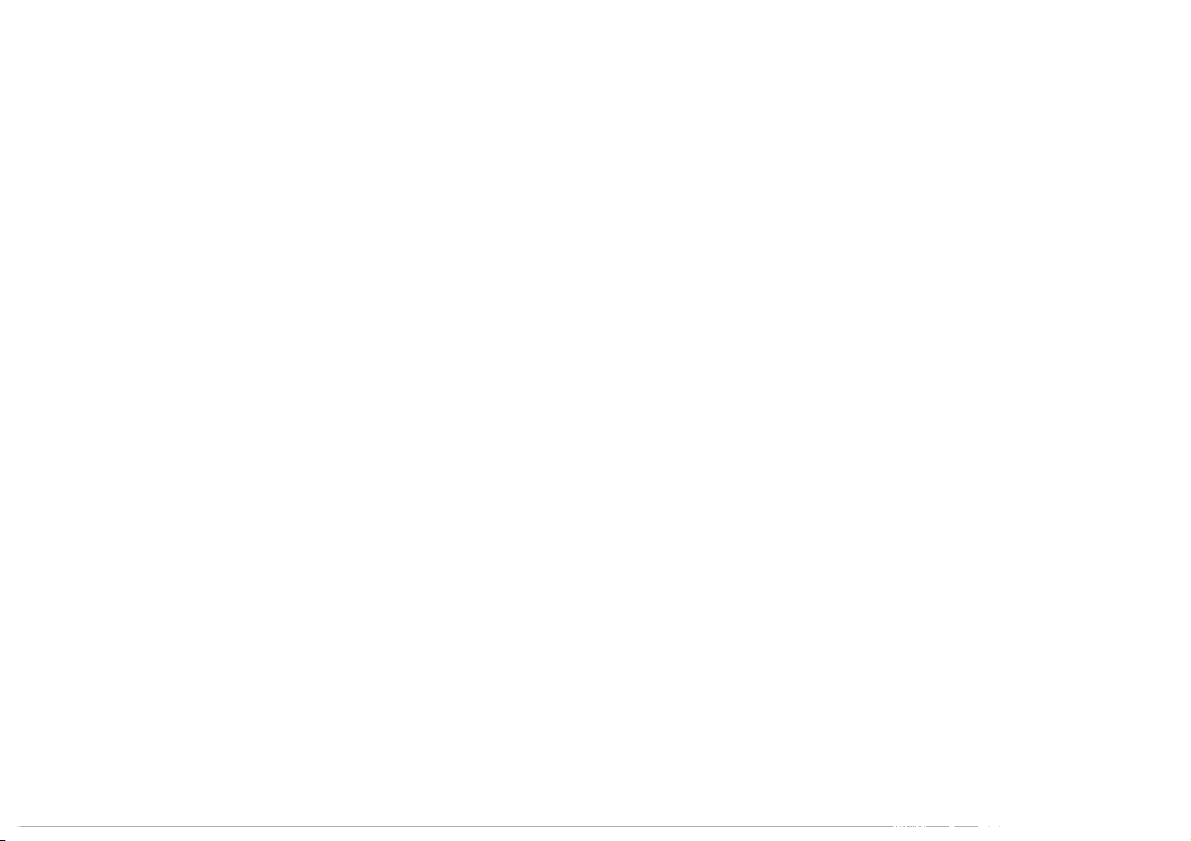
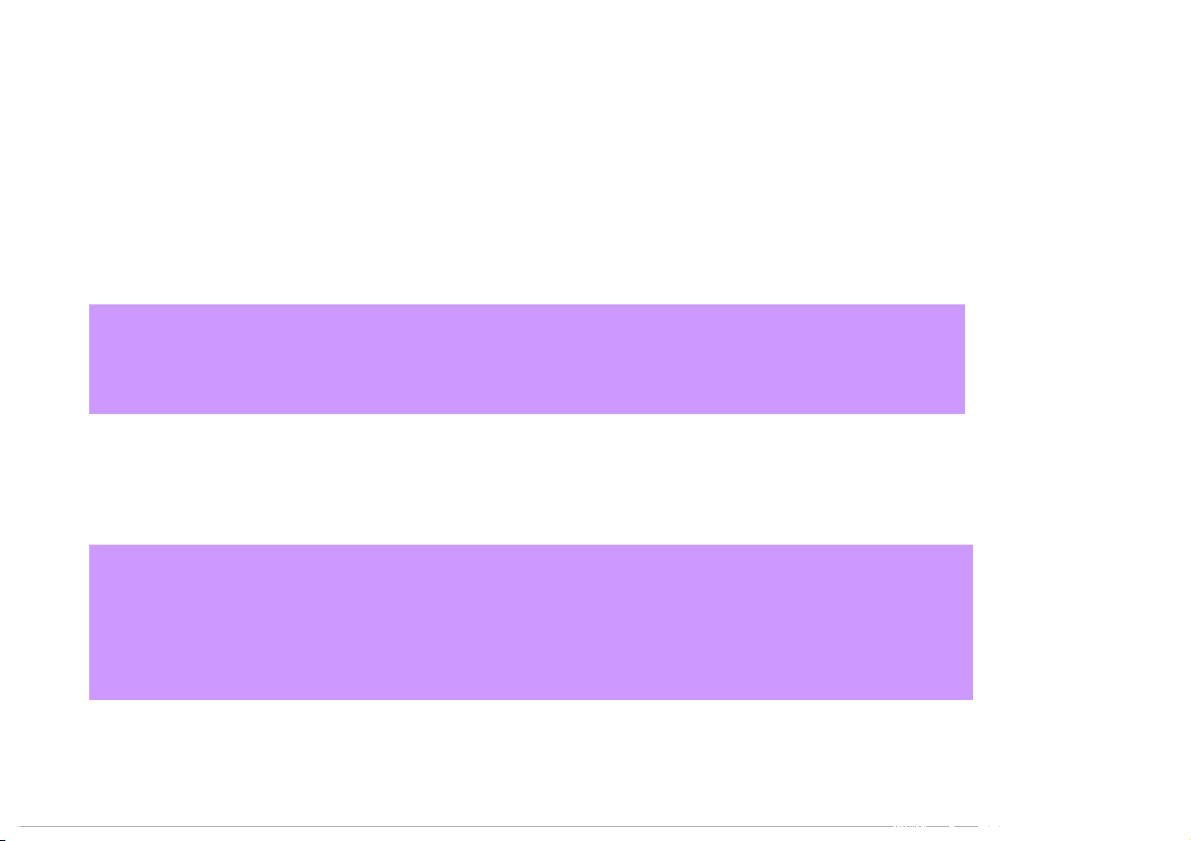
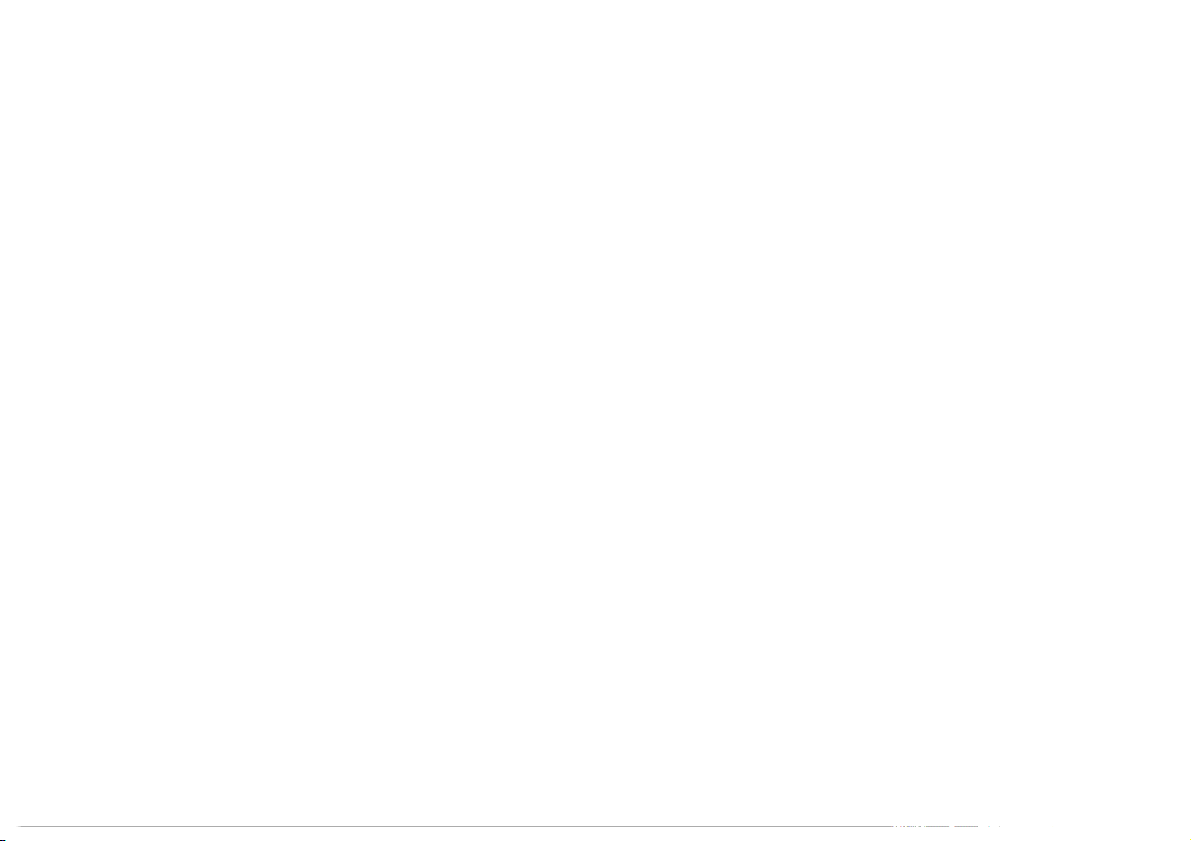
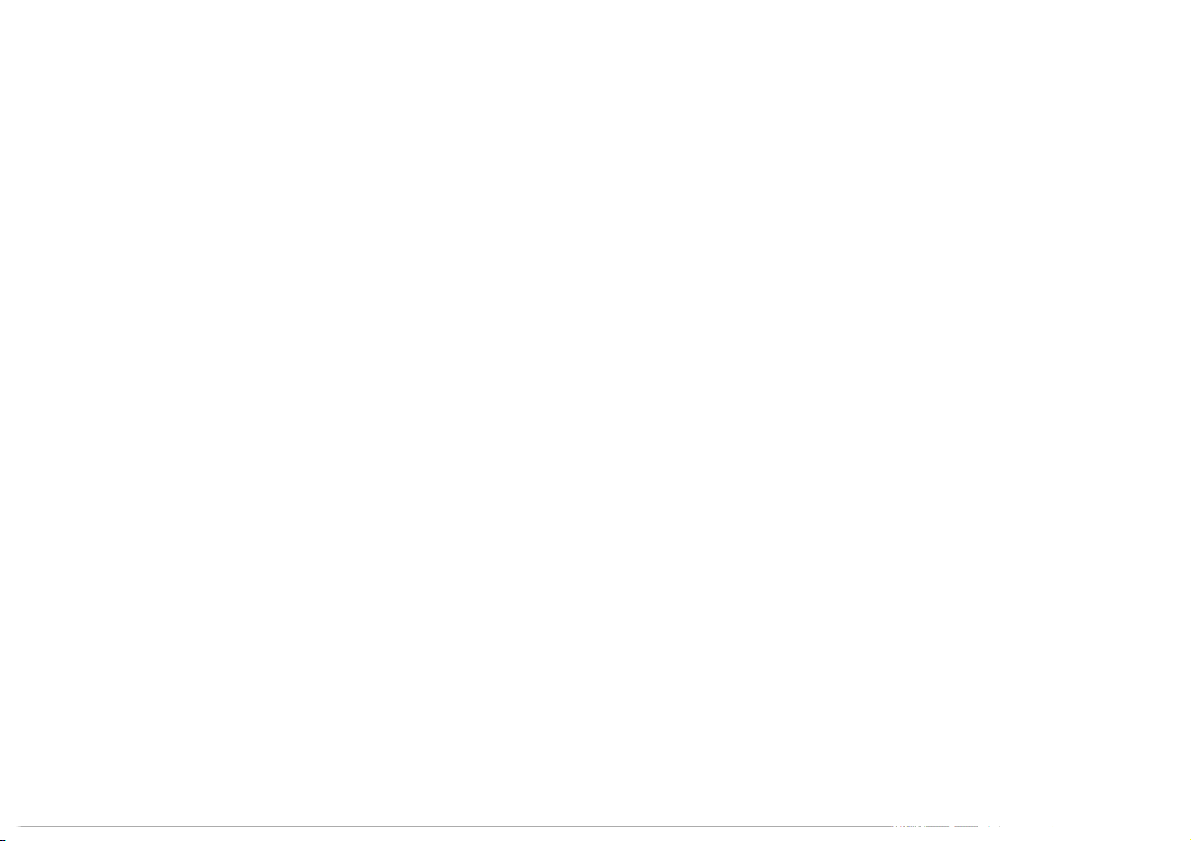
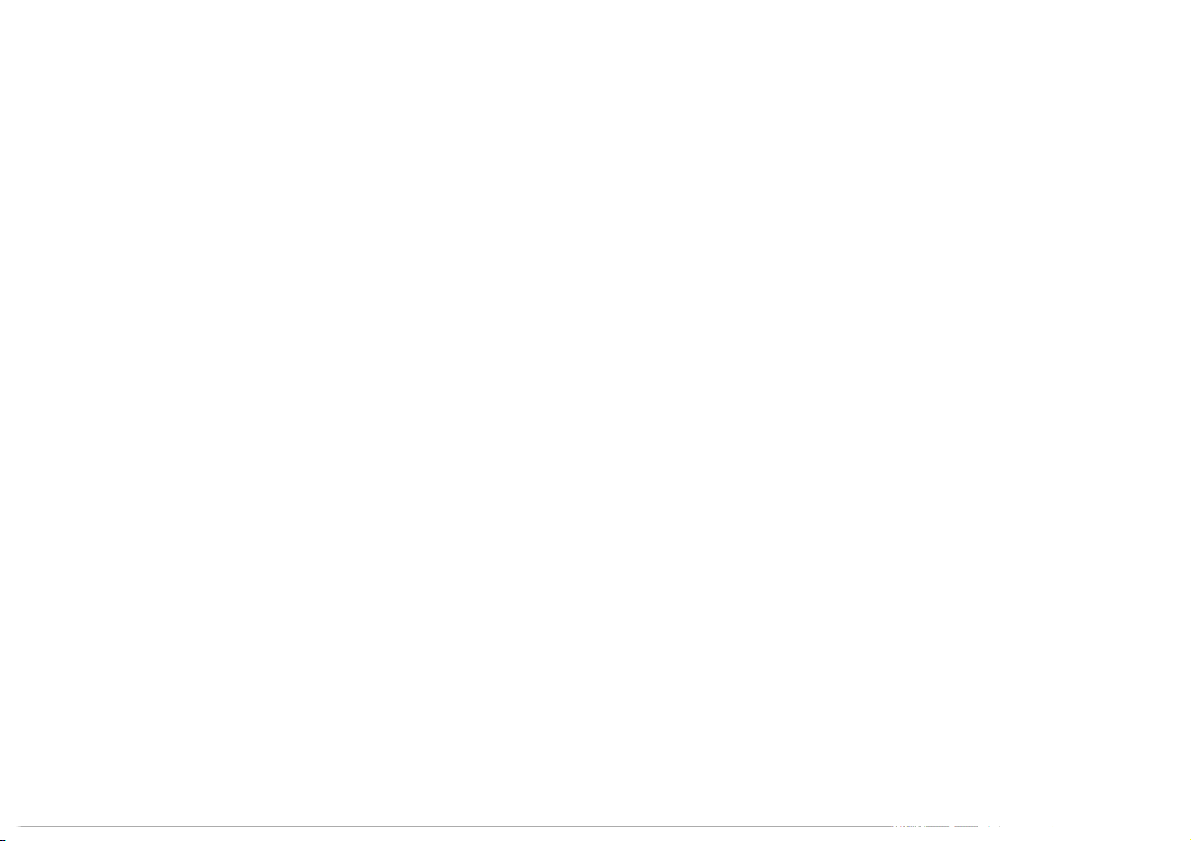
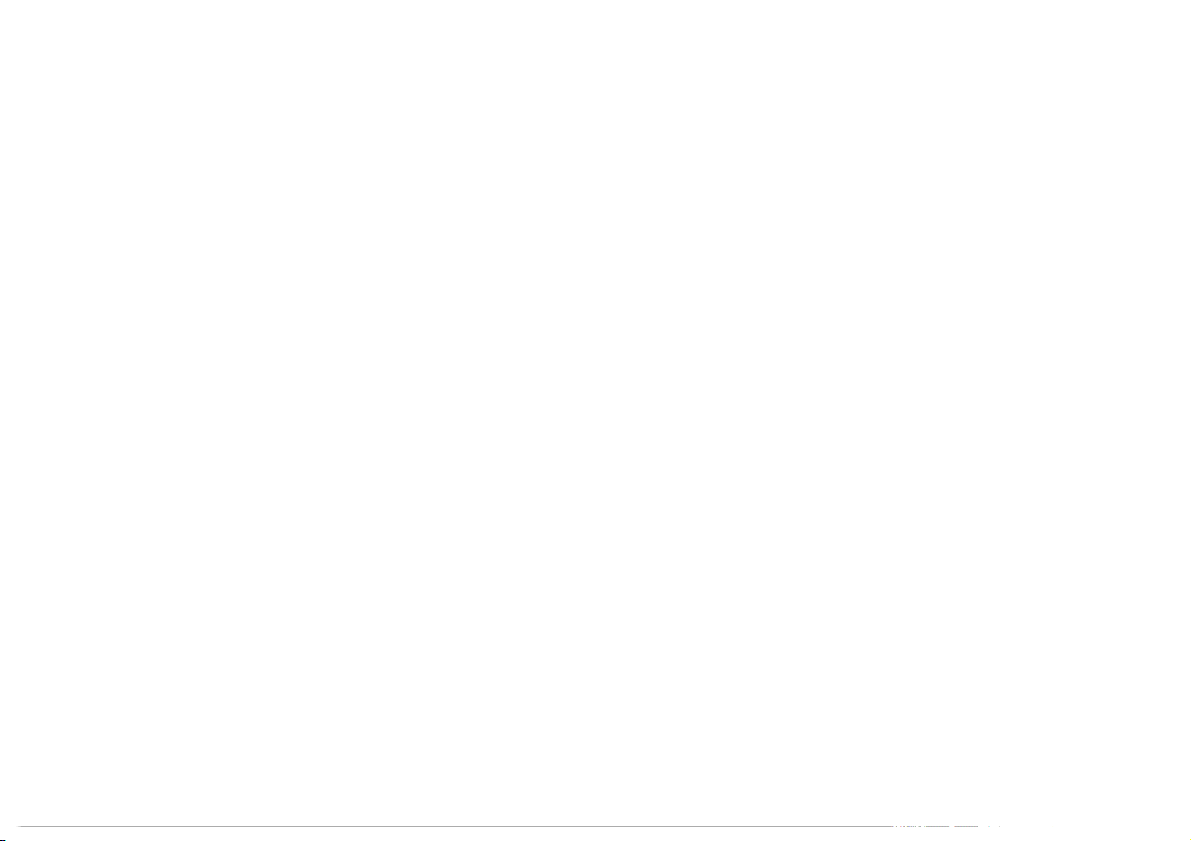
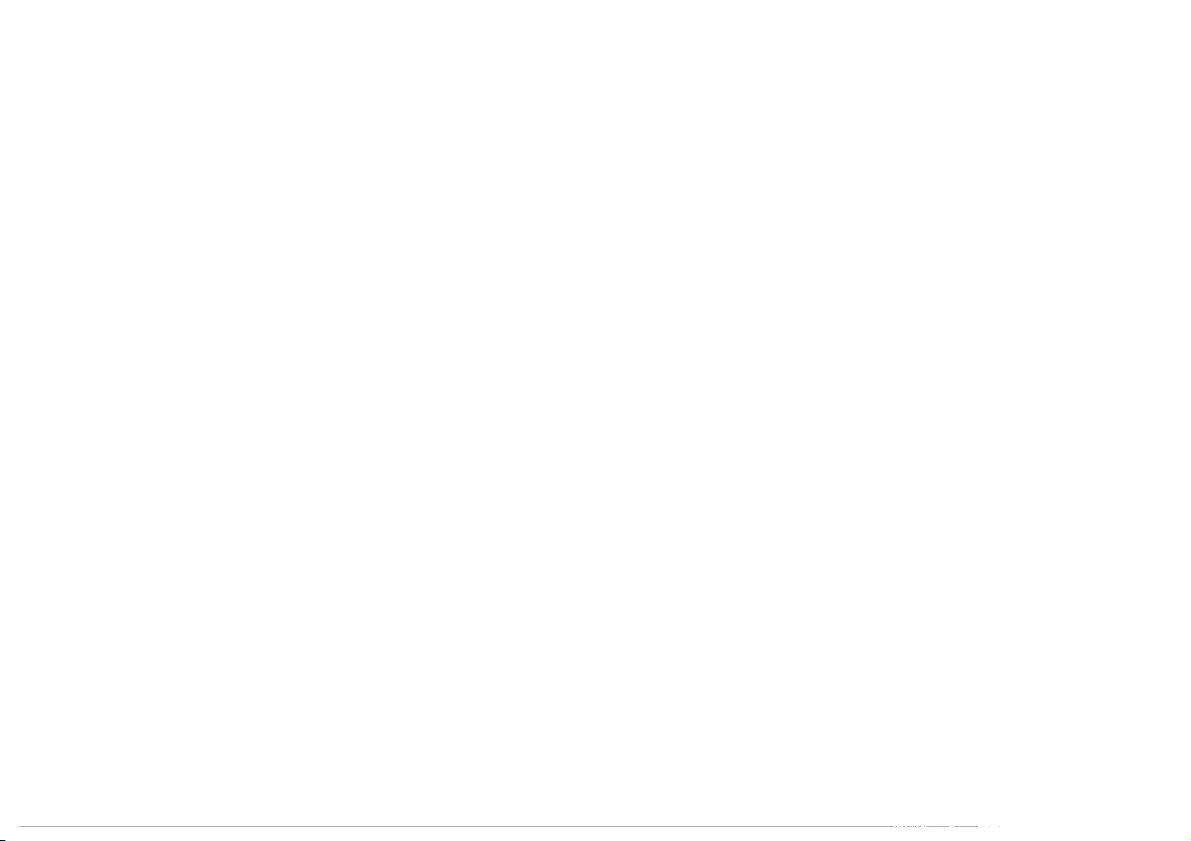
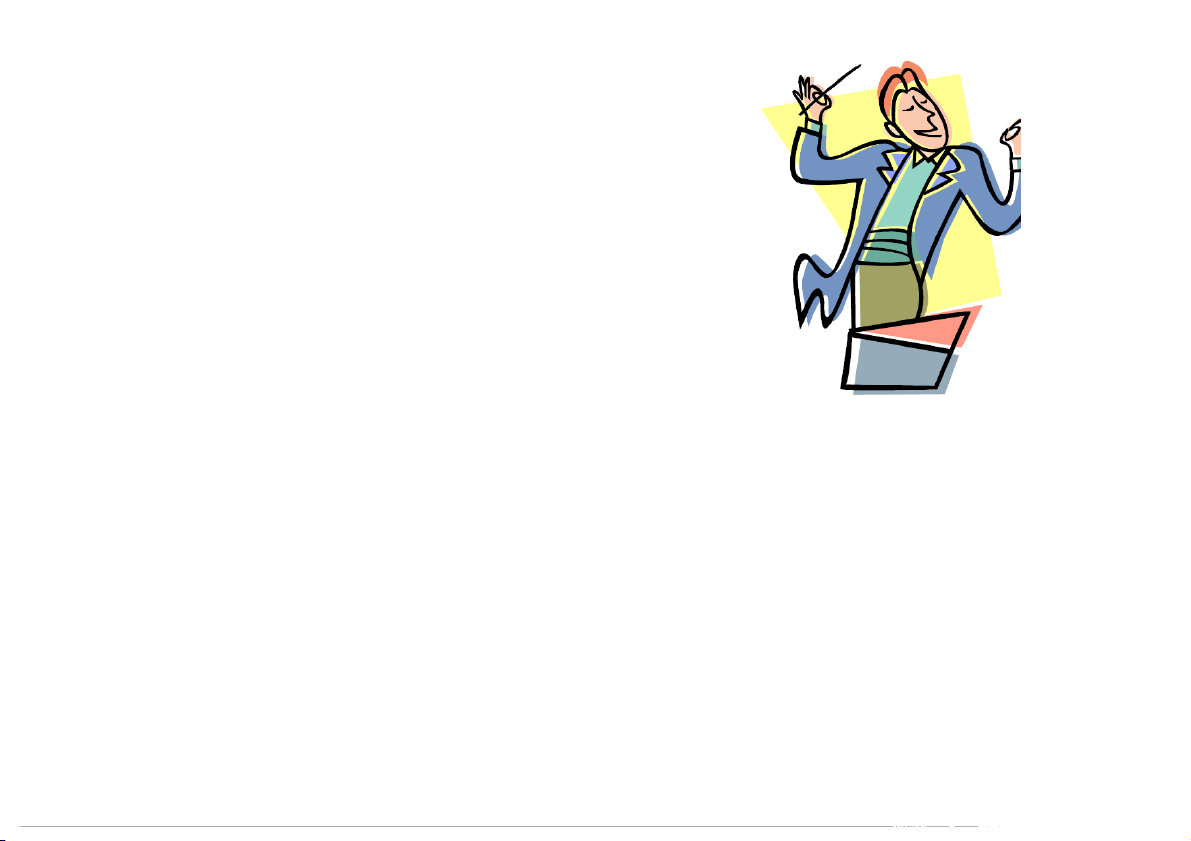
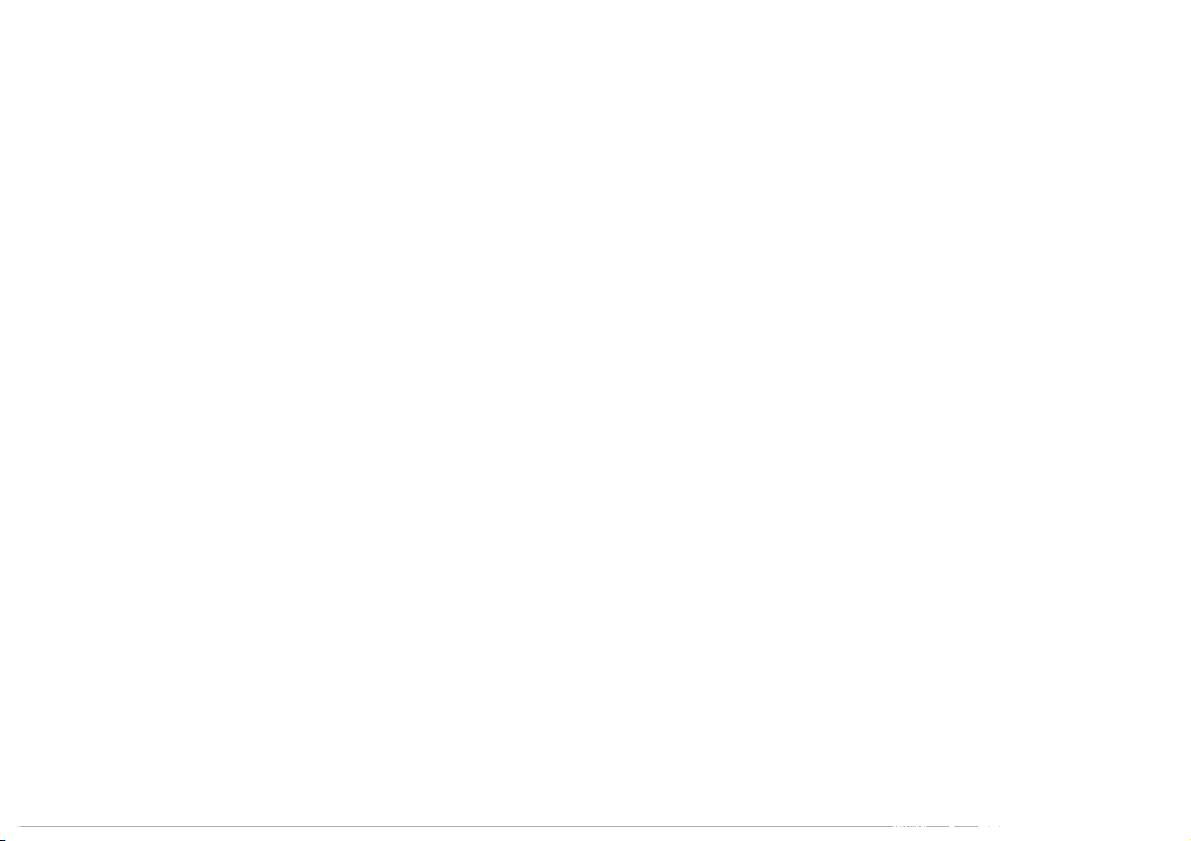
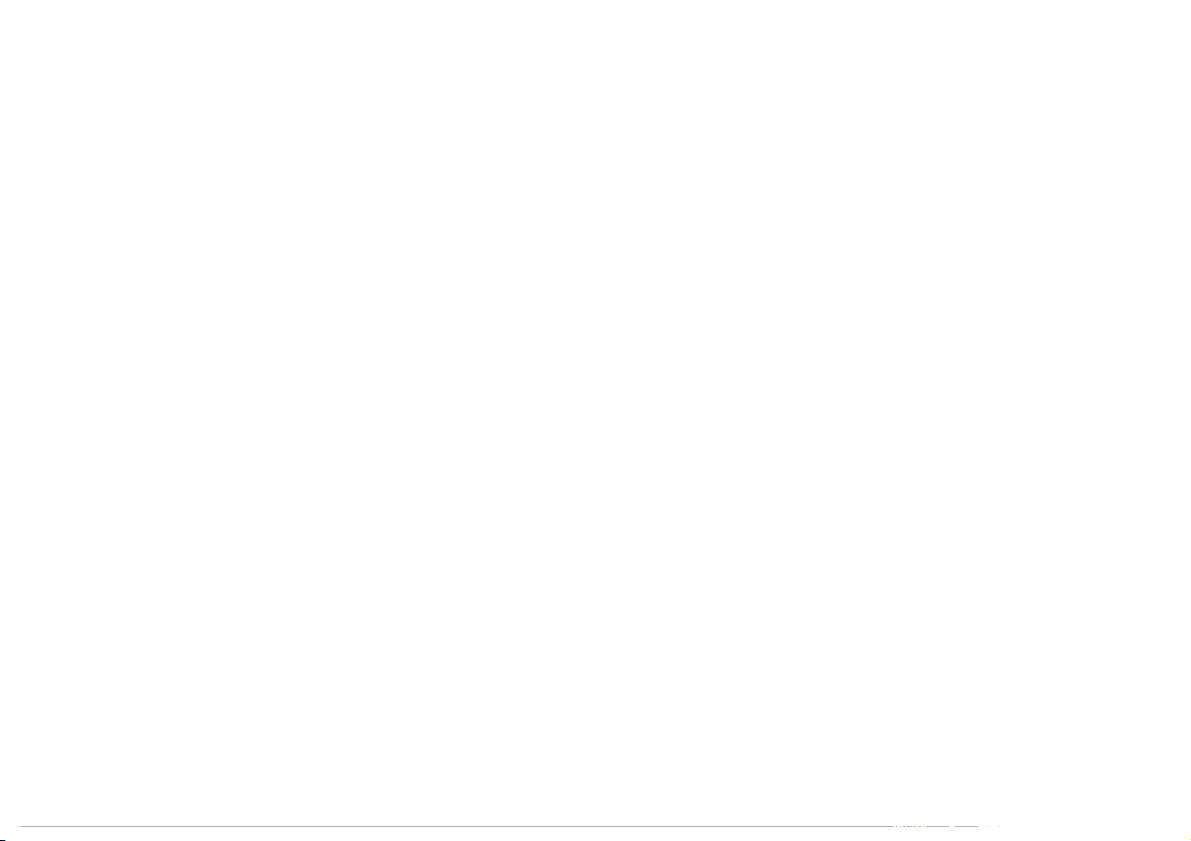
Preview text:
INT I ERNA E T RNA I T O I NAL UNIVE V RS E I RS T I Y T Y – –VNU V HCM NU C Ch C ap a t p e t r 11 IN I DU D CT C IV I E E RE R A E SONI S N ONI G N G Inductiv ucti e argu ar me m nt EX:
Many Vietnamese brides choose “Áo dài” for their traditional
dress in their engagement ceremony.
So, An might wear “Áo dài” in her engagement.
• Indicator words: likely, probably, it’s plausible to suppose
that, it’s reasonable to believe that, one would expect that,
it’s a good bet that, chances are that, and odds are that 6 Gr G oup discussion
Discuss with your friends to find examples for
each types of inductive arguments as follows: • Inductive generalization • Statistical arguments • Arguments from analogy • Causal arguments • Predictive argument • Argument from authority Inductiv ucti e argu ar me m nt
Another way to identify inductive arguments is
look for their COMMON PATTERNS: • Inductive generalization • Statistical arguments • Arguments from analogy • Causal arguments • Predictive argument • Argument from authority 8 Inductiv ucti e Generaliza i tio ti ns
An inductive generalization is an argument that
relies on characteristics of a sample population to
make a claim about the populations as a whole.
• In other words, it is an argument that uses evidence
about a limited number of people or things of a certain
type (the sample population) to make a claim about a
larger group of people or things of that type (population as a whole) 9 Inductiv ucti e Generaliza i tio ti ns I kn k o n w o f w ifv i e v e o r o si s x i x t r t u r ck c k dr d irv i e v rs, s ,a n a d n d a l a ll lo f o th t e h m e ar a e r e Democ mo r c a r t a s t . s .So S , o pr p o r b o ab a l b y l y mos mo t s tt r t uc u k c k dr d irv i e v rs r s ar a e r e De m De o m cr c a r t a s t .
This argument is an inductive generalization.
An inductive generalization is an argument that uses evidence
about a limited number of things of a certain type to make a
claim about all or most things of that type.
In the example above, a general conclusion about most truck
drivers is supported by personal experience of a relatively
small sample of only five or six truck drivers. 10 Inductiv ucti e Generaliza i tio ti ns
All the bass Hank has caught in the Susquehanna River have weighted less than one pound.
So, most of the bass in the Susquehanna River weigh less than one pound
The sample population is the bass Hank has caught in the Susquehanna River .
The population as a whole is all the bass in the Susquehanna River
All the bass Hank has caught in the Susquehanna River have weighted less than one pound.
So, many of the bass in the Susquehanna River weigh less than one pound
To lower the certainty of the conclusion make the argument stronger 11 Inductiv ucti e Generaliza i tio ti ns
In understanding inductive generalizations: • identify the sampl p e l e pop o u p l u a l t a iton o n and the po p p o u p l u a l t a iton o n as a a who h l o e l
(i.e. the population that the generalization is about) in an inductive generalization.
• A good inductive argument should reach a conclusion that is
appropriate to the evidence offered in the premises.
• A more moderate conclusion makes the inference stronger.
• An overstated conclusion makes the inference weaker. 12 Inductiv ucti e Generaliza i tio ti ns •
None of the medical doctors Jen has ever met smoked
cigarettes while examining her. •
So, no doctor smokes cigarettes while examining patients. 13 Evaluat l i uat ng i ind i ucti ct ve i generalizations
In evaluating inductive generalizations, there are three questions we should ask: Are the premises true? Is the sample large enough?
Is the sample representative or typical of the population as a whole?
An inductive generalization is a good one only if
we can answer "yes" to all three questions. 14 Evaluat l i uat ng i ind i ucti ct ve i generalizations • Are the premises true?
EX: Most CEOs of Fortune 500 companies are women
So, the CEOs of big businesses are probably women •
Is the sample population large enough?
EX: None of the thousands of rabbits Alan has come across has tried to attack him
So, most rabbits are not inclined to attack human beings. 15 Eva E luat l in i g ind g i u nd c u t c itve generalilzat i i zat o i n o s n • Is s the h e s a s m a p m l p e l po p pu p l u a l t a ion o n r e r p e r p es e e s n e t n a t t a itve e of ft h t e h e po p p o ul u a l t a ito i n o n as a a a w ho h le l ? e
EX: Two nuclear bombs were dropped on Japan, and
today Japan has one of the strongest economies in the
world. So, all the concern about nuclear warfare at the
end of humankind is a brunch of nonsense. • A re r p e re r s e e s n e t n a t t a itv i e v e sam a pl p e l
e is similar to the population as
a whole in all relevant respects. 16 Evaluat l i uat ng i ind i ucti ct ve i generalizations
I've dated three men from Texas, and all of them wore
cowboy boots. So, it's probably the case that all men from Texas wear cowboy boots.
Assuming that the premise of this argument is
true, is this a good inductive generalization or not? 17 Opi O nion ni pol on l pol s l s and in i ducti duct v i e v ar guments nt • Le L v e el e lof o fc e c r e ta t i a n i t n y t y and marg r i g n i n of fer e r r or; • Se S l e fl-s - e s l e e l c e t c itn i g n g sa s m a pl p e l s
e . Some polls do not even attempt
to utilize a truly random sample. Instead, they use self- selecting samples.
• the tendency of people to respond to polls di d s i h s on o e n s e t s lty l ,
and the tendency of agencies with vested interests to ask sl s a l n a t n ed e d qu q e u st s ito i n o s n , can bias a poll sample;
Ex: Slanted question (Loaded question) Where do you like to party?
Instead, could ask it like this: What do you like to do on weekend evenings? 20 • The merits of a do d u o bl b e-b - lin i d n d pol for generating objective results.
• Double blind: the person taking the poll and the
person responding should have no information
about each other, or at least at little as
possible. And neither the pollster nor the
respondent should have any indication of the “ right answer” 21 Sta S tistica ti l stica A l r A g r uments
A statistical argument argues from premises regarding
a percentage of population to a conclusion about an
individual member of that population or some part of that population. 22 Exa E mp m le l •
Only 3 percent of IU are against building the new gymnasium • A is a IU student •
So, A is not against building the new gymnasium Strong argument
Only 3 percent of IU are against building the new gymnasium A is a IU student
So, A is probably not against building the new gymnasium 23 Sta S tistica ti l stica A l r A g r uments
• Like other inductive arguments, statistical arguments
are evaluated along a continuum of strong to weak.
• Statistical arguments that approach 50 percent may be
strong but are to be considered relative unreliable. • The more specific the re r fe f r e e r n e c n e c e c l c a l s a s s s i s, the better the argument is 24 Refere r nce class •
90% of IU students are in favor of not having a cumulative final
exam in their critical thinking class. • Andy is a IU student. •
So, Andy is in favor of not having a cumulative final exam in her critical thinking class.
85% of IU students who like writing want the cumulative final because it will have an essay
Andy is a IU student who like writing essays
So, Andy is probably wants the cumulative final. 25 Induction ucti a on nd analo al gy • What is an analogy?
• Up is to down and right is to?
• An analogy is a comparison of things based similarities those thing share.
• A mother ‘s love is like the sun. 26 How can we argue by analog? l
• Whereas analogies simply point out a similarity,
arguments from analogy claim that certain similarities
are evidence that there is another similarity (or other similarities).
• EX: The Post Office is a government agency
The Department of Motor Vehicles is a government agency
The Post Office is closed for Martin Luther King Jr. Day
The Department of Motor Vehicles must be closed for Martin Luther King Jr. Day 28 How can we argue by analog? l • A has characteristic X • B has characteristic X • A has characteristic Y
• Therefore, B has characteristic Y
• A has characteristics X and Y
• B has characteristics X and Y • A has characteristic Z
• Therefore, B has characteristic Z. 29 Eva E luat l in i g argume gum nts from r analo anal gy
• Good argument: the compared items in an analogical argument share a sufficient nu n mbe mb r of r f e r l e e l v e a v nt n tsi s milarities
to warrant accepting the conclusion;
• Bad argument: the compared items in an analogical argument share a sufficient nu n mbe mb r of r f e r l e e l v e a v nt n t di d s i si s milarit i i milarit e i s e
s to warrant rejecting the conclusion;
• To Strengthen the argument: in i c n re r a e s a e s e sa s mpl mp e l s e i s z i e z , di d v i er e s r i s tiy t , y s , p s e p ci c fiic i ity t o y f o t f h t e h co c nc n l c u l s u i s o i n o r n e r l e a l titv i e v e to t th t e e pr p e r mise mis s e . 31 Eva E luat l in i g argume gum nts from r analo anal gy •
A and B are both tall and play basketball • A is also play vol eyball •
So, B must also play volleyball
True premise? Relevance? Number of relevance similarities? MAKE THE ARGUMENT STRONGER 1. Increase sample size
A, B, C and D are all tall and play basketball
A, C and D are also play volleyball
So, B must also play volleyball
2. Increase specificity of the conclusion relatice to the premise
“B must also play volleyball”: narrow and specific.
B must have played a game of volleyball at some time B may also play volleyball 32 Causal A al r A g r u g m u ents
• A cause is that brings about a change, that which produces an effect.
• Causal terms: such as, produce, is responsible for,
affects, makes, changes and contributes to 33 Eva E luat l in i g causal argu ar m gu ents
SINGLE INSTANCE: X cause higher rate of Y in some individuals.
A’s car wouldn’t start this morning, and she hasn’t replaced the
battery since she bought six months ago.
So, it is probably a dead battery that caused the car not to start.
GENERAL RELATIONSHIP: X causes a higher rate of Y in the population.
The Surgeon General has found that there is a strong link between
smoking cigarettes and getting lung cancer
So, smoking cigarettes causes lung cancer 35 Eva E luat l in i g causal argu ar m gu ents •
The distorting effect of selective attention and memory ( focusing
attention on, or recal ing from memory, only certain example distort
example) to evidence supporting a causal conclusion; •
EX: Every time we have a full moon, people behave strangely. So, the
full moon must cause the stranger behavior. •
EX: When the national Vietnam football team plays at the Mỹ Đình
stadium, the team always has a good score. So, the Mỹ Đình stadium
must cause the VN players performing better that other team players. •
The unreliability of anecdotal evidence: what others tell us 36
• The merit of a control ed experiment in discerning causal relationships EX: • To T se s e e e i fi fc h c em e i m c i a c l a lX X p r p e r ve v n e t n s s d i d s i e s a e s a e s e Y : Y
• A scientist gives chemical X to group one: the experimental group; and
does not give X to group two : the control group.
• A human believes that the drug is given will prevent or curve her disease
will have a better chance of prevention or cure: placebo effect. • do d ub u l b e l - e bl b iln i d n d s t s u t d
u y: neither the subjects nor the experimenters know who
receive the treatment and who is receiving the placebo until the experiment is finish 37 Correl r at el io i n and cause
• Sometimes, two things or events are clearly associated or linked: where
you find X, you will often find Y. A relationship such of this, in which two
things are frequently found together, is called a correlation.
• Sometimes a correlation is an indicator of a cause and effect relationship.
• A positive correlation, one that indicates two things are found together
more than 50 percent of the time, may indicate a causal connection
between one thing and the other.
• A negative correlation, one that indicates two things are found together less
than 50 percent of the time, may indicate that one thing prevents the other. •
The important question is this: Is the correlation significant? The answer
depends, in part, on the size of the sample 38 Qui Q zzes f zzes or f chapter 11 er • On separate sheet 39 A c A lose l r ose l r o l o o k at a Priori or i Pr P obabil bi ilty i
• Epistemic probability is the kind of probability we assign to things
we have good reason to believe but to which we cannot assign a
probability on any truly mathematical basic.
• Relative frequency probability is the kind of probability that takes
information about a group as a whole and applies it to an
individual case, based on accumulated data derived from what
has already been observed in the group. 40 A f A ew words about pro ds about pr b o ab
• Probability – Inductive reasoning:
• There is an important connection between probability and induction.
• All induction arguments are a matter of probability because they
are not certain. They do not guarantee the truth of their
conclusion, but only offer evidence that the conclusion is probably true. 41 A c A loser l oser l ook l ook at at a Priori Pri ori Pro babil abi iltiy t
• Statements of a priori probability have odds that can be calculated
prior to, and independent of, sensory observation.
• The gambler’s fallacy is the mistaken belief that a past event has an
impact on a current random event.
• According to the law of large numbers, the proximity of theoretically
predicted and actual percentages tends to increase as the sample
grows. In other words, the bigger the sample, the closer the actual
results are likely to be to the predicted results.
• Expected value is essentially the payoff or loss you can expect from making a bet. 42 A c A loser l oser l ook l ook at at a Priori Pri ori Pro babil abi iltiy t
• Relative value is the value a bet has in relation to an individual’s
own needs, preferences, and resources.
• Diminishing marginal value is the principle that as quantity
increases, relative value tends to decrease. 43