Bài tập tiểu luận nhóm học phần Econometrics with Financial Application | Trường Đại học Quốc tế, Đại học Quốc gia Thành phố Hồ Chí Minh
You obtain the following sample autocorrelations and partial autocorrelations for a sample of 100 observations from actual data? Can you identify the most appropriate time series process for this data? Using the Ljung-Box Q* test to determine whether the first three autocorrelation coefficients taken together are jointly significantly different from zero. Tài liệu giúp bạn tham khảo, ôn tập và đạt kết quả cao. Mời bạn đón xem.
Môn: Econometrics with Financial Application (BA174IU)
Trường: Trường Đại học Quốc tế, Đại học Quốc gia Thành phố Hồ Chí Minh
Thông tin:
Tác giả:
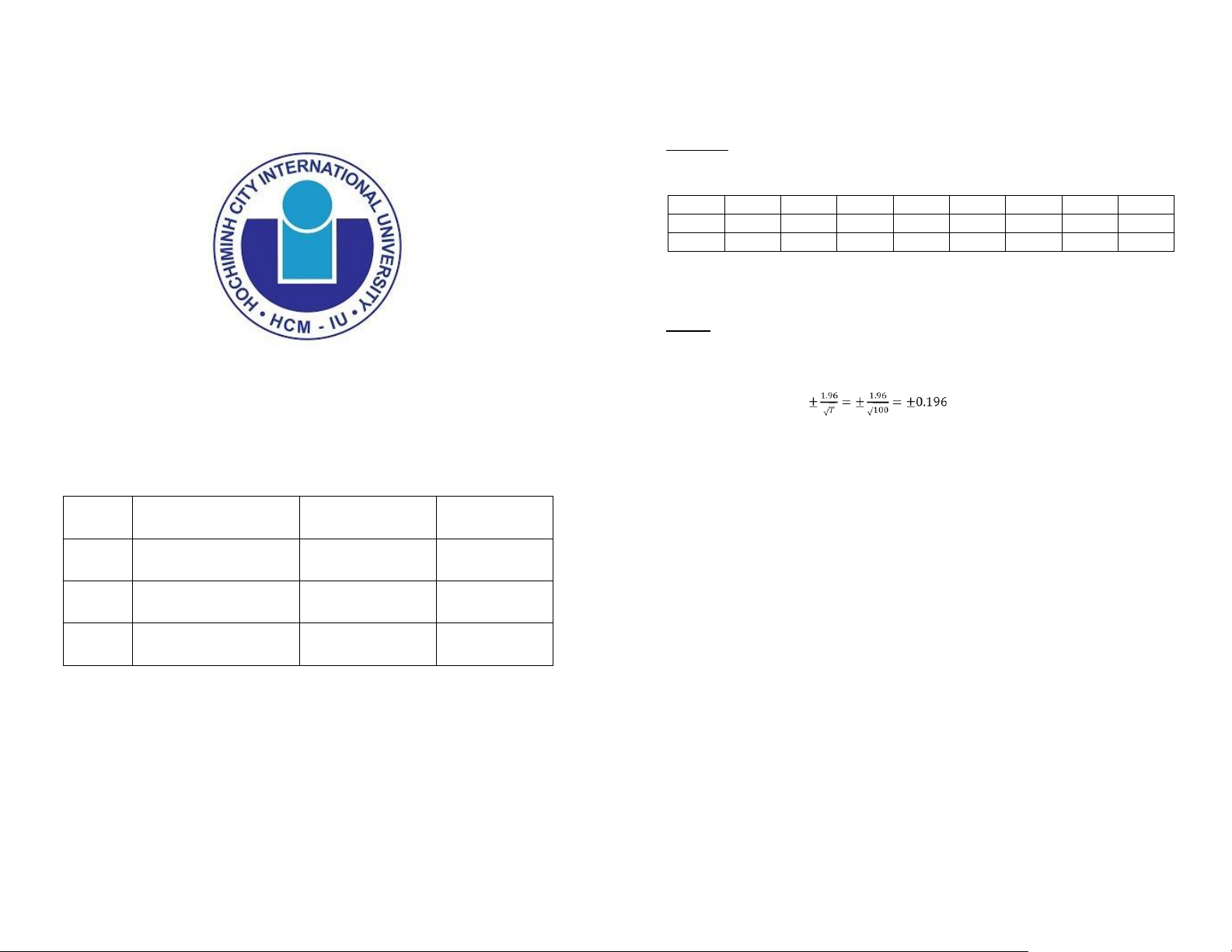
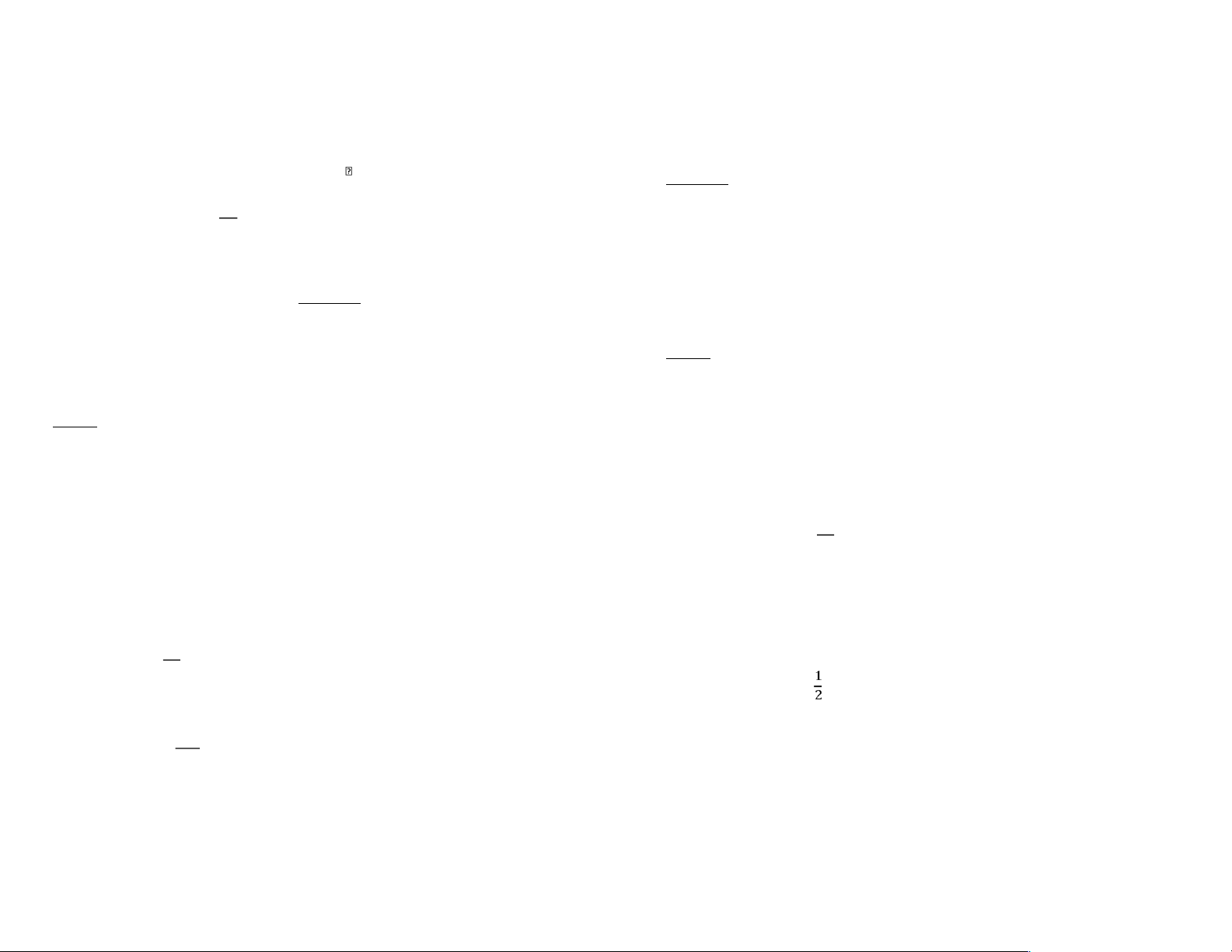
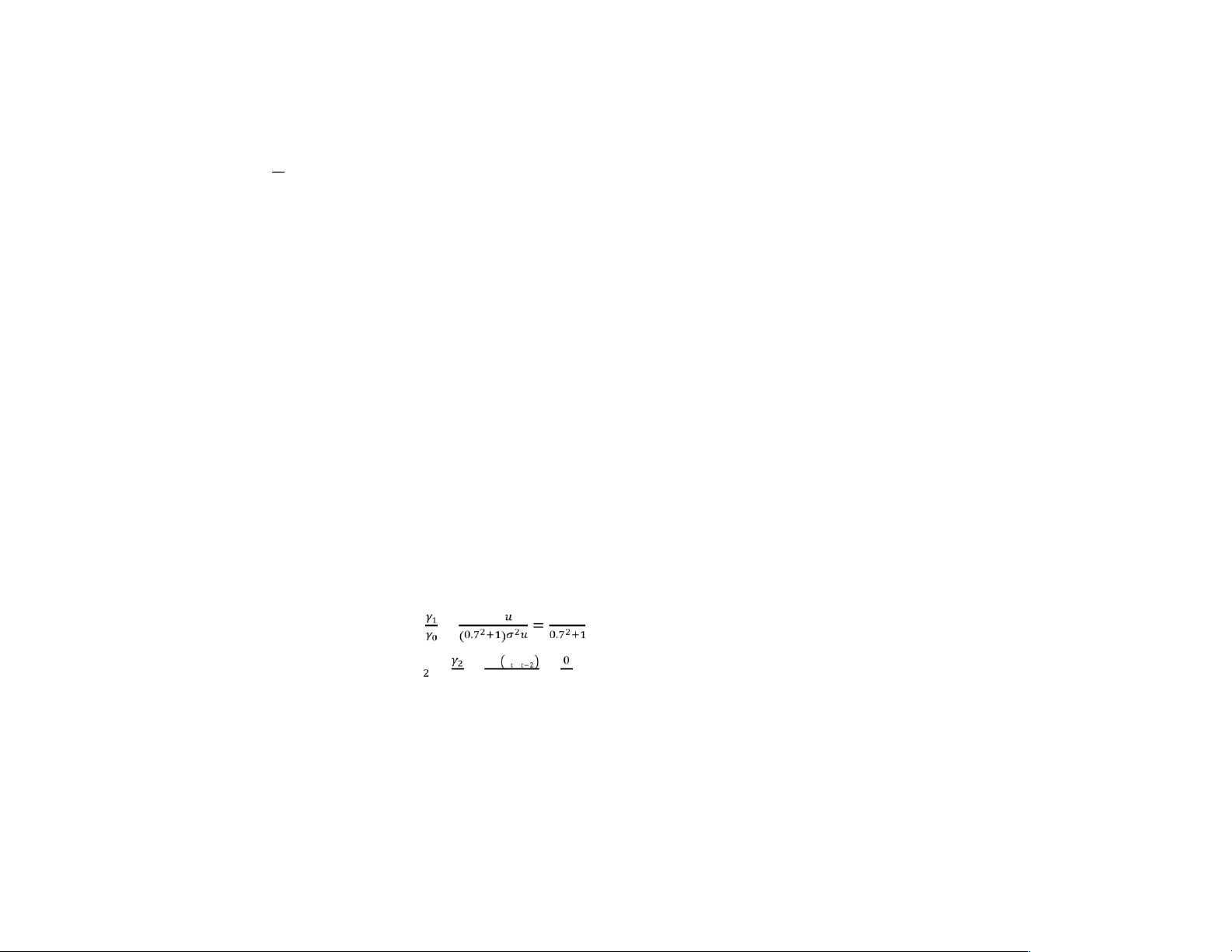
Preview text:
International University – HCMC
Econometrics with Financial Application
Vietnam National University – HCMC
International University – HCMC
Econometrics with Financial Application International University HOMEWORK CHAPTER 6 SCHOOL OF BUSINESS Question 1:
You obtain the following sample autocorrelations and partial autocorrelations for a sample of
100 observations from actual data: Lag 1 2 3 4 5 6 7 8 ACF 0.420 0.104 0.032 -0.206 -0.138 0.042 -0.018 0.074 PACF 0.632 0.381 0.268 0.199 0.205 0.101 0.096 0.082
Can you identify the most appropriate time series process for this data?
Using the Ljung-Box Q* test to determine whether the first three autocorrelation coefficients
taken together are jointly significantly different from zero. Answer: Single test: 𝐻 :𝜏 = 0
Econometrics with Financial Application_S2_2022-23_G01 Dr Using confidential interval Nguyen Phuong Anh
0.42 > 0.196 => Reject 𝐻 :𝜏 = 0
-0.196 < 0.104 < 0.196 => Not reject 𝐻 :𝜏 = 0
-0.196 < 0.032 < 0.196 => Not reject 𝐻 :𝜏 = 0
-0.206 < 0.196 => Reject 𝐻 : 𝜏 = 0 Seq. Full name Student ID Contribution
-0.196 < -0.138 < 0.196 => Not reject 𝐻 : 𝜏 = 0 1 Trương Phúc An BABAIU20526 100%
-0.196 < 0.042 < 0.196 => Not reject 𝐻 :𝜏 = 0
-0.196 < -0.018 < 0.196 => Not reject 𝐻 : 𝜏 = 0 2 Nguyễn Hoàng Bảo Hân BAFNIU19077 100%
-0.196 < 0.074 < 0.196 => Not reject 𝐻 :𝜏 = 0
Since only 𝜏 ≠ 0 𝑎𝑛𝑑 𝜏
≠ 0 => 𝐴𝐶𝐹 = 0 𝑎𝑓𝑡𝑒𝑟 4 𝑙𝑎𝑔𝑠 3 Hồ Thế Phong BAFNIU19141 100% |𝜏 | = 0.643 |𝜏 | = 0.381 |𝜏 | = 0.268 Page 1 of 5
This shows that PACF is slowly decaying to 0
International University – HCMC
Econometrics with Financial Application Page 2 of 5 0.68
→ 𝐴𝑅𝑀𝐴 𝑖𝑠 𝑖𝑛𝑣𝑒𝑟𝑡𝑖𝑏𝑙𝑒
• MA is more suitable. Since ACF = 0 after 4 lags, we use MA (4). Page 3 of 5 Ljung-box formula: 𝐻 : 𝜏 = 𝜏 = 𝜏 = 0 (𝑚 = 3)
International University – HCMC
Econometrics with Financial Application
• Test statistic Q* compared with CV from 2 (3) • Question 3: CV=7.815 •
Considering the following 3 models that a researcher suggests might be a reasonable model of
𝑄∗ = 𝑇 × (𝑇 + 2) ∑ stock market prices:
• 𝑄∗ = 19.4 > 𝐶𝑉 => 𝑄∗ 𝑏𝑒𝑙𝑜𝑛𝑔𝑠 𝑡𝑜 𝑡ℎ𝑒 𝑟𝑒𝑗𝑒𝑐𝑡𝑖𝑜𝑛 𝑟𝑒𝑔𝑖𝑜𝑛 i) 𝑦 = 0.7𝑢 + 𝑢
• 𝑅𝑒𝑗𝑒𝑐𝑡 𝐻 : 𝜏 = 𝜏 = 𝜏 = 0 j) 𝑦 = 0.4𝑦 + 𝑢
• The first three autocorrelation coefficients taken together are jointly k) 𝑦 = 2𝑦 + 𝑢
significantly different from zero Question 2:
a) What classes of model are these examples of?
b) Are these models stationary?
Considering the following ARMA process:
c) Calculate the autocorrelation coefficients for the process (i) and (j) up to lag 2. 𝑦 = 2.1 + 1.5𝑦 + 0.68𝑢 + 𝑢 Answer:
Determine whether the MA part of the process is invertible.
a) i is MA. While j and k is AR.
Determine whether the AR part of the process is stationary.
b) Since MA is always stationary => i is stationary 𝑗) 𝑦 = 0.4𝑦 + 𝑢 Answer:
↔ 𝑦 = 0.4𝐿𝑦 + 𝑢 (1 −
ARMA (1, 1) model is stationary when AR part is stationary 0.4𝐿)𝑦 = 𝑢 𝑦 = 2.1 + 1.5𝑦 (𝐴𝑅(1))
𝛷(𝑍) = 0 ↔ 1 − 0.4𝑍 = 0 𝑦 − 1.5𝑦 = 2.1 + 0.68𝑢 + 𝑢 1
𝑦 − 1.5𝐿𝑦 = 2.1 + 0.68𝐿𝑢 + 𝑢 → 𝑍 =
> 1 → 𝑜𝑢𝑡𝑠𝑖𝑑𝑒 𝑢𝑛𝑖𝑡 𝑐𝑖𝑟𝑐𝑙𝑒 → 𝑆𝑡𝑎𝑡𝑖𝑜𝑛𝑎𝑟𝑦
(1 − 1.5𝐿)𝑦 = 2.1 + (0.68𝐿 + 1)𝑢 0.4 𝑘) 𝑦 = 2𝑦 + 𝑢
(1 − 1.5𝐿)𝑦 = 𝛷(𝐿), (0.68𝐿 + 1)𝑢 = 𝜃(𝐿)
↔ 𝑦 = 2𝐿𝑦 + 𝑢 (1 −
𝛷(𝑍) = 0 ↔ 1 − 1.5𝑍 = 0 2𝐿)𝑦 = 𝑢 1 → 𝑍 =
< 1 → 𝑖𝑛𝑠𝑖𝑑𝑒 𝑢𝑛𝑖𝑡 𝑐𝑖𝑟𝑐𝑙𝑒 → 𝐴𝑅 𝑝𝑎𝑟𝑡 𝑖𝑠 𝑛𝑜𝑡 𝑠𝑡𝑎𝑡𝑖𝑜𝑛𝑎𝑟𝑦
𝛷(𝑍) = 0 ↔ 1 − 2𝑍 = 0 1.5
𝜃(𝐿) = 0 ↔ 0.68𝑍 + 1 = 0
→ 𝑍 = < 1 → 𝑖𝑛𝑠𝑖𝑑𝑒 𝑢𝑛𝑖𝑡 𝑐𝑖𝑟𝑐𝑙𝑒 → 𝑁𝑜𝑡 𝑠𝑡𝑎𝑡𝑖𝑜𝑛𝑎𝑟𝑦 1 → |𝑍| =
> 1 → 𝑜𝑢𝑡𝑠𝑖𝑑𝑒 𝑢𝑛𝑖𝑡 𝑐𝑖𝑟𝑐𝑙𝑒 → 𝑀𝐴 𝑝𝑎𝑟𝑡 𝑖𝑠 𝑖𝑛𝑣𝑒𝑟𝑡𝑖𝑏𝑙𝑒
International University – HCMC
Econometrics with Financial Application
c) 𝐴𝑅(1): 𝑦 = 0.4𝑦 + 𝑢 + 0 × 𝑦 Yule-Walker system 𝜏 = 𝛷 + 𝜏 × 0 = 0.4 i) 𝑦 = 0.7𝑢 + 𝑢 𝑀𝐴(1) 𝛾
𝜏 = , 𝛾 = 𝑉𝑎𝑟(𝑦 ) 𝛾
(𝑖) → 𝐸(𝑦 ) = 𝐸(0.7𝑢 + 𝑢 ) Page 4 of 5 Page 5 of 5
= 0.7𝐸(𝑢 ) + 𝐸(𝑢 )
(𝑖) → 𝑉𝑎𝑟(𝑦 ) = 𝐶𝑜𝑣(𝑦 , 𝑦 )
= 𝐸[(𝑦 − 0)(𝑦 − 0)] = 𝐸[(0.7𝑢 + 𝑢 )(0.7𝑢 + 𝑢 )] = 𝐸[(0.7 𝑢 + 𝑢 + 1.4𝑢 𝑢 )] = (0.7 + 1)𝑉𝑎𝑟𝑢 = (0.7 + 1)𝜎 𝑢
(𝑖) → 𝛾 = 𝐶𝑜𝑣(𝑦 , 𝑦 ) = 𝐸[(𝑦 − 0)(𝑦 − 0)] = 𝐸[(0.7𝑢 + 𝑢 )(0.7𝑢 + 𝑢 )] = 𝐸[(0.7 𝑢 𝑢 + 𝑢 𝑢 + 1.4𝑢 )] = 1.4𝜎 𝑢 . . Then 𝜏 = =
Similarly, 𝜏 = = 𝐶𝑜𝑣 𝑦 ,𝑦 = =0 𝑉𝑎𝑟 𝑦𝑡
For MA (1), ACF = 0 after q lags.