-
Thông tin
-
Quiz
Key Economic Determinants of Foreign Direct Investment (FDI) Inflows in G7 Countries | Bài báo cáo học phần International financial management | Trường Đại học Quốc tế, Đại học Quốc gia Thành phố Hồ Chí Minh
G7 is the group containing seven countries regarded as developed countries in the world, namely the United States, United Kingdom, Germany, Canada, Japan, Italy, and France. These countries are considered advanced economic nations belonging to one of the largest organizations in the world named IMF. Until 2020, the aggregate group constitutes more than half of the global total wealth, which was at $418 trillion (Global Wealth Databook 2021, Credit Suisse). These countries constitute 32 to 46 percent of global GDP, the value is approximately 10 percent of the global population. The Group of Seven is also a powerful group that holds major world powers in terms of politics, economy, military, legal, etc. Due to their influencing power, these countries are regarded as advanced and developed countries that have an influence on every world activity in general, and economic activity in specific. Tài liệu giúp bạn tham khảo, ôn tập và đạt kết quả cao. Mời bạn đón xem.
International financial management 1 tài liệu
Trường Đại học Quốc tế, Đại học Quốc gia Thành phố Hồ Chí Minh 695 tài liệu
Key Economic Determinants of Foreign Direct Investment (FDI) Inflows in G7 Countries | Bài báo cáo học phần International financial management | Trường Đại học Quốc tế, Đại học Quốc gia Thành phố Hồ Chí Minh
G7 is the group containing seven countries regarded as developed countries in the world, namely the United States, United Kingdom, Germany, Canada, Japan, Italy, and France. These countries are considered advanced economic nations belonging to one of the largest organizations in the world named IMF. Until 2020, the aggregate group constitutes more than half of the global total wealth, which was at $418 trillion (Global Wealth Databook 2021, Credit Suisse). These countries constitute 32 to 46 percent of global GDP, the value is approximately 10 percent of the global population. The Group of Seven is also a powerful group that holds major world powers in terms of politics, economy, military, legal, etc. Due to their influencing power, these countries are regarded as advanced and developed countries that have an influence on every world activity in general, and economic activity in specific. Tài liệu giúp bạn tham khảo, ôn tập và đạt kết quả cao. Mời bạn đón xem.
Môn: International financial management 1 tài liệu
Trường: Trường Đại học Quốc tế, Đại học Quốc gia Thành phố Hồ Chí Minh 695 tài liệu
Thông tin:
Tác giả:
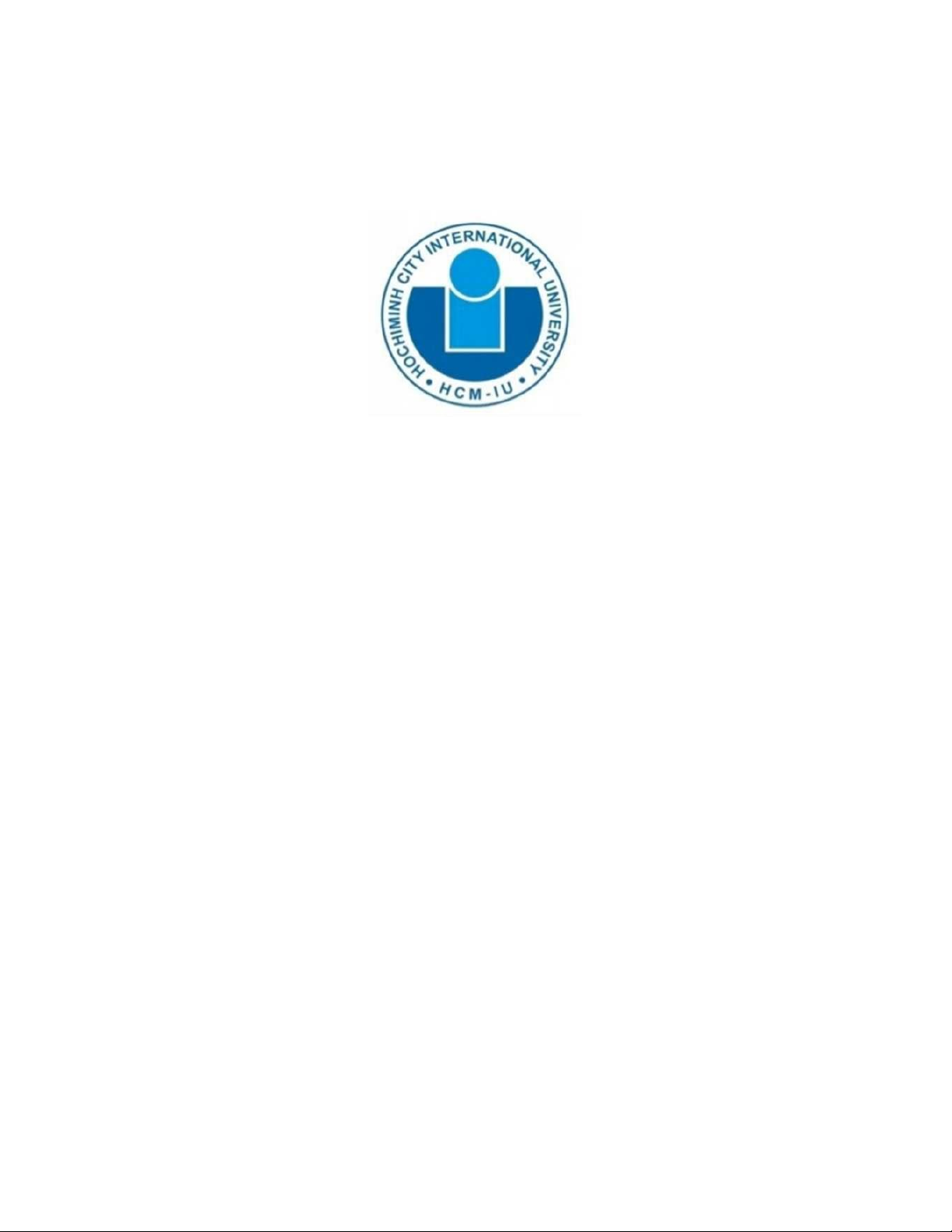
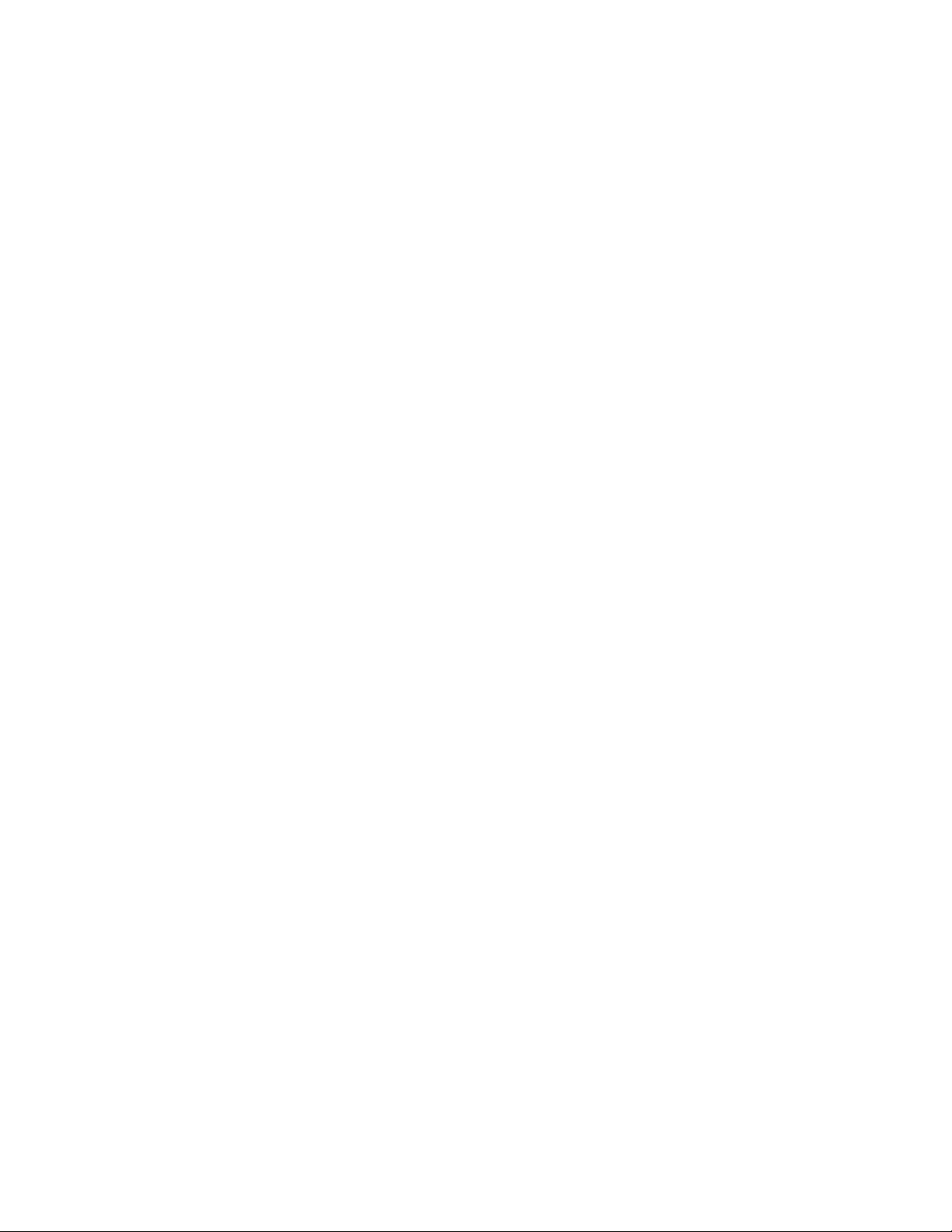
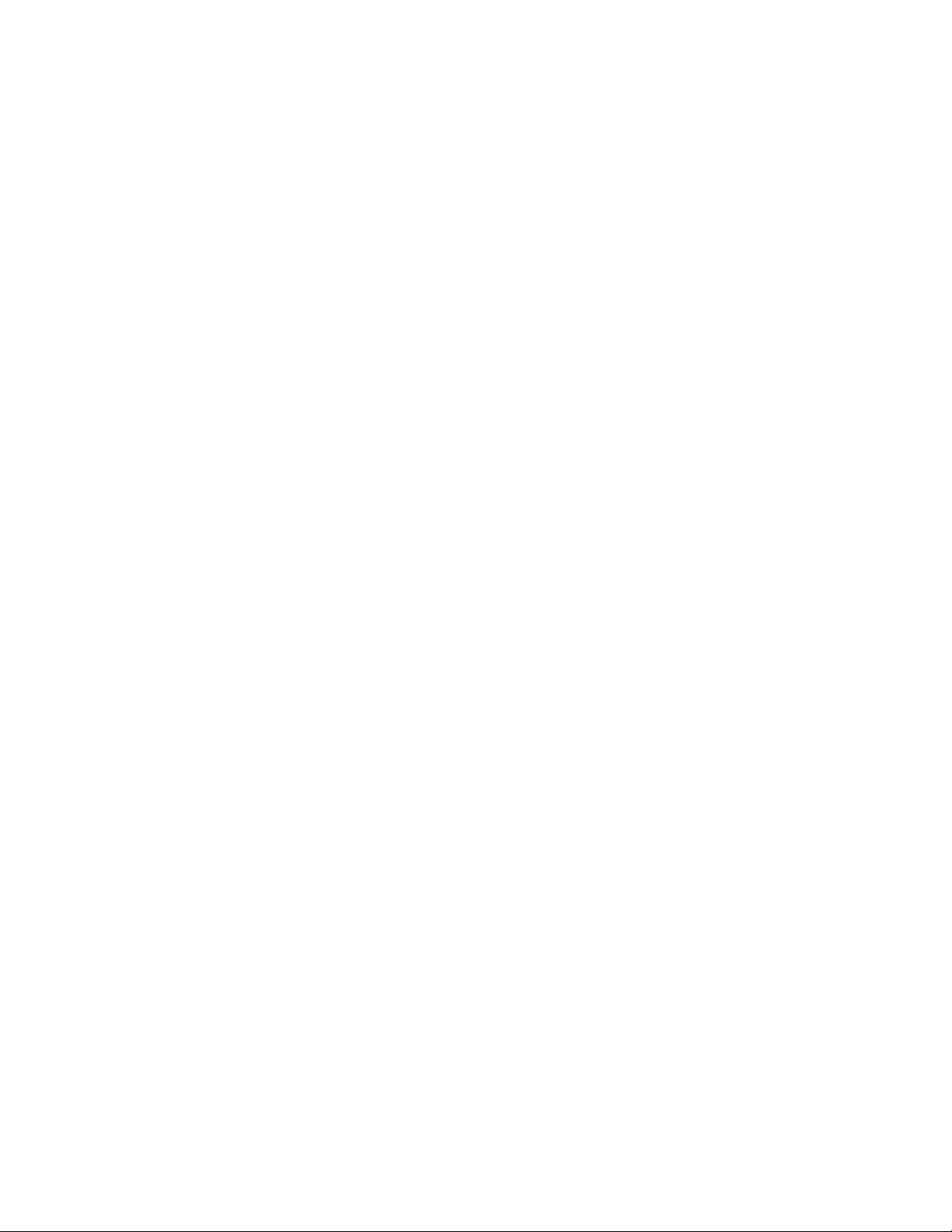
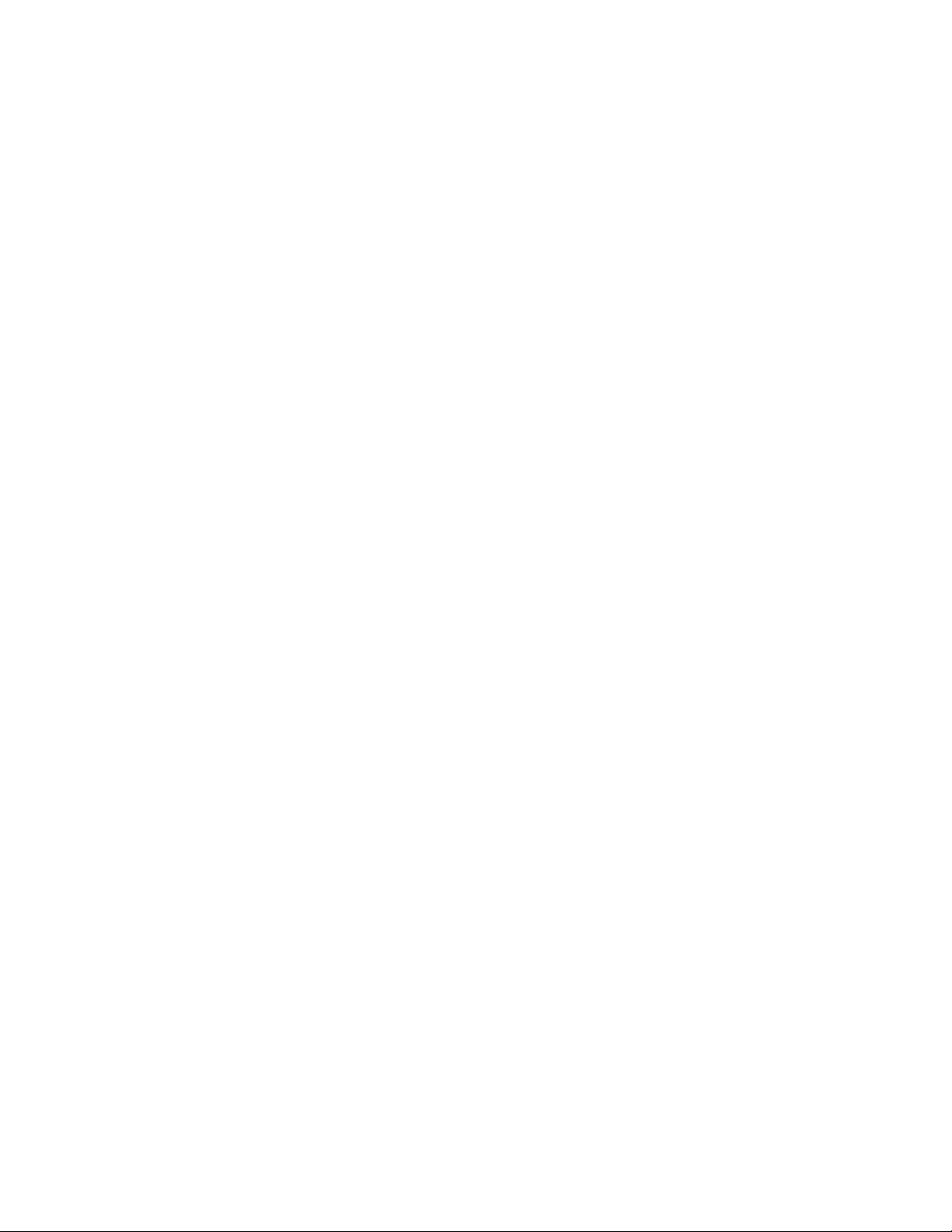
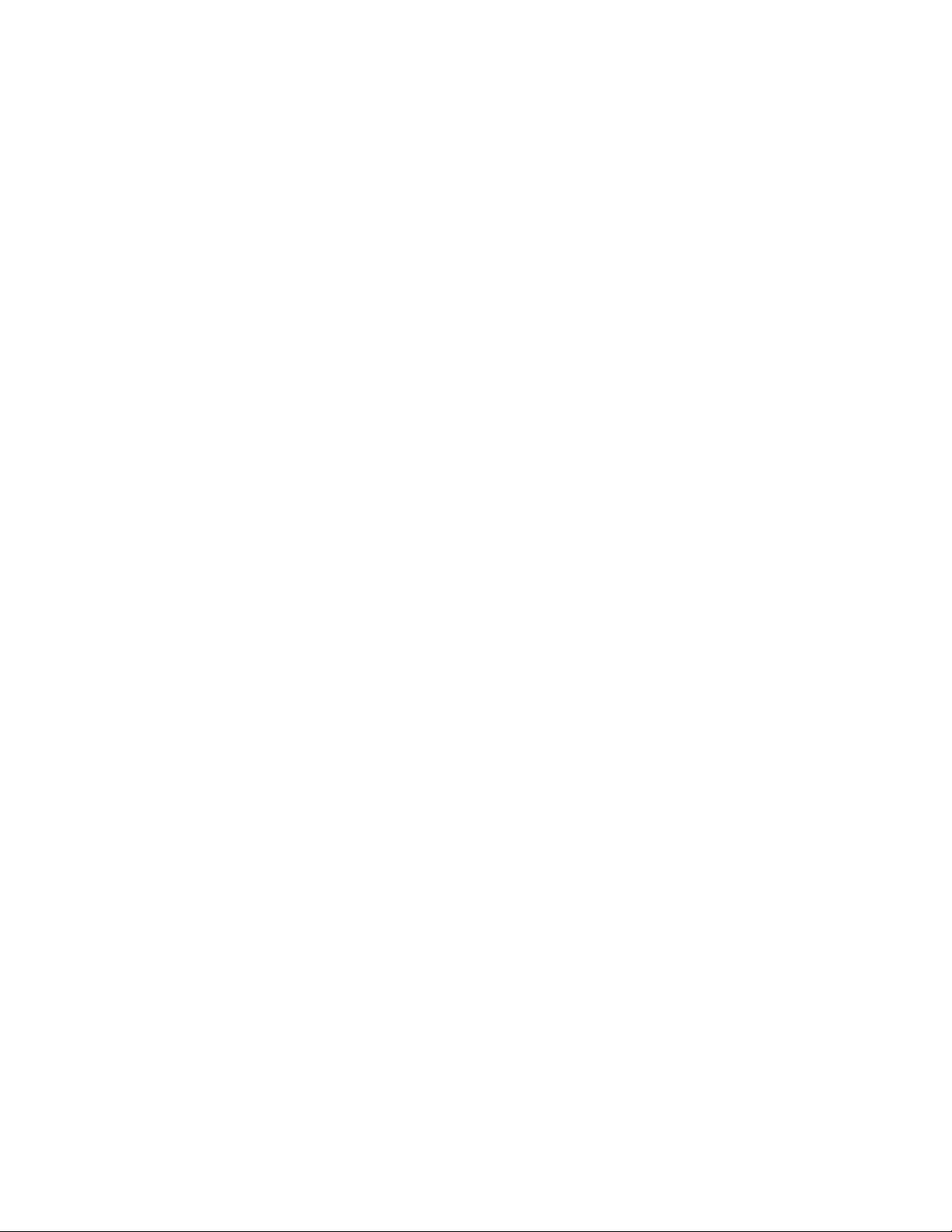
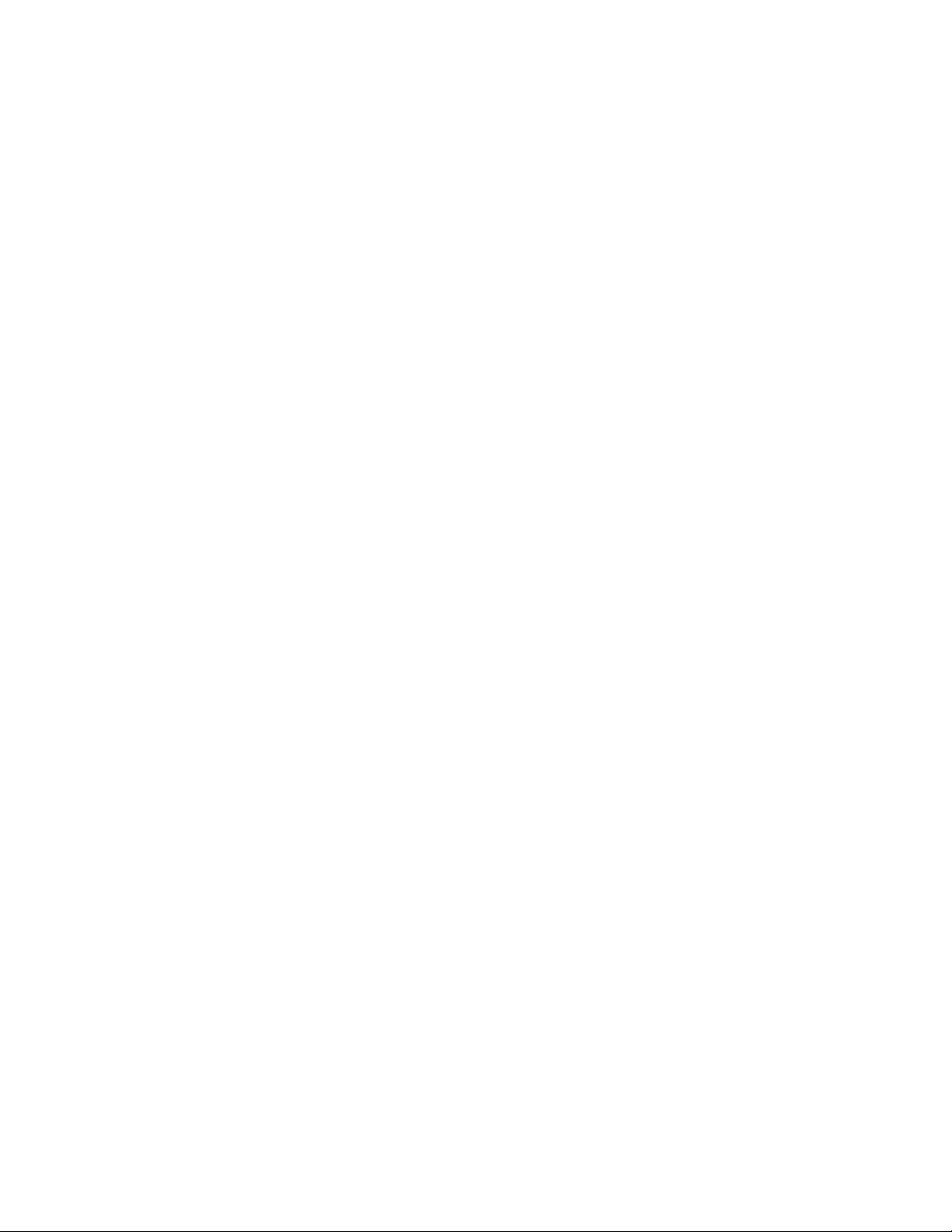
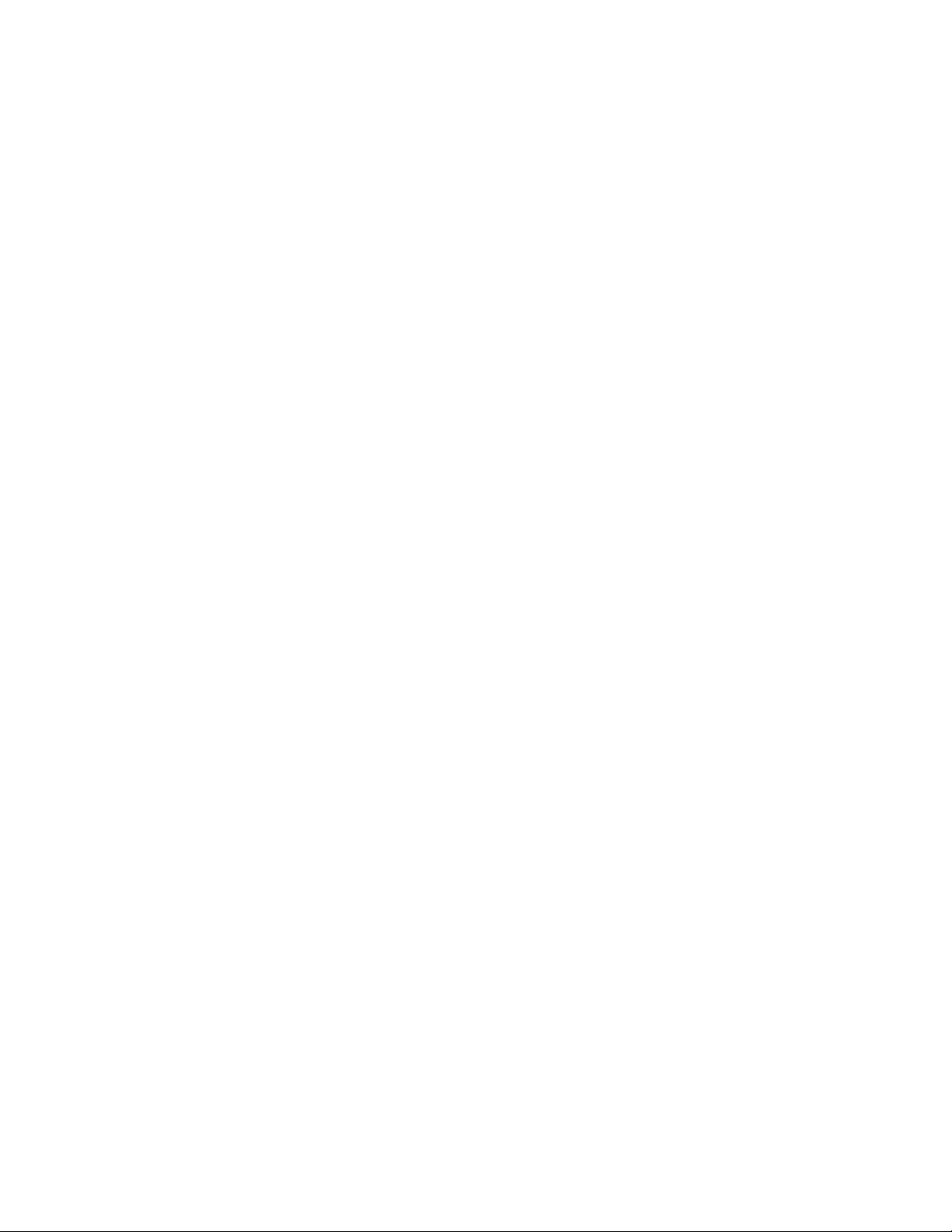
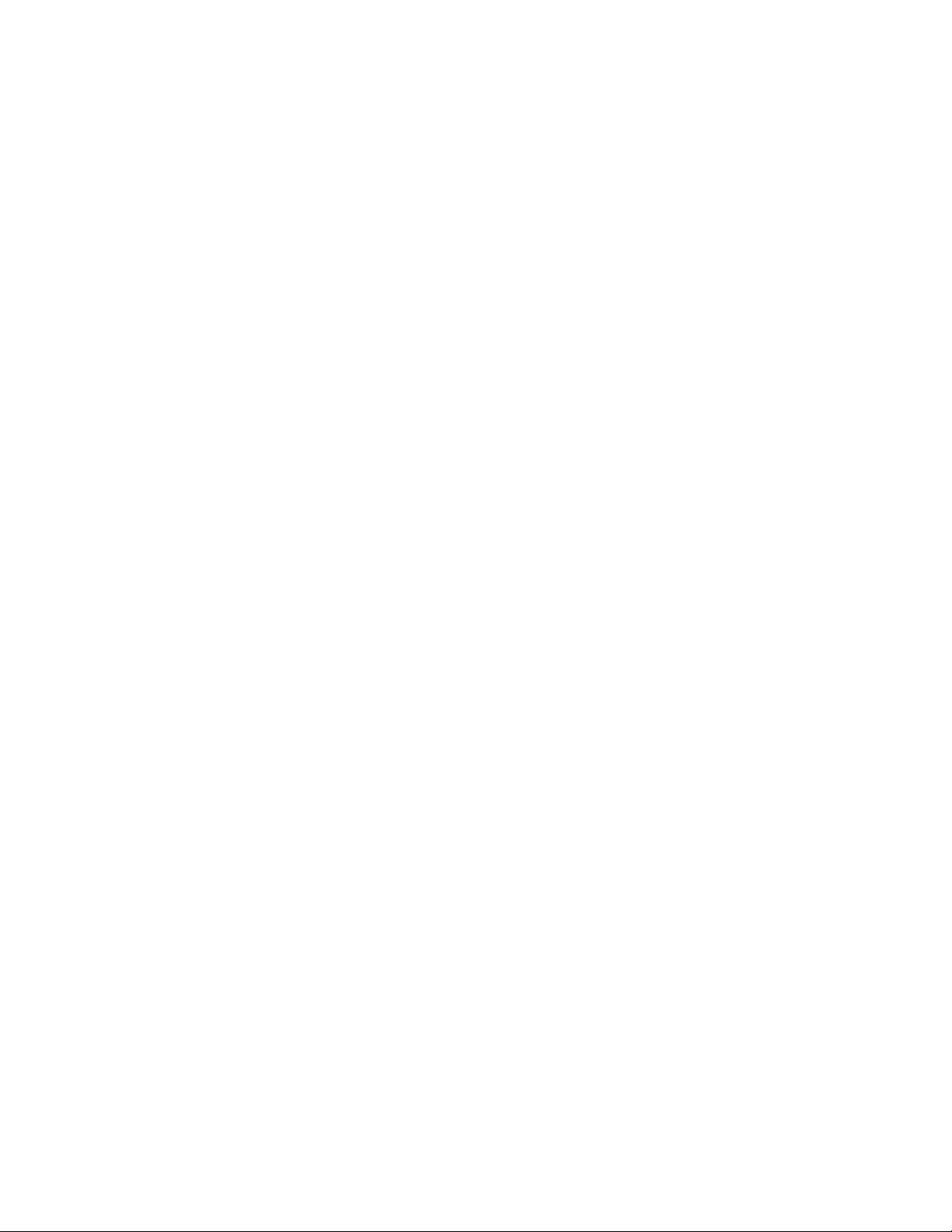
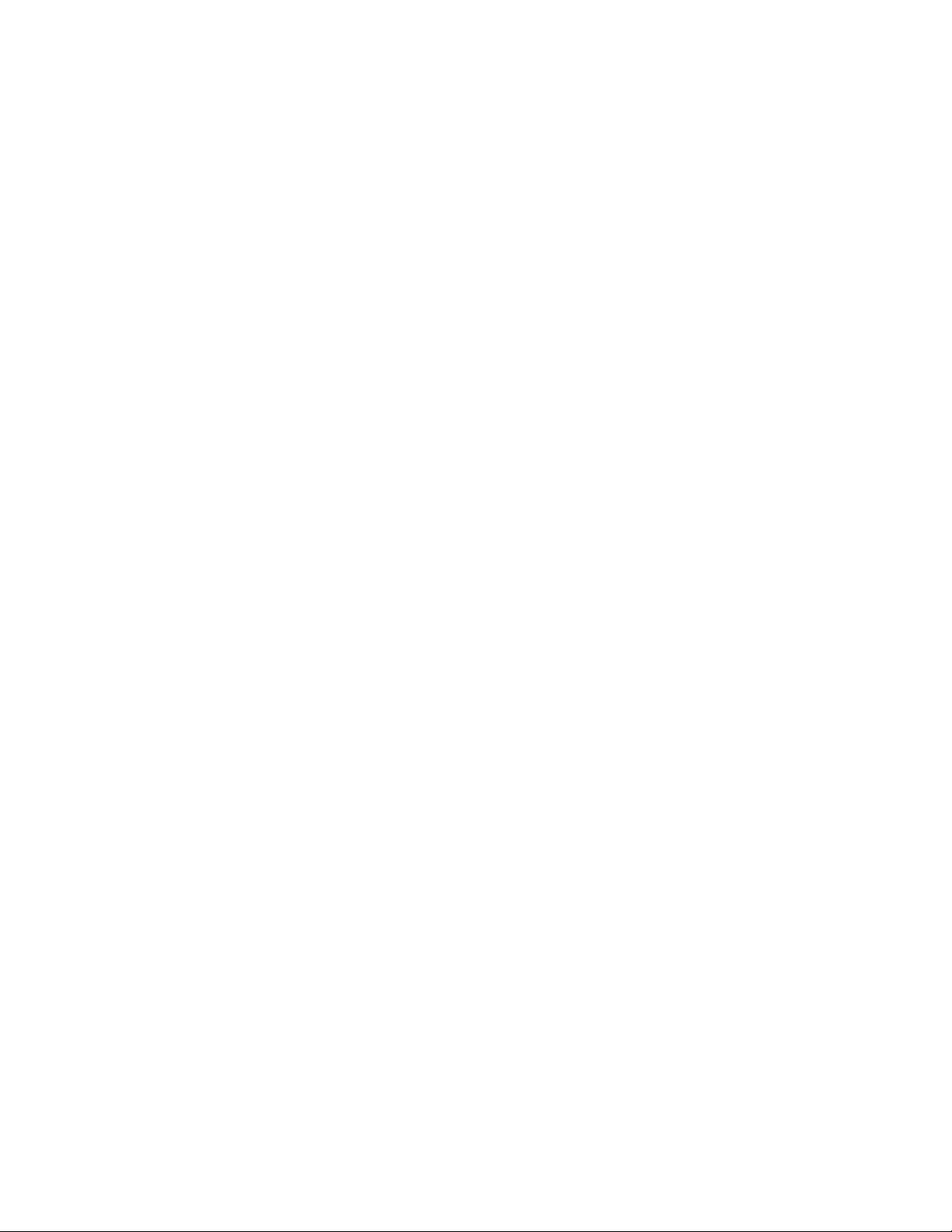
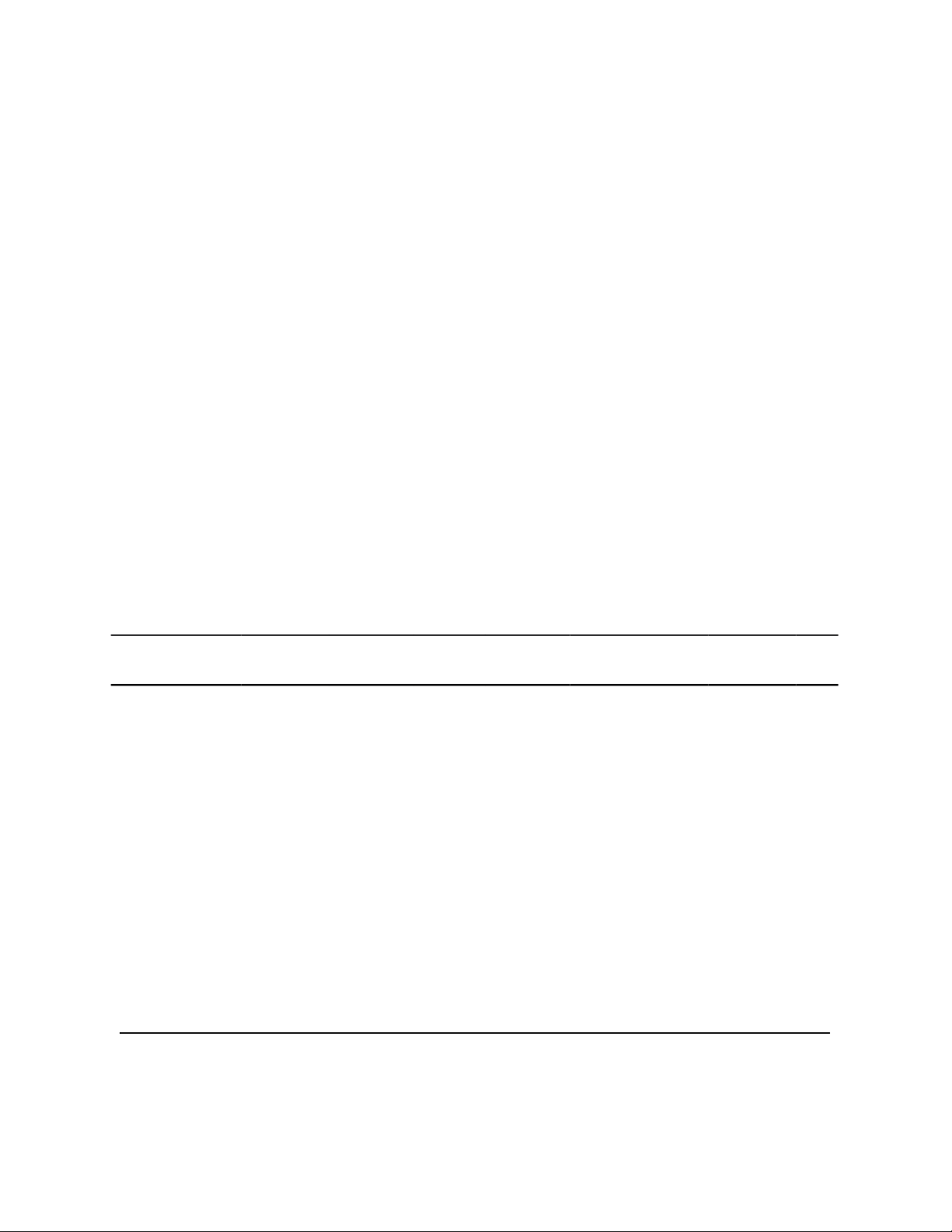
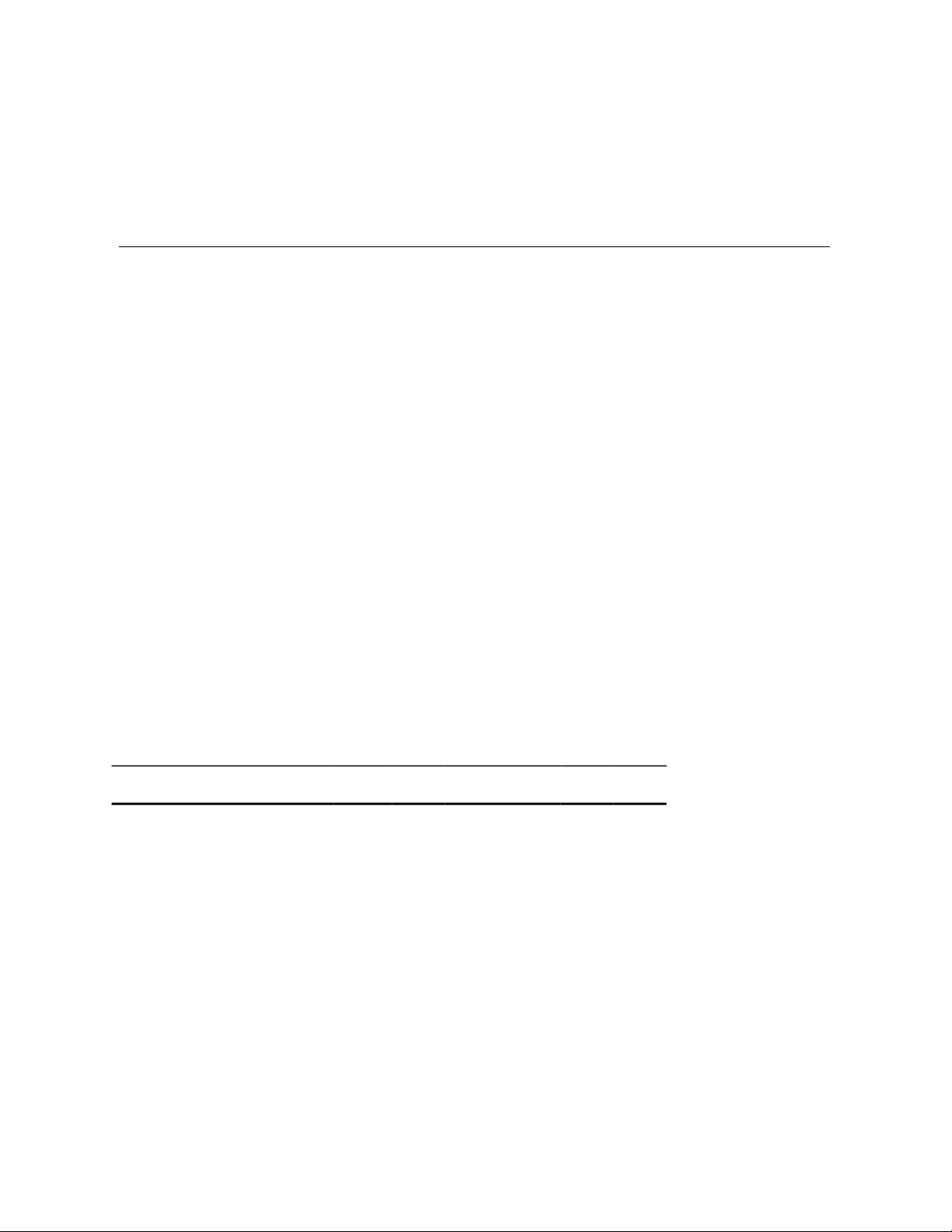
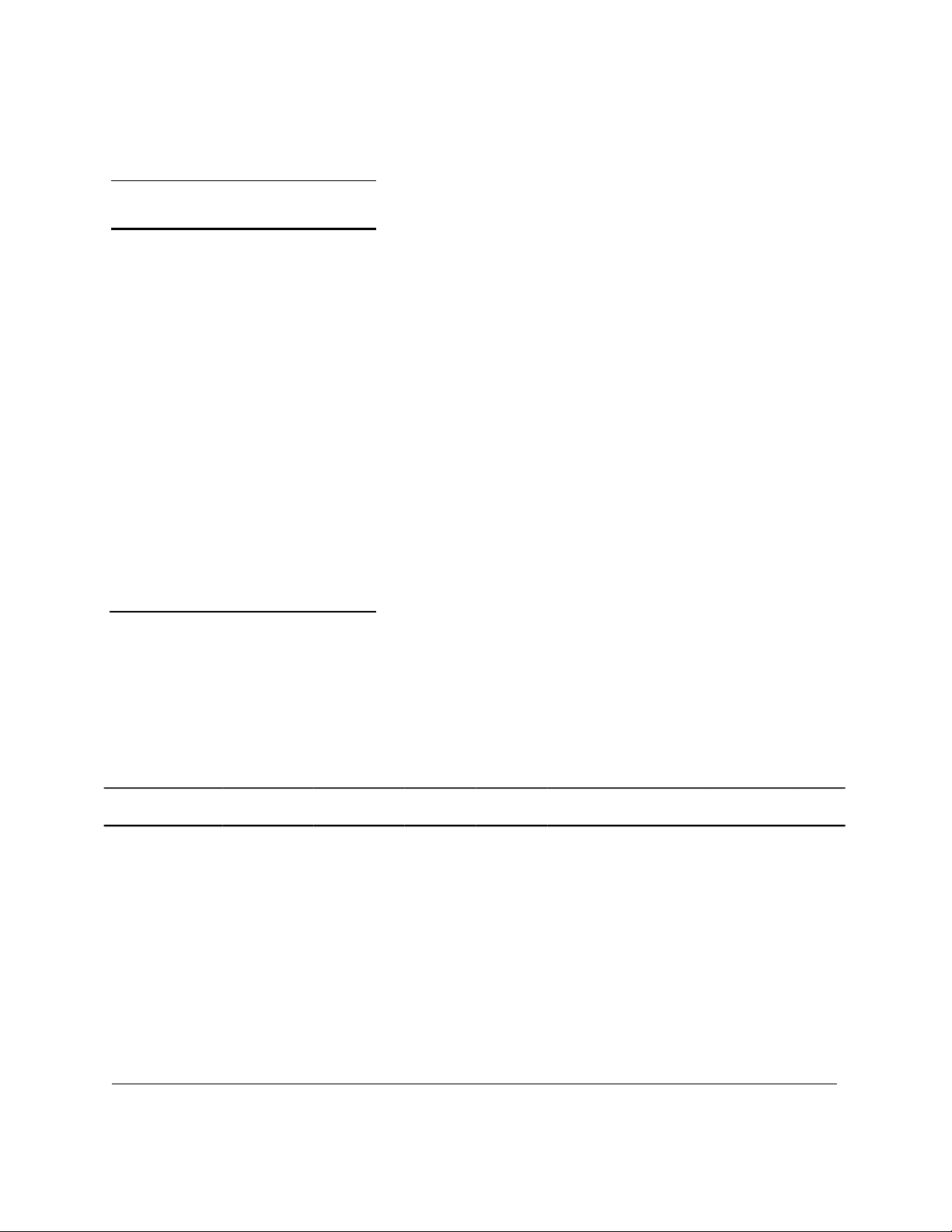
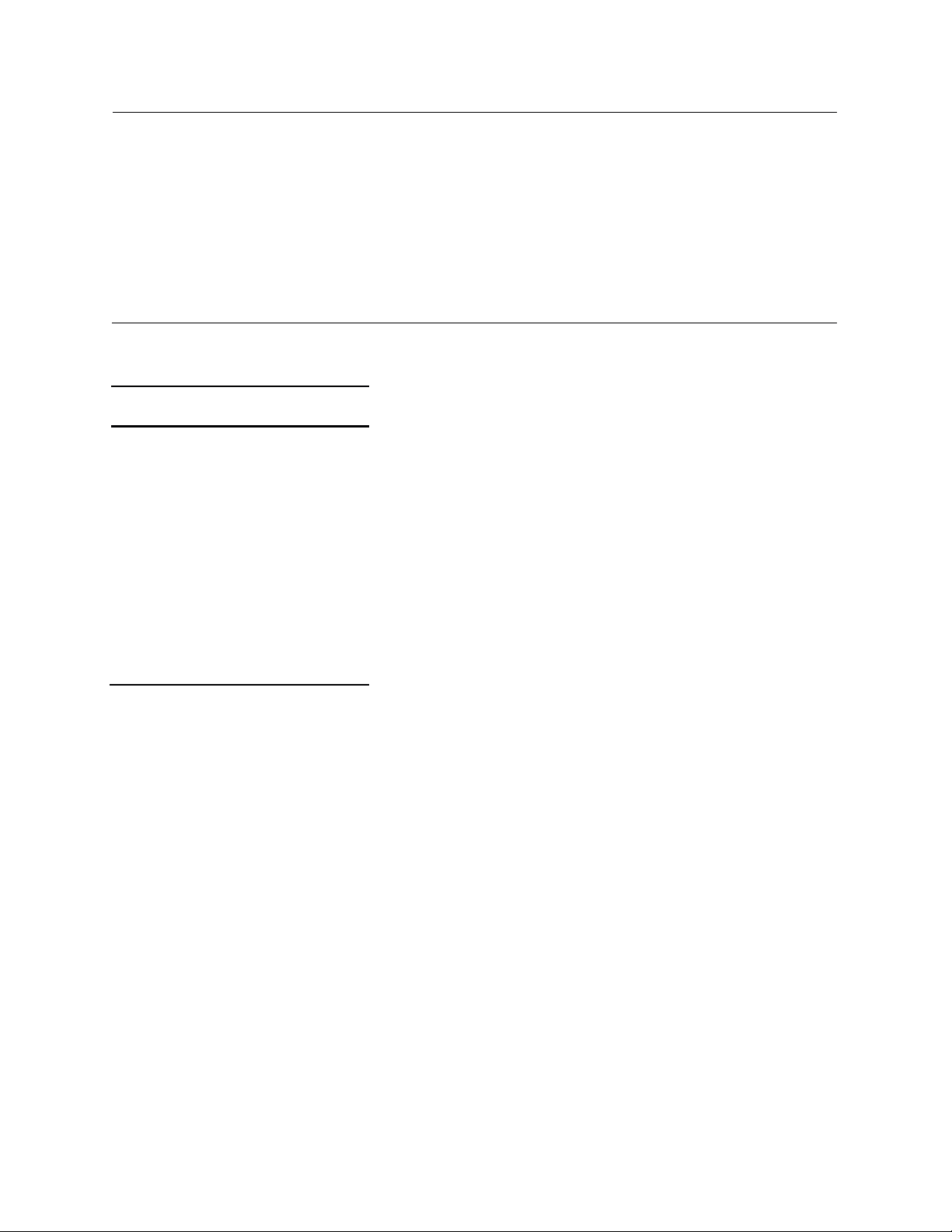
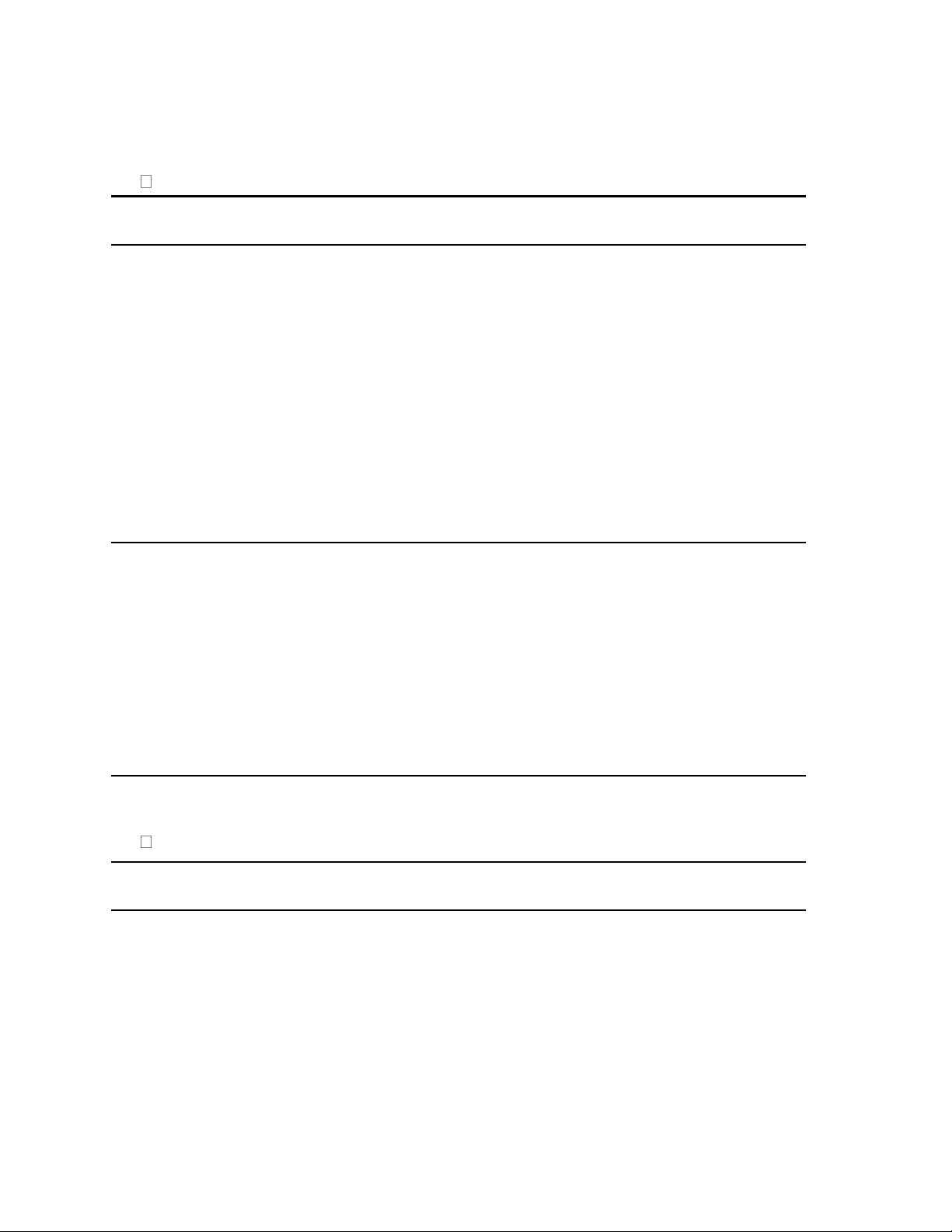
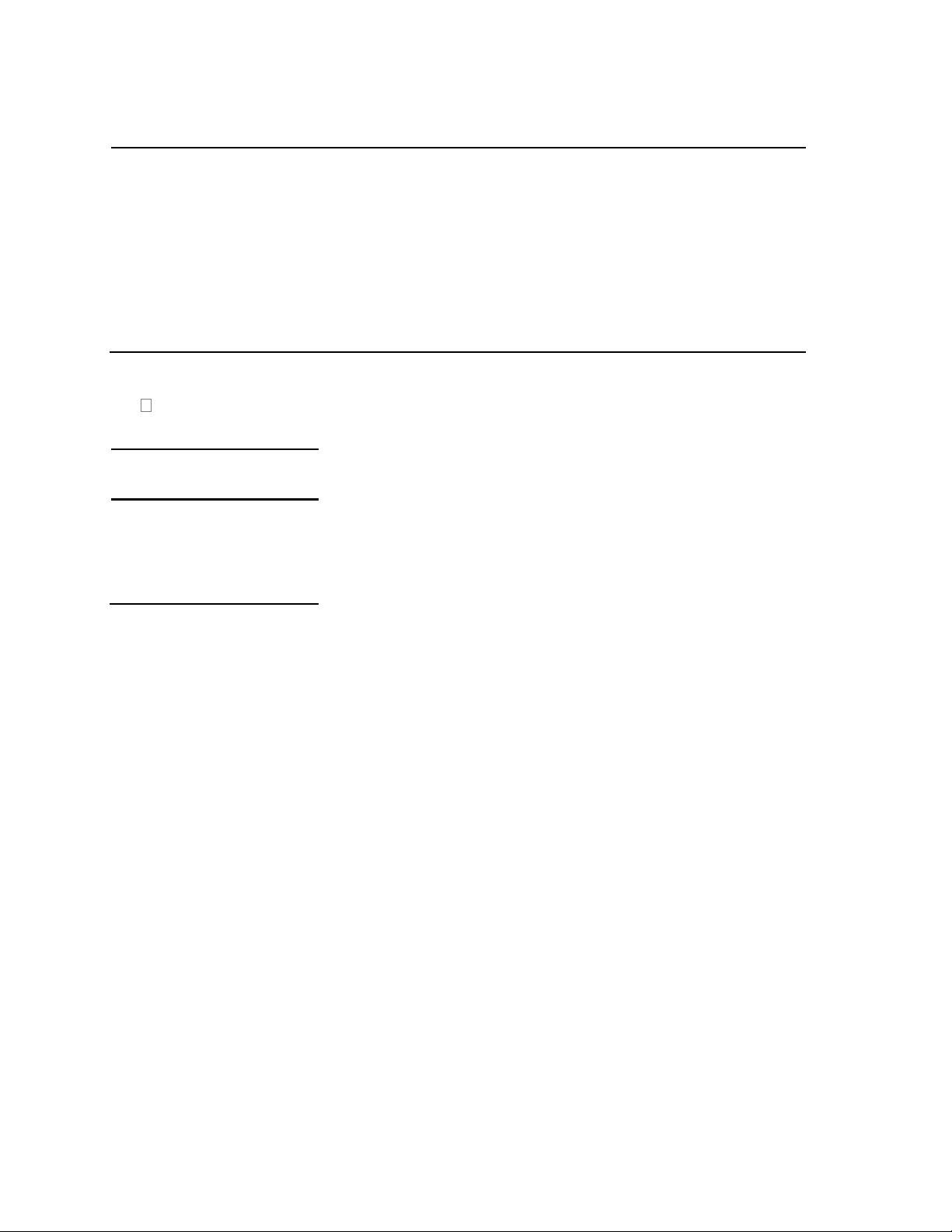
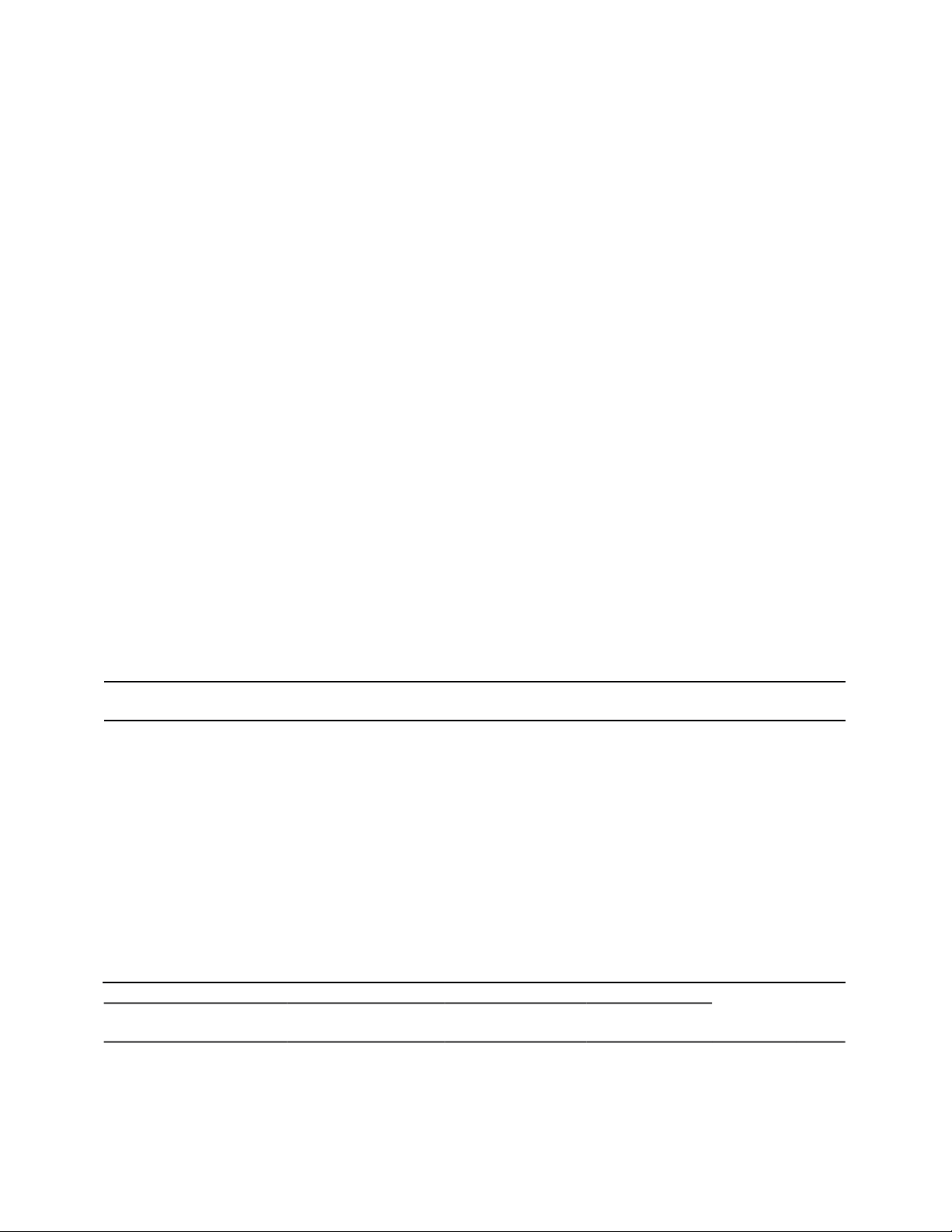
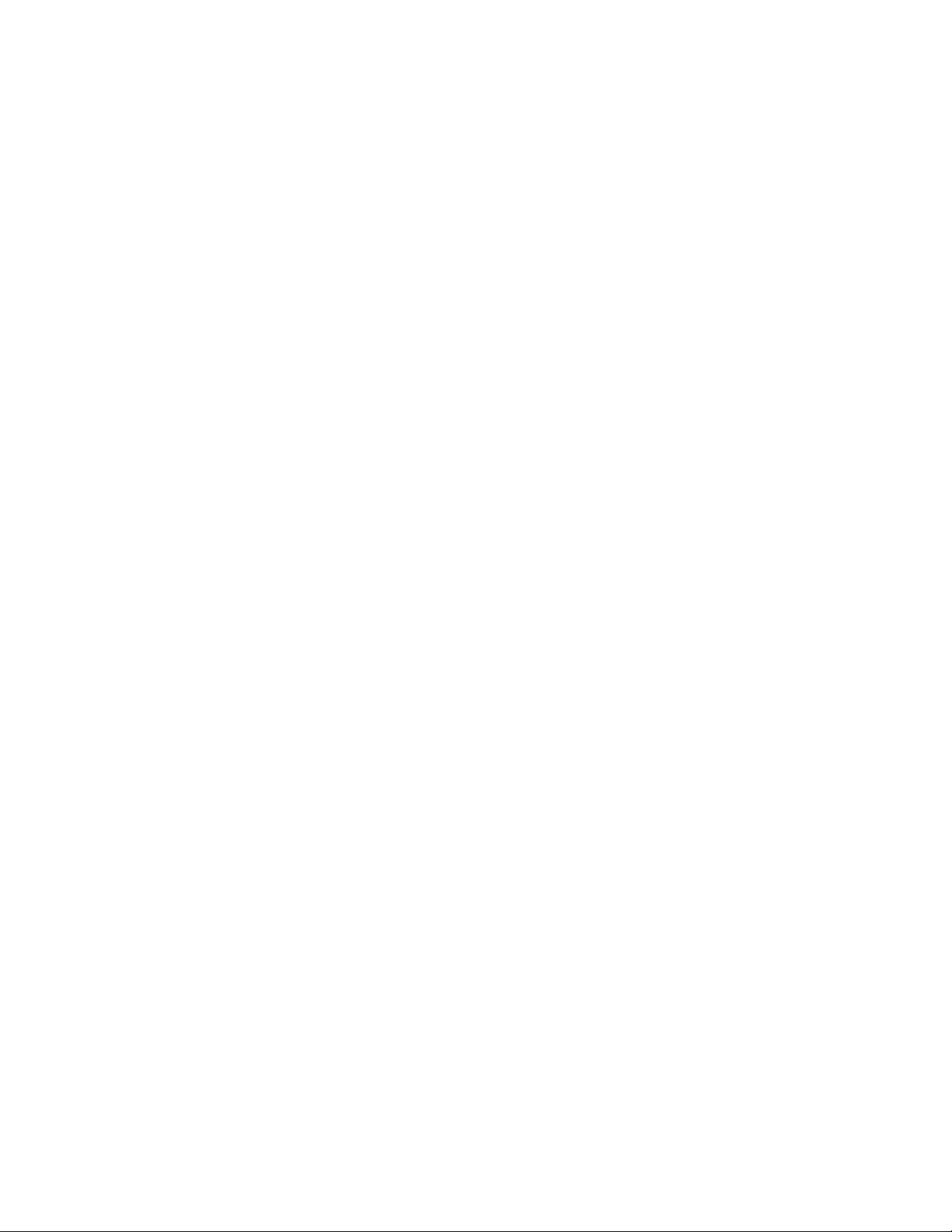
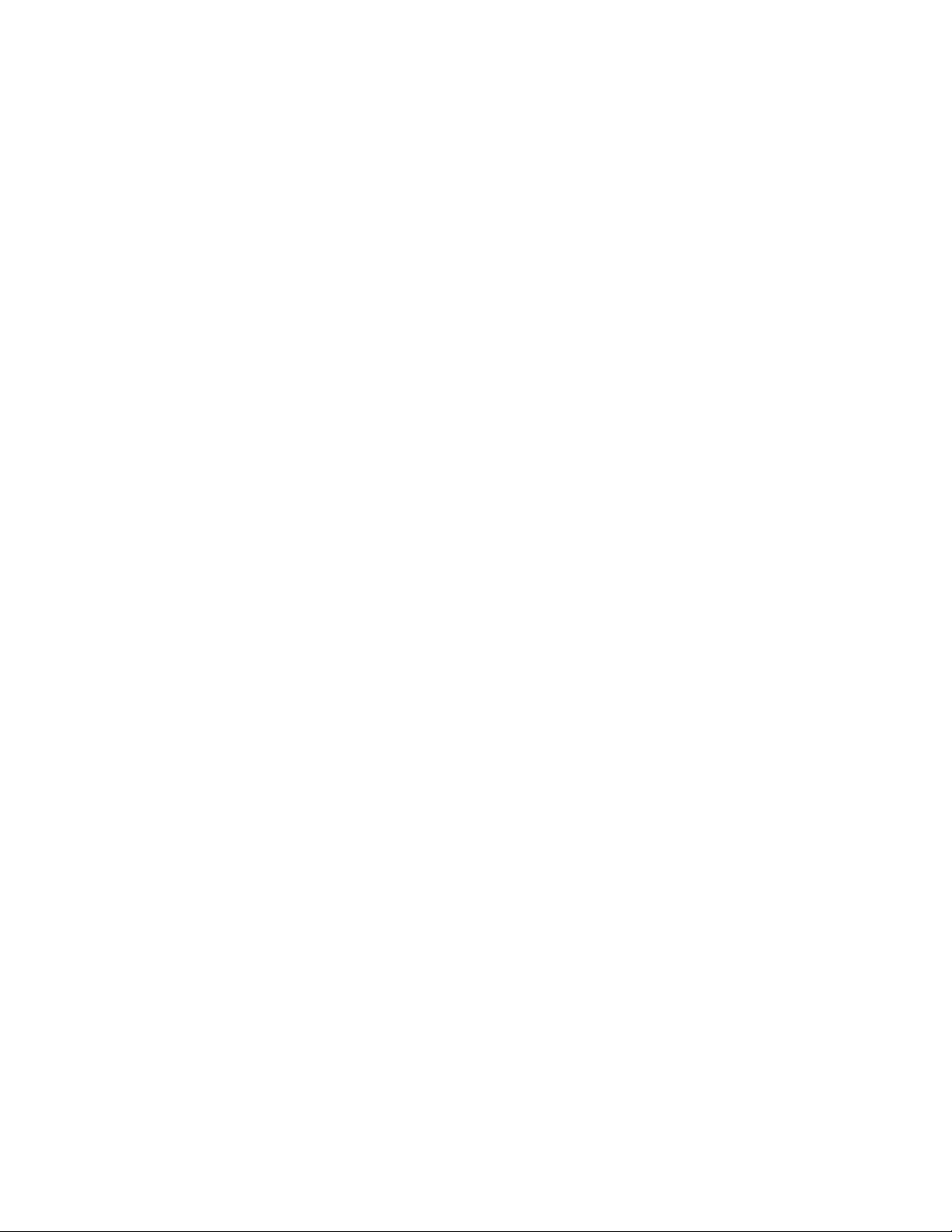
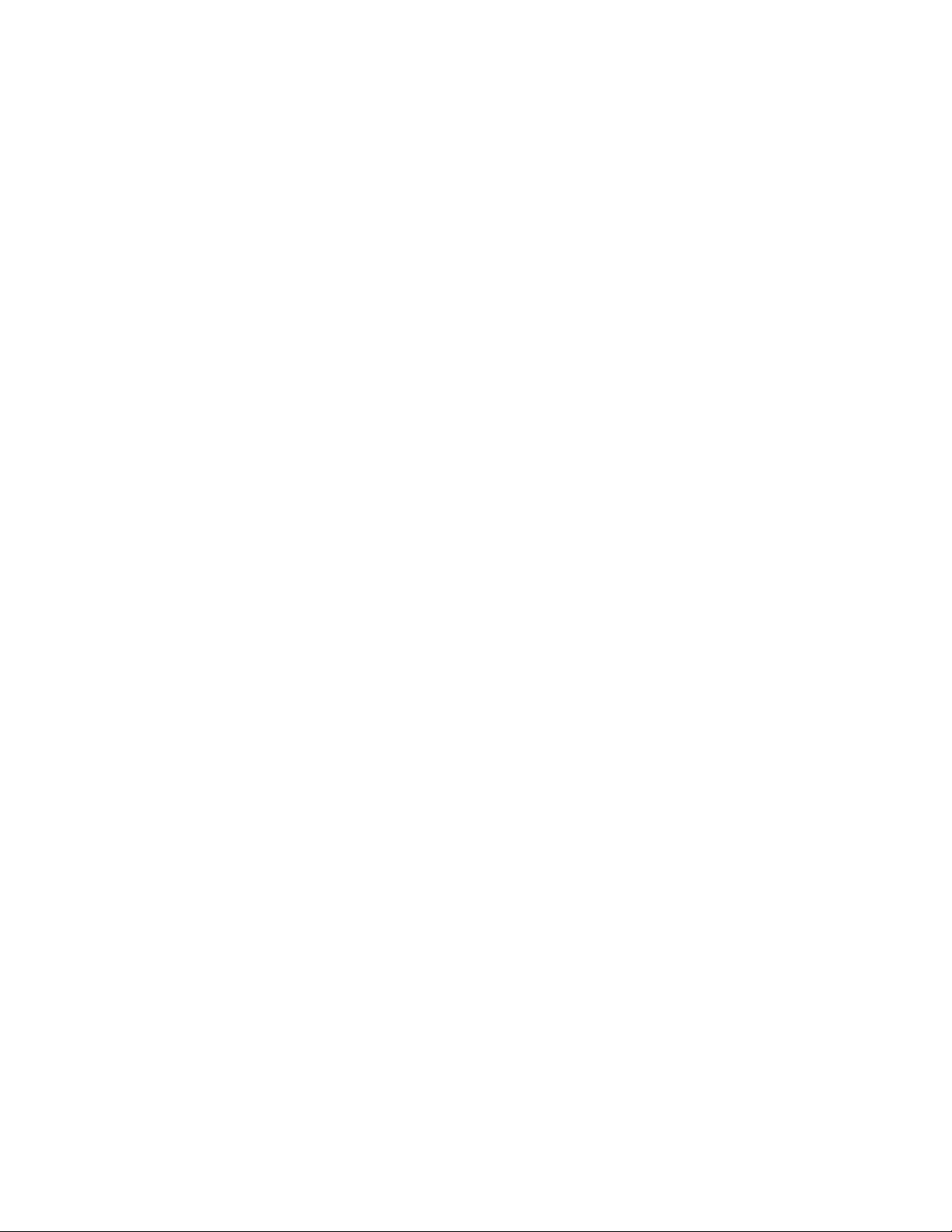
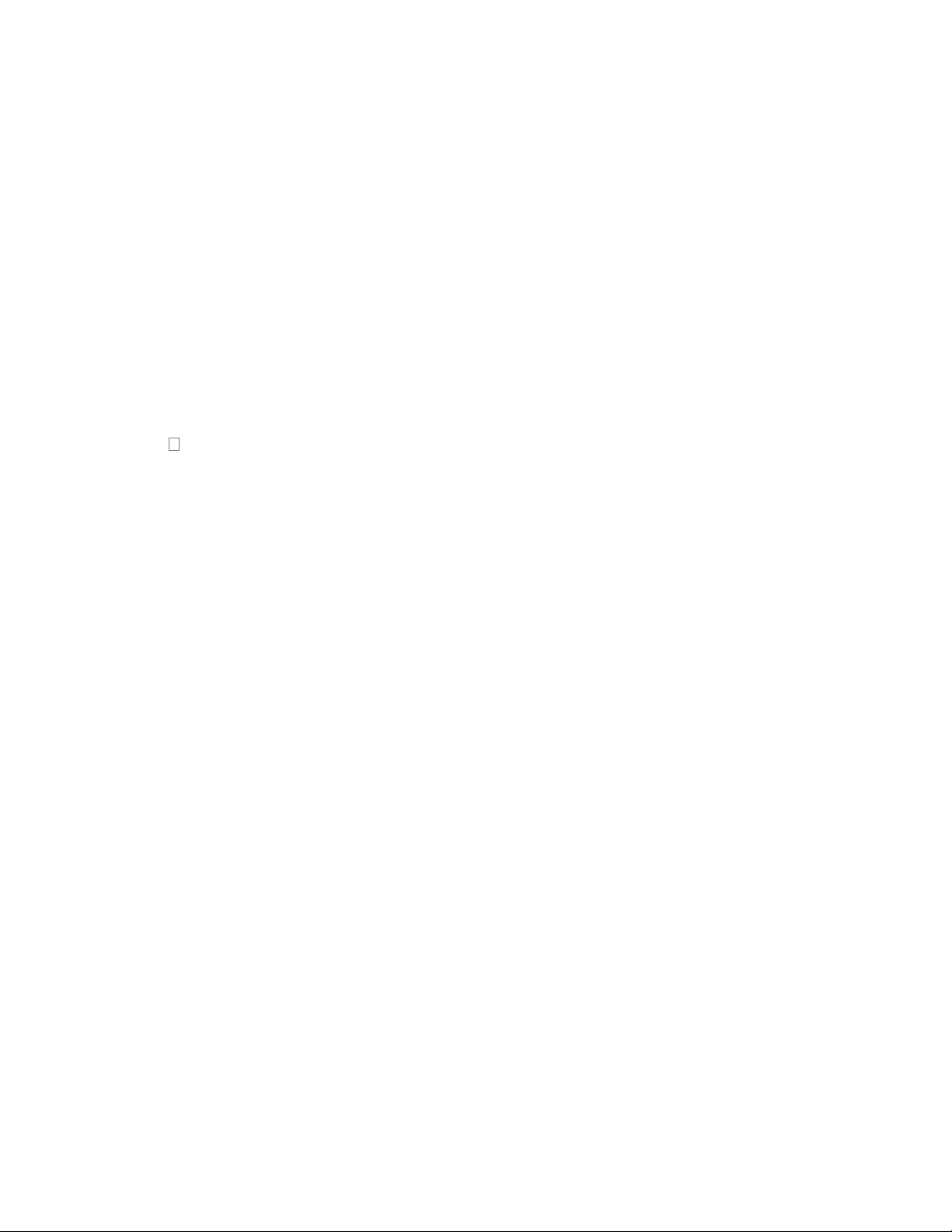
Tài liệu khác của Trường Đại học Quốc tế, Đại học Quốc gia Thành phố Hồ Chí Minh
Preview text:
VIETNAM NATIONAL UNIVERSITY – HO CHI MINH CITY
INTERNATIONAL UNIVERSITY
INTERNATIONAL FINANCIAL MANAGEMENT PROJECT
Topic: Key Economic Determinants of Foreign Direct Investment
(FDI) Inflows in G7 Countries Group 4 Võ Khải Điền BABAIU19020
Đặng Giang Bảo Như BABAIU19278
Nguyễn Thị Ngọc Sâm BABAIU19086
Nguyễn Trọng Anh Tài BABAIU19088
Phan Thùy Phương Thảo BABAIU19322
Ho Chi Minh City, June 10th, 2022 Table of Contents
ABSTRACT ....................................................................................................................... 3
I. INTRODUCTION ......................................................................................................... 3
II. LITERATURE REVIEW ............................................................................................ 4
1. Relationship between FDI inflows and Gross Domestic Product (GDP) .............. 4
2. Relationship between Unemployment Rate and FDI inflows ................................ 5
3. Relationship between the Balance of Trade (BOT) and FDI inflows .................... 5
4. Relationship between Trade Openness and FDI inflows ........................................ 6
5. Relationship between Inflation Rate and FDI inflows ........................................... 6
6. Relationship between Exchange Rate and FDI inflows .......................................... 6
III. METHODOLOGY ..................................................................................................... 7
1. Research Method ....................................................................................................... 7
2. Data Collection Method ............................................................................................. 7
3. Sampling Design ......................................................................................................... 7
4. Data Analysis .............................................................................................................. 8
4.1. Panel Data ............................................................................................................ 8
4.2. Ordinary Least Squares (OLS) Model .............................................................. 8
4.3. Multicollinearity Test .......................................................................................... 9
4.4. Homoscedasticity Test ......................................................................................... 9
4.5. Autocorrelation Test ............................................................................................ 9
4.6. Hausman Specification Test ................................................................................ 9
IV. EMPIRICAL RESULTS ........................................................................................... 10
1. Ordinary Least Squares (OLS) Model ................................................................... 10
2. Multicollinearity Test – VIF .................................................................................... 11
3. Hausman Specification Test .................................................................................... 13
4. Homoscedasticity Test .............................................................................................. 15
5. Autocorrelation Test ................................................................................................. 16
V. DISCUSSION .............................................................................................................. 17
VI. CONCLUSION ......................................................................................................... 18
REFERENCE .................................................................................................................. 19 2 ABSTRACT
Despite the fact that the FDI topic is frequently addressed in published scientific studies,
this subject has never been outdated. The study of FDI always delivers insightful
implications about economic and social features that are applied to attract FDI inflows. To
investigate the incentives for FDI inflows into developed countries, this study attempts to
analyze critical factors from 2002 to 2021 in Group Seven, which is known as G7 countries,
to find out key criteria for economic strength and opportunity for these advanced countries.
The empirical results indicate that the balance of trade, inflation rate and exchange rate
have positive relationships with FDI inflows, while GDP and GDP growth have
insignificant relationships. In addition, no relationship is found between inward FDI with
unemployment rate and trade openness. This study contributes to the existing literature on
the determinants of FDI in the context of G7 countries with new mixed results. I. INTRODUCTION
G7 is the group containing seven countries regarded as developed countries in the world,
namely the United States, United Kingdom, Germany, Canada, Japan, Italy, and France.
These countries are considered advanced economic nations belonging to one of the largest
organizations in the world named IMF. Until 2020, the aggregate group constitutes more
than half of the global total wealth, which was at $418 trillion (Global Wealth Databook
2021, Credit Suisse). These countries constitute 32 to 46 percent of global GDP, the value
is approximately 10 percent of the global population. The Group of Seven is also a powerful
group that holds major world powers in terms of politics, economy, military, legal, etc. Due
to their influencing power, these countries are regarded as advanced and developed
countries that have an influence on every world activity in general, and economic activity in specific.
Despite the fact that these countries are advanced economies, they cannot avoid the
negative impact of Covid-19 on a global scale. The Covid-19 pandemic has damaged
humanity and destroyed a whole financial and economic system, hurting trade flow and
business activity (Rakshit & Paul, 2020). Not outside of that influence, the group of seven
was severely hit, making the economic activity negatively affected. Among these countries,
the United Kingdom is the country that has been hit the most, which bears a significant
reduction in its GDP by -20.4% in Q2 from Q1 in 2019. However, the United Kingdom is
not the sole country in this group that endured significant declines in GDP, there were also
countries coming from European regions, and the United States that showed a huge decline
in its GDP (Deloitte, 2019). That raises the question of whether the G7 can maintain its
attractiveness to foreign investors for FDI inflows. However, these countries still managed
to maintain their economies and attract foreign direct investment inflows, especially the
United States (OECD). According to the data from OECD, in the group of G7, the United 3
States is the country having the largest FDI inflow among other countries (179,142 million
US dollars total Q4-2021); while other countries have a steady trend in their FDI inflow
from 2017 to 2021. That reflects the need to examine the differences behind FDI inflow in these countries over time.
Studying foreign direct investment (FDI) has been a hot topic in the worldwide economy,
and it has been regarded as significant in intellectual debate (Pan, 2002). Strong economies
like the G7 also need to be involved in the examination of the determinants that affect the
attractiveness of FDI inflow. Because the FDI inflows into any nation are often regarded
as a significant indicator of economic development and opportunity. Nevertheless, the
patterns of FDI inflow differ for each nation. This is mostly due to disparities in nations'
economic, social, and political infrastructure (Adhikary, 2017). The attraction of FDI to
promote an economy is determined by a variety of crucial domestic variables. Most
academics believe that market size, human capital, trade openness, inflation rates, and
currency rates are the most important variables influencing FDI inflows into a nation
(Asongu et al., 2018; Ergano & Rambabu, 2020; Kisto, 2017; Kumari & Sharma, 2017).
This study mainly focuses on the economic determinants of inward FDI into G7 countries,
including GDP, GDP Growth, unemployment rate, balance of payment (BOP), trade
openness, inflation rate and exchange rate. II. LITERATURE REVIEW
1. Relationship between FDI inflows and Gross Domestic Product (GDP)
Gross Domestic Product (GDP) is an economic variable that measures the value of all
products and services produced within a country's boundary in a particular period. This
variable indicates the level of life of a whole country; in other words, it is a country's living
standard. The relationship between FDI and GDP is still a controversial topic; however, it
is undeniable to say FDI and GDP have an impact on each other with strong evidence. GDP
growth is also used to determine the productivity of a country, which measures a whole
economy’s output or final goods and services. Based on the theory of growth, there are
several factors that may be used to foster or prevent a country from receiving FDI, these
factors are regarded as stimulants for a country's GDP growth (World Investment Report
UNCTAD, 1994), such as the size of capital investment, sophisticated technologies, well-
constructed infrastructures, political stability, skilled labor, tax rates, and beneficial
regulatory regimes. Differences in the distributions or levels of certain variables can affect
and create differences in growth rates among countries (Dondeti and others). When these
variables are in low supply, governments may rely on non-resident investors from other
countries to disperse these resources in the form of foreign direct investment (Sawyer &
Sprinkle, 2006; Lipsey & Chrystal, 2006). However, when bringing these resources, there
must be further studies to prove the effectiveness of this action to a host country. According 4
to a study conducted by Moran, Graham and Blomstrom (2005), they studied several
studies to determine if the GDP growth is positively affected and enhanced by an inflow of
FDI. These researchers drew a conclusion that the impact of FDI on a host country’s GDP
growth is heavily influenced by the host-economic country’s openness. When a country
further liberates its economy, the country is likely to receive FDI inflow from foreign
investors. Furthermore, according to Seetanah and Khadaroo (2007), FDI not only
generates GDP growth, while its contribution is minimal when compared to other growth
variables, but it also goes along with economic growth. Another study that indicates the
relationship between FDI and GDP is the study conducted by Nonnemberg and Cardoso de
Mendonça (2004) discovered that while high GDP growth might stimulate FDI inflows,
FDI does not always cause economic growth. Therefore, to figure out and examine again
the impact of GDP and GDP growth on FDI, this study proposes the following hypotheses
regarded as H1: GDP does not have a significant impact on FDI inflows of country i at time t.
H2: GDP growth does not have a significant impact on FDI inflows of country i at time t.
2. Relationship between Unemployment Rate and FDI inflows
Unemployment rate is perceived as a proxy for macroeconomic stability or labor
availability (Botric & Skuflic, 2006). It is widely known that a country observing high
unemployment rates will be considered an inappropriate destination for foreign investors
(Brozen, 1958). However, a number of researchers have recently supported the positive
influence of the high unemployment rate on FDI inflows (Friedman et al., 1992;
Nunnenkamp et al., 2007; Chidlow et al., 2009; Strat V. A. et al., 2015). Blanchard (2011)
argues that countries with high unemployment rates can catch the eyes of foreign investors
due to available workforce abundance and the labor’s willingness to receive lower wages.
Specifically, high unemployment figures will manipulate employed people to place higher
value and put more effort on their current or potential future jobs and make people who
have already fallen into unemployed status more likely to accept lower wages to earn a
living (Boateng, 2015). Therefore, with all of the preceding considerations, the following hypothesis is formulated:
H3: Unemployment rates will exert a positive impact on FDI inflow of country i at time t.
3. Relationship between the Balance of Trade (BOT) and FDI inflows
A large deficit in the balance of payments implies that a country is currently living beyond
its means, which possibly raises the restriction of free capital movement and the difficulty
in profits transfer from the direct investments into the investing country (Schneider, 1985).
Balance of trade, which limits the difference between export and import of goods, may
have the same effect on FDI as the balance of payment. The empirical results from Rahmen
(2015) also show that BOT and FDI inflows have a strong negative relationship. Given the
above logic, the following hypothesis is constituted: 5
H4: Balance of trade has a negative impact on FDI inflows of country i at time t.
4. Relationship between Trade Openness and FDI inflows
Trade openness implies the restriction level controlled by the host country's government
on trade activities (Aziz & Mishra, 2015). An economy’s liberal trade degree is a critical
indicator of the import and export relationships that one nation is willing to have with
another (Asiedu and Lien, 2004). In addition, a greater extent of trade openness also brings
more investment opportunities and enhances the network between domestic and
international markets (Tintin, 2013). Empirical studies have widely reached a consensus
that trade openness positively promotes FDI (Morrisset, 2000; Chakrabarti, 2001;
Noorbakhsh et al., 2001; Asiedu, 2002; Biglaiser & deRouen, 2006; Na & Lightfoot, 2006;
Cevis & Camurdan, 2007; Quazi, 2007; Demirhan & Masca, 2008; Bagli & Adhikary,
2014), especially efficiency-seeking FDI (Ramasamy & Yeung, 2010). It is claimed that
trade openness increases competition, which ultimately fosters efficiency, technical
transformation and product improvements, and decreases production costs. These positive
conditions will stimulate economic growth via raising profits, thus encouraging more
foreign capital investment and expertise inflows (Nishimizu & Robinson, 1986; Nishimizu
& Page, 1991; Tybout, 1992; Helleiner, 2002). Therefore, trade openness, with no harsh
control in terms of taxes, quotas or state monopolies on exports, can promote a business-
friendly economic climate and attract more investment, thus resulting in greater FDI
inflows (Boateng et al., 2015). With all of the foregoing discussions, the following hypothesis is proposed:
H5: Trade openness has a significant impact on FDI inflows of country i at time t.
5. Relationship between Inflation Rate and FDI inflows
Inflation rate – an indicator of macroeconomic stability, reflects the internal economic
situation and the government and central bank’s capability to restrict the money supply and
balance the national budget (Buchanan, 2011; Boateng et al., 2015). A low inflation rate is
desirable as it represents internal national economic stability, which will attract foreign
investors and increase FDI inflow (Balasubramanyam, 2002). In contrast, a high inflation
rate will suffer inward investing firms by decreasing the real value of earnings in the form
of local currency (Buckley et al., 2007). It is widely accepted that the inflation rate
negatively affects FDI inflow (Cevis & Çamurdan, 2007; Rodriguez & Pallas, 2008;
Demirhan & Masca, 2008). Therefore, the following statement is hypothesized:
H6: High inflation rate has a negative impact on FDI inflows of country i at time t.
6. Relationship between Exchange Rate and FDI inflows
Exchange rate, defined as the domestic currency price of a foreign currency can affect FDI
through relative production cost or wealth effect (Tolentino, 2010). A country experiencing 6
currency depreciation will accordingly observe its wages and production costs less
expensive than its foreign counterparts (Lartey et al., 2014), which raises the export-
oriented FDI profit and further attracts FDI inflow (Boateng, 2015). In addition, the
reduction in production inputs costs such as labor, land, machines and other resources
following the currency devaluation will encourage foreign investors to acquire more host
country’s assets at a cheaper price (Blonigen, 1997). This effect has led to investors’
tendency to postpone their investments when the host country’s currency is appreciating to
maximize the profits at a later phase (Barrell & Pain, 1996). While there is a viewpoint that
future profits to be exchanged from the acquiring firm’s subsidiary back to the home
country where the currency strengthens will have a lower discount value (Kish &
Vasconcellos, 1993), most the empirical studies show a positive correlation between
currency depreciation and increased FDI inflows (Caves, 1989; Froot & Stein, 1991;
Ahnetal, 1998). Given the above discussions, the following hypothesis is stated:
H7: Exchange rate positively impacts FDI inflows of country i at time t. III. METHODOLOGY 1. Research Method
According to the characteristics of the research, which concentrate generally on statistical
data and calculations in order to determine which factors have a strong impact on foreign
direct investment inflows of selected countries, the quantitative method will be the suitable approach.
2. Data Collection Method
The research will use the secondary data of some economic indicators affecting the inflows
of foreign direct investment in selected countries. More to mention, the secondary data
used in this research is mainly collected from several platforms, namely Eikon, World Bank
Open Data, Trading Economics, etc. 3. Sampling Design
The sample group targeted for the data collection process consists of the nations in the
Group of Seven (G7) forum, including Canada, France, Germany, Italy, Japan, the United
Kingdom, and the United States. These countries are well-known for their high FDI inflows.
Selecting them can help us to have an insight into independent variables affecting the FDI
inflows from other countries around the world. To be more specific, each G7 country will
be provided with data corresponding to each chosen variable. In that, the time interval for
the data will be quarterly, from the year 2002 to 2021. 7 4. Data Analysis
After completing the process of data collection, the inspection of assembled data and
demonstration of descriptive statistics should be conducted respectively. Several diagnostic
tests must also be completed to assess the reliability and the validity of the obtained data
(normality, multicollinearity, homoscedasticity, autocorrelation, Hausman specification).
Moreover, another crucial statistical method called Regression Analysis will be applied to
authenticate the proposed hypothesis. During the Regression Analysis, there will be three
major regression models applied to examine the impact of each factor on the FDI inflows
in G7 countries. These three models include the OLS (ordinary least square) model, the
fixed-effect model, and the random effect model. Finally, during the research, Stata 14.0
will be utilized to construct and examine the data, which can be served to evaluate the findings. 4.1. Panel Data
As mentioned above in the Sampling Design section, the data used for the study is the
unbalanced panel data of all nations in the G7 forum, in which all the cross-sections spread
over the period, quarterly, from 2002 to 2021. Specifically, France’s data is collected from
2008, and Canada’s data is collected from 2004. 4.2.
Ordinary Least Squares (OLS) Model
Ordinary Least Square, an abbreviation of OLS, is a sort of linear least square approach
used for evaluating the undetermined parameters inside a linear regression model. In Stata,
the OLS model needs to be performed before running any other statistical models or tests.
To ensure the reliability and validity of the dataset, the below values should be evaluated:
• If the probability (Prob) is less than 0.05, it means that the data is fit for analysis (fit model).
• R-squared value means the percentage of the independent variables explaining the dependent variable.
• Coefficient (Coef.) means the relationship between independent variables and
dependent variable. The relationship between them can be a positive impact or
negative impact (illustrated by the minus sign before the value).
• The p-value (P) illustrates the significance of the independent variables on the
dependent variable. If the p-value is less than 0.05, then that independent variable
is significant to the dependent variable. 8 4.3. Multicollinearity Test
When some of the independent variables are related to each other or can be generated from
a single variable, the regression equation can witness an additional impact because some
of the variables are identical or equivalent; as a result, the predicted value can be
overestimated (Kothari, 2015). This phenomenon is called multicollinearity. This unwanted
condition can be recognized by performing variance inflation factor (VIF)
If the VIF value of the variable is smaller than 3, then the multicollinearity can be ignored
and that variable is eligible for analysis. On the other hand, if the VIF value is above 3, we have to adjust the model. 4.4. Homoscedasticity Test
Homoscedasticity, also known as homogeneity of variances, is the concept that variances
in various groups are equivalent or similar.
When the variance given by the residuals is not a constant, in this case, the residual variance
can be considered heteroscedasticity. An approach that can be used to discern
heteroscedasticity in Stata is the Hettest or Breusch – Pagan test.
As Kothari (2015) cited, the null hypothesis is that the residuals' variance is homogeneous.
The p-value will be extremely low in this situation (almost negligible). In such instances,
denying the hypothesis and accepting the second hypothesis of nonhomogeneous variance is the preferable option. 4.5. Autocorrelation Test
Autocorrelation is a statistical phenomenon where the observational error at time t is related
to the observational error at time t - 1 or at any other time in the past. In Stata, to recognize
this condition, we can apply the Wooldridge test for panel data. If the p-value of the test
result is lower than 0.05, then the tested model has autocorrelation. 4.6.
Hausman Specification Test
Hausman specification test is a diagnostic test to know which regression model (random
effect or fixed effect) will be more suitable for the research. When the test’s results are
established, if the probability is more than 5%, it indicates that the chosen model is the
appropriate one for the research.
4.6.1. Random Effect Model
The random effect model is a regression model where several parameters (impacts) which
determine the model’s structural elements vary randomly. The random-effect model is
justified by the fact that, unlike the fixed-effect model, variation among entities is believed 9
to be unpredictable and uncorrelated with the predictor or independent variables included
in the model (Torres-Reyna, 2007). If the probability shown in the model is less than 5%,
it means that the model is qualified for analysis.
4.6.2. Fixed Effect Model
The fixed effect model is also a regression model but opposes to the random-effect model,
in which the constants are handled as group-specific, which means each group has its own
set of constants (Gupta and Singh, 2016). When the researchers merely prefer to look at
the impact of factors that change over time, they should use fixed-effect model. The fixed-
effect model has the disadvantage that it cannot be applied to explore timeinvariant factors
of dependent variables (Torres-Reyna, 2007). If the probability shown in the model is less
than 5%, it means that the model is qualified for analysis.
The Hausman specification test will then be conducted to select which model is more appropriate. IV. EMPIRICAL RESULTS
1. Ordinary Least Squares (OLS) Model
The table illustrated below is the results of the ordinary least squares performed on Stata. Linear regression fdi inflow
Coef. St. Err. t-value p-value [95% Conf Interval] Si g unemployment - 2.698e+11 -0.63 .528 -7.002e+11 3.599e+11 rate 1.702e+11 inflation rate 1.945e+12 8.236e+11 2.36 .019 3.272e+11 3.563e+12 ** trade openness 4.895e+09 2.872e+10 0.17 .865 -5.152e+10 6.131e+10 gdp growth rate - 3.122e+11 -0.27 .788 -6.975e+11 5.292e+11 8.412e+10 exchange rate - 1.851e+09 -2.56 .011 -8.364e+09 - ** 4.729e+09 1.093e+09 gdp .003 0 6.78 0 .002 .003 *** BOT .104 .015 6.69 0 .073 .134 *** Constant -0.51 - .612 -8.335e+10 4.912e+10 3.372e+10 1.712e+10 Mean dependent 138364220109.656 368325151633.373 SD dependent var var 10 R-squared 0.731 Number of obs 532 F-test 203.519 Prob > F 0.000 Akaike crit. (AIC) 29162.721 29196.934 Bayesian crit. (BIC)
*** p<.01, ** p<.05, * p<.1
As can be obviously seen from the result table, the p-value (Prob), which is 0.000, is smaller
than 0.05. Hence, this value indicates that the data is suitable for analysis (fit model). The
R-squared value equals 0.731. In other words, the independent variables can explain about
73.1% variance in the dependent variable.
Observing the coefficient (Coef.) column, we can conclude that unemployment rate, GDP
growth rate, exchange rate has negative impacts on FDI inflows; while inflation rate, trade
openness, gross domestic product, and balance of trade have positive relationships with FDI inflows.
The p-values talk about the significance of the independent variables with the dependent
variable. If the p-value is less than 0.05, then that independent variable is significant to the
dependent variable. As can inevitably be seen from the table, there are three independent
variables which have insignificant connection with FDI inflows, including unemployment
rate (p-value = 0.528), trade openness (p-value = 0.865), and GDP growth rate (p-value = 0.788).
2. Multicollinearity Test – VIF
In the research, we use this test to point out if there is any correlation between the
independent variables in the research. As a result, we would delete one of the independent
variables out if there is any correlation between them. Matrix of correlations (1) (2) (3) (4) (5) (6) (7) Variables (1) unemployment rate 1.000 (2) inflation rate - 1.000 0.190 (3) bot - -0.047 1.000 0.063 (4) trade openness 0.101 0.019 0.021 1.000 (5) gdp growth rate 0.004 - 0.042 -0.016 1.000 0.032 (6) exchange rate -0.441 - 0.116 -0.434 -0.022 1.000 0.243 11 (7) gdp
- -0.441 0.154 -0.454 -0.020 0.992 1.000 0.247
Variance inflation factor VIF 1/VIF gdp 72.92 .014 exchange rate 69.306 .014 inflation rate 1.504 .665 trade openness 1.405 .712 1.223 .817 unemployment rate bot 1.151 .869 gdp growth rate 1.006 .994 Mean VIF 21.217 .
After running the VIF test, we can see that the VIF of GDP and Exchange Rate is quite
high. Thus, we need to consider whether there is any multicollinearity in the research. As
can be seen in the table of matrix of correlation, the correlation point for GDP and
Exchange Rate is high (0.992) which is much higher than the 0.8 point required. Because
of that, in the research, a multicollinearity exists between the GDP and Exchange Rate. To
solve the problem, we need to exclude one of those variables and rerun the tests. Linear regression fdi inflow Coef. St. Err. t-value p-value [95% Conf Interval] Sig
unemployment -3.138e+11 2.803e+11 -1.12 .263 -8.644e+11 2.367e+11 rate inflation rate 1.312e+12 8.526e+11 1.54 .125 -3.635e+11 2.986e+12 bot .139 .015 9.10 0 .109 .168 *** trade openness -4.570e+10 2.889e+10 -1.58 .114 -1.025e+11 1.106e+10 gdp growth rate -1.218e+11 3.252e+11 -0.37 .708 -7.607e+11 5.171e+11 exchange rate 7.642e+09 3.175e+08 24.07 0 7.018e+09 8.266e+09 *** Constant 3.170e+10 3.432e+10 0.92 .356 -3.571e+10 9.912e+10 12 Mean dependent var 138364220109.656 368325151633.373 SD dependent var R-squared 0.708 532 Number of obs F-test 211.669 Prob > F 0.000 Akaike crit. (AIC) 29205.420 29235.356 Bayesian crit. (BIC)
*** p<.01, ** p<.05, * p<.1
Variance inflation factor VIF 1/VIF exchange rate 1.879 .532 inflation rate 1.485 .674 trade openness 1.31 .763 unemployment rate 1.216 .823 bot 1.024 .977 gdp growth rate 1.006 .994 Mean VIF 1.32 .
The tables above show the results of the OLS model and multicollinearity tests after the
elimination of GDP variable. From the tables, we can see that the R-squared has reduced
from 73.1% to only 70.8%. This means that the independent variables can explain about
70.8% variance in the dependent variable. However, the result is still reasonable and
acceptable for further research. Besides, the coefficient shows that in addition to
unemployment rate and GDP growth rate, trade openness also has a negative relationship
with fdi inflow which is different from the first OLS test. The p-value also changes, which
illustrates that only BOT and exchange rate are the independent variables that have high
significance to the dependent variable (p-value = 0 < 0.05) while the others variables are
considered to be insignificant (unemployment rate: 0.263, inflation rate: 0.125, trade
openness: 0.114, GDP growth rate: 0.708).
3. Hausman Specification Test
The next test that we are going to conduct is the Hausman specification test to pick out the
most appropriate model for the research. As if the panel data of our research is balanced,
the FE model would be suitable while if it is unbalanced, the RE model would be better. 13
With the Hausman test, we would point out whether FE model or RE model would be best suited for the panel data.
Fixed effects in regression (FE) results: fdi inflow Coef. St. Err. t-value p-value [95% Conf Interval] Sig
unemployment rate -1.017e+12 5.179e+11 -1.96 .05 -2.034e+12 4.847e+08 * inflation rate 1.257e+12 8.761e+11 1.43 .152 -4.641e+11 2.978e+12 bot .135 .015 8.77 0 .104 .165 *** gdp growth rate -1.267e+11 3.230e+11 -0.39 .695 -7.613e+11 5.080e+11 trade openness 9.138e+10 1.365e+11 0.67 .503 -1.767e+11 3.595e+11 exchange rate 3.741e+09 1.844e+09 2.03 .043 1.180e+08 7.364e+09 ** Constant 7.656e+10 8.214e+10 0.93 .352 -8.481e+10 2.379e+11 Mean dependent var
138364220109.656 SD dependent var 368325151633.37 3 R-squared 0.154 Number of obs 532 F-test 15.743 Prob > F 0.000 Akaike crit. (AIC)
29191.101 Bayesian crit. (BIC) 29221.037
*** p<.01, ** p<.05, * p<.1
Random effects in regression (RE) results fdi inflow Coef. St. Err. t-value p-value [95% Conf Interval] Sig
unemployment rate -3.138e+11 2.803e+11 -1.12 .263 -8.631e+11 2.355e+11 inflation rate 1.312e+12 8.526e+11 1.54 .124 -3.596e+11 2.983e+12 bot .139 .015 9.10 0 .109 .168 *** gdp growth rate -1.218e+11 3.252e+11 -0.37 .708 -7.592e+11 5.157e+11 trade openness -4.570e+10 2.889e+10 -1.58 .114 -1.023e+11 1.093e+10 exchange rate 7.642e+09 3.175e+08 24.07 0 7.020e+09 8.265e+09 *** 14 Constant 3.170e+10 3.432e+10 0.92 .356 -3.556e+10 9.897e+10 Mean dependent var 138364220109.656
SD dependent var 368325151633.37 3 Overall r-squared 0.708 Number of obs 532 Chi-square 1270.015 Prob > chi2 0.000 R-squared within 0.142 R-squared between 0.994
*** p<.01, ** p<.05, * p<.1
Hausman specification test results
Hausman (1978) specification test Coef. 4.671 Chi-square test value P-value .457
As can be seen from the result table of the Hausman specification test, we can see that the
p-value is equal to 0.457, which is higher than 0.01. This means that the most suitable
model for us to analyze our data is by using the Random Effects model.
To this point, our research has already pointed out 2 models which we would use to
illustrate our hypotheses (OLS and RE model).
4. Homoscedasticity Test
In this section, firstly, we will conduct homoscedasticity tests with the ordinary least
squares (OLS) model. The homoscedasticity test used in this part is Breusch Pagan/Cook -
Weisberg test. The results of test are demonstrated below:
Null hypothesis H0: the variance is constant
Variables: fitted values of fdi inflow Chi2(1) = 1682.82 Prob > chi2 = 0.0000
Based on the results, we can see that the p-value is less than 0.05. Therefore, there is
homoscedasticity in the OLS model. After evaluating the homoscedasticity on the OLS
model, the test will be conducted with the random effect model: 15
Null hypothesis H0: sigma(i)^2 = sigma^2 for all i Chi2(7) = 6.1e+05 Prob > chi2 = 0.0000
As can be seen, the p-value is below 0.05, thus, the random effect model has homoscedasticity.
5. Autocorrelation Test
For this section, the autocorrelation test will be performed. Using the Wooldridge test for
autocorrelation in panel data, the results can be observed as follows:
Null hypothesis H0: no first-order autocorrelation F(1, 6) = 66.080 Prob > F = 0.0002
Obviously, the p-value, which is 0.0002, is lower than 0.05. Hence, the random effect
model has an autocorrelation phenomenon.
Taking everything into consideration, both OLS and random effect models experience
heteroscedasticity; additionally, autocorrelation condition for random effect models. After
adjusting the OLS model, we get the result table as follows:
Cross-sectional time-series FGLS regression fdi inflow Coef. St. Err. t-value p-value [95% Conf Interval] Sig unemployment rate 0 . . . . . inflation rate 0 . . . . . bot -.075 .021 -3.53 0 -.116 -.033 *** trade openness 0 . . . . . gdp growth rate 0 . . . . . exchange rate 8.159e+09 5.427e+08 15.04 0 7.096e+09 9.223e+09 *** Constant 53447226 1.287e+09 0.04 .967 -2.469e+09 2.575e+09 Mean dependent var
138364220109.656 SD dependent var 368325151633.373 Number of obs 532 Chi-square 234.566
*** p<.01, ** p<.05, * p<.1 16
In conclusion, only two independent variables of balance of trade and exchange rate, whose
p-values are both 0, are significant to FDI inflows. In that, balance of trade shows a
negative relationship with FDI inflows, while exchange rate demonstrates a positive
relationship with FDI inflows. V. DISCUSSION
For H1, when conducting the VIF test, the researchers discovered that the result of GDP
and exchange rate appeared to be multicollinear; thus, we decided to discontinue a GDP
variable to eliminate this problem and conclude that this GDP variable may have an
insignificant effect on the FDI inflows of G7 countries. In terms of H2, the results reveal
that the variable GDP growth rate has little effect on FDI inflows into G7 nations. These
findings indicate an insignificant coefficient and a significant difference between the
dependent and independent variables. That demonstrates the G7 countries' insignificance
in attracting FDI inflows based on GDP or GDP growth rates. In reality, the influence of
FDI on GDP growth rate is non-linear (Zadeh and Madani, 2012); therefore, only focusing
on the impact of GDP growth rate cannot bring a clear observation of the FDI decision
from foreign investors. The G7 countries should investigate further factors apart from these
GDP and GDP growth rate variables.
According to the regression analysis, it is clearly stated that the unemployment rate has a
negative relationship with FDI inflows, which is not in agreement with hypothesis 3. The
result is consistent with some empirical research (Gupta and Singh (2016), Chang (2007),
Ozughalu and Ogwumike (2013)). Nevertheless, several studies found that FDI inflows
and unemployment rate have a positive relationship. (Ciftcioglu et al. (2007), Hisarciklilar
et al. (2013), Strat et al. (2015)). Interestingly, even some researchers concluded that FDI
inflows and unemployment rate have no relationship. (Aktar and Ozturk, 2009). Obviously,
we can say that each empirical research might have a different group of subjects for study,
which lead to different results. According to Strat et al. (2015), increased unemployment
contributes to improved inflows of foreign direct investment, demonstrating that
international investors seek places where labor availability is not a concern. However, to
some extent, the positive relationship between them could change reversely if the
unemployment rate was considered high since overseas investors would not be motivated
in locating a prospective investment or expanding a current one in a nation where
macroeconomic instability is visible. Theoretically, the acceptable natural unemployment
rate for a country is in the range of
4% to 5% (Investopedia. Kagan, 2021). This is partially valid for the study results since we
study G7 nations, where some members of this forum have quite a high unemployment rate,
belonging to the three European countries (from 8% to 9% on average).
According to the OLS test, the balance of trade has a positive influence on inward FDIs,
which contradicts the previously proposed hypothesis. However, this result is supported by 17
Chotia (2015). Trade openness is found to not have any significant impact on the FDI
inflows, which is inconsistent with hypothesis 5. This result is not in agreement with
several previous studies (Shah & Samdani, 2017; Shah & Khan, 2016; Panagiotis &
Konstantinos, 2012). However, those previous studies were aimed at developing countries.
So, the inconsistency in the results could be from the difference in the context of the
research as different areas may be affected by different aspects.
Inflation rate is found to have a positive relationship with FDI inflow, which is inconsistent
with hypothesis 6. This result is also not in agreement with some previousmentioned
studies (Cevis & Çamurdan, 2007; Rodriguez & Pallas, 2008; Demirhan & Masca, 2008).
However, this result is consistent with the findings of more recent research (Singhania,
2011; Boateng, 2015). The year-on-year inflation rate in the G7 countries is on average
stable and under 10% so it can be a good explanation for the positive relationship with FDI.
A low inflation rate can reflect economic stability, thus encouraging foreign investors and
increasing FDI inflows (Coskun, 2001). The result of the exchange rate is in alignment
with hypothesis 7 which proposes a positive relationship with inward FDIs. As exchange
rate ensures the use and importance of money’s flow and value, it can act as an incentive
for foreign investors to invest in a country (Singhania, 2011).
This research investigates a quarterly data set of macroeconomic and policy variables to
figure out their influence on FDI inflows into G7 countries from 2001 to 2021. Our
empirical results indicate that FDI inflows have positive relationships with the balance of
trade, inflation rate and exchange rate; insignificant relationships with GDP, GDP growth;
no relationship with the unemployment rate and trade openness. It seems that
macroeconomic policy tends to have more impact on FDI inflows into G7 countries during
the study period than market-related and resource-related economic variables. VI. CONCLUSION
This research investigates a quarterly data set of macroeconomic and policy variables to
figure out their influence on FDI inflows into G7 countries from 2002 to 2021. Our
empirical results indicate that FDI inflows have positive relationships with the balance of
trade, inflation rate and exchange rate; insignificant relationships with GDP, GDP growth;
no relationship with the unemployment rate and trade openness. It seems that
macroeconomic policy tends to have more impact on FDI inflows into G7 countries during
the study period than market-related and resource-related economic variables. This result
can be due to the lack of other potential variables from each category; therefore, further
research should take into account more representative determinants on FDI inflows into
developed countries to have a less biased finding. 18 REFERENCE
• Ahn Y.S, Adji S.S and Willett T.D (1998).The Effects of Inflation Exchange rate
policies on Direct Investment to Developing Countries. International Economic
Journal.12 (1), page 95 – 104.
• Aziz, O. G., & Mishra, A. V. (2015). Determinants of FDI inflows to Arab
economies. The Journal of International Trade & Economic Development, 25(3), 325 – 356.
• Asiedu, E., & Lien, D. (2004). Capital Controls and Foreign Direct Investment.
World Development, 32(3), 479 – 490.
• Asiedu, E. (2002). On the determinants of foreign direct investment to developing
countries: If Africa different? World Development, 30, 107 – 119.
• Bagli, S. and Adhikary, M. (2014), “FDI inflow and economic growth in India an
empirical analysis”, Economic Affairs, Vol. 59 No. 1, pp. 23 – 33.
• Blanchard, O. (2011). Macroeconomics, Boston, Mass., Pearson Prentice Hall.
• Botric, V. & Škuflic, L. (2006). Main Determinants of Foreign Direct Investment in
the Southeast European Countries. Transition Studies Review, 13, 359 – 377.
• Brozen, Y. (1958). Means for Maintaining Economic Stability. Journal of Farm Economics, 40, 1069 – 1078.
• Biglaiser, G., & DeRouen, K. (2006). Economic reforms and inflows of foreign
direct investment in Latin America. Latin American Research Review, 41(1), 51 – 75.
• Boateng, A., Hua, X., Nisar, S., & Wu, J. (2015). Examining the determinants of
inward FDI: Evidence from Norway. Economic Modelling, 47, 118 – 127.
• Buchanan, A.E. (2011), Beyond Humanity? The Ethics of Biomedical Enhancement, Oxford University Press.
• Buckley, P.J., Clegg, L.J., Cross, A.R., Liu, X., Voss, H. and Zheng, P. (2007), “The
determinants of Chinese outward foreign direct investment”, Journal of
International Business Studies, Vol. 38 No. 4, pp. 499 – 518.
• Balasubramanyam, V.N. (2002), “Foreign direct investment in developing
countries: determinants and impact”, New Horizons for Foreign Direct Investment, No. 548, pp. 187 – 208.
• Barrel R and Pain N. (1996). An Econometric Analysis of U.S Foreign Direct
Investment. The Review of Economics and Statistics, 78(2), page 200 – 207.
• Blonigen B.A (1997). Frim-Specific Assets and Link between Exchange rates and
Foreign Direct Investment. The Amercan Economic Review, 87(3), page 447 – 465.
• Caves, R.E., 1989. Mergers, takeover and economic efficiency. Int. J. Ind. Organ. 7, 151 – 175.
• Chidlow, A., Salciuviene, L., Young, S., 2009. Regional determinants of inward FDI
distribution in Poland. Int. Bus. Rev. 18 (2), 119 – 133. 19
• Chakrabarti, A. (2001). The determinants of foreign direct investment: Sensitivity
analyses of cross-country regressions. KYKLOS, 54, 89 – 112.
• Cevis, İ. and Çamurdan, B. (2007), “The economic determinants of foreign direct
investment in developing countries and transition economies”, The Pakistan
Development Review, Vol. 46 No. 3, pp. 285 – 299.
• Demirhan, E. and Masca, M. (2008), “Determinants of foreign direct investment
flows to developing countries: a cross-sectional analysis”, Prague Economic Papers,
Vol. 2008 No. 4, pp. 356 – 369.
• Froot, K., Stein, J.C., 1991. Exchange rates and foreign direct investment: an
imperfect capital markets approach. Q. J. Econ. 106, 1191 – 1217.
• Friedman, J., Gerlowski, D.A., Silberman, J., 1992. What attracts foreign multinational
• corporations? Evidence from branch plan location in the United States. J. Reg. Sci. 32 (4), 403 – 418.
• Helleiner, G. 2002. Trade Policy and Industrialization in Turbulent Times. London: Routledge.
• Kish, R.J., Vasconcellos, G.M., 1993. An empirical analysis of factors affecting
cross-border acquisitions: US–Japan. Manag. Int. Rev. 33 (3), 227 – 245.
• Morisset, P. 2000. Foreign Direct Investment to Africa: Policies Also Matter.
Transnational Corporation 9: 107 – 125.
• Na, L. and Lightfoot, W.S. (2006). Determinants of foreign direct investment at the
regional level in China”. Journal of Technology Management in China, Vol. 1 No. 3, pp. 262 – 278.
• Nunnenkamp, P., Schweickert, R., Wiebelt, M., 2007. Distributional effects of FDI:
how the interaction of FDI and economic policy affects poor households in Bolivia.
Dev. Policy Rev. 25, 429 – 450.
• Noorbakhsh, F., Paloni, A., & Youssef, A. (2001). Human Capital and FDI Inflows
to Developing Countries: New Empirical Evidence. World Development, 29(9), 1593 – 1610.
• Nishimizu, M., and J. Page. 1991. Trade Policy, Market Orientation and
Productivity Change in Industry, in Trade Theory and Economic Reform: Essays in
honor of Bela Balassa, edited by Jame De Melo and Andre Sapir. Cambridge, MA: Brasil Blackwell.
• Nishimizu, M., and S. Robinson. 1986. Productivity growth in manufacturing, in
Industrialization and Growth: A Comparative Study, edited by Hollis B. Chenery,
Sherman Robinson and Moshe Syrquin. New York: Oxford University Press, pp 283 – 308.
• Quazi, R.M. 2007. Investment Climate and Foreign Direct Investment: A Study of
Selected Countries in Latin America. Global Journal of Business Research, 1(1), 1 – 13. 20