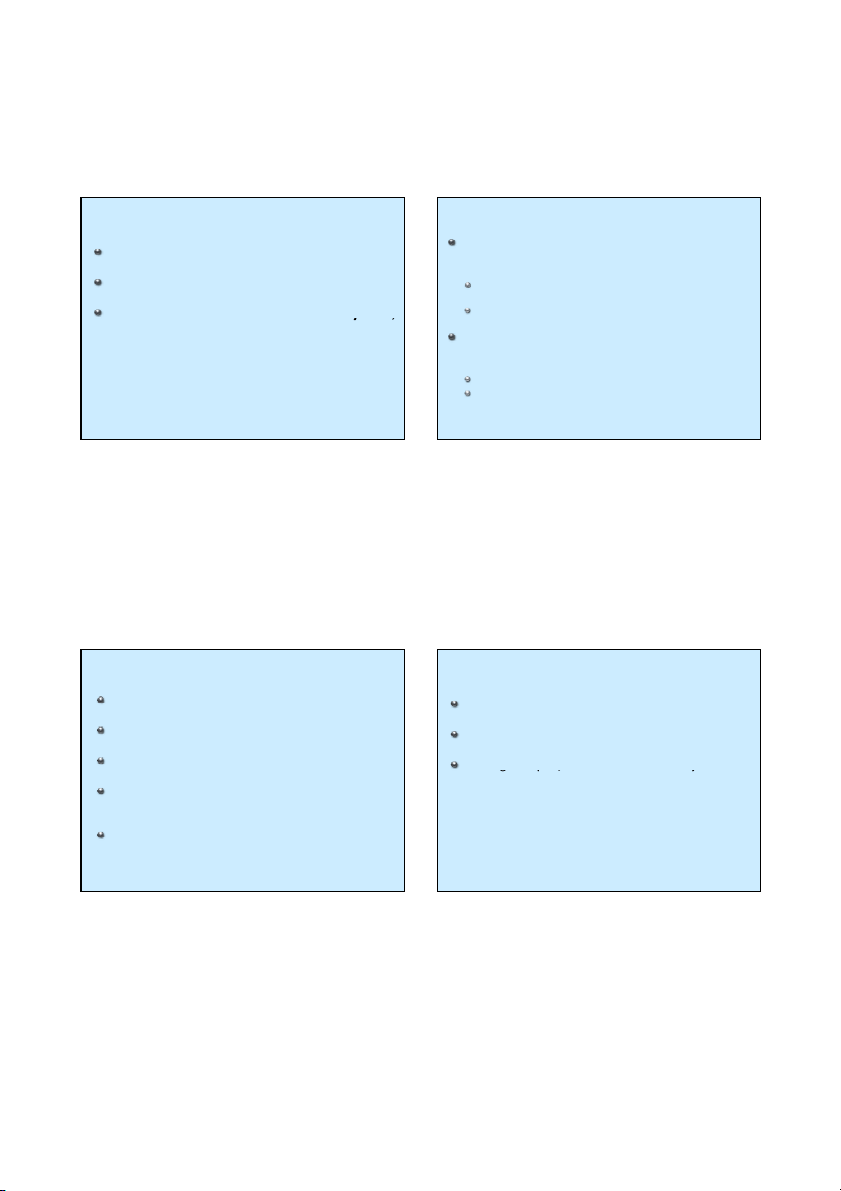
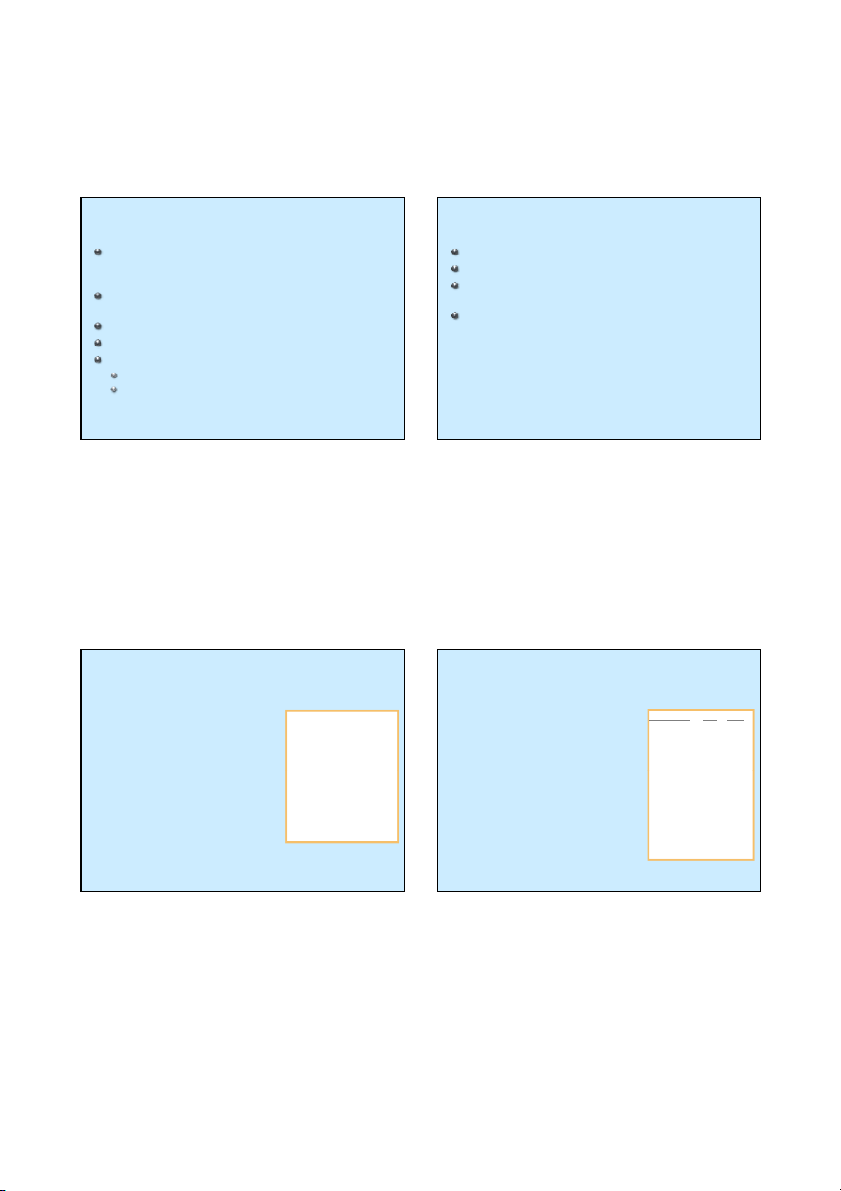
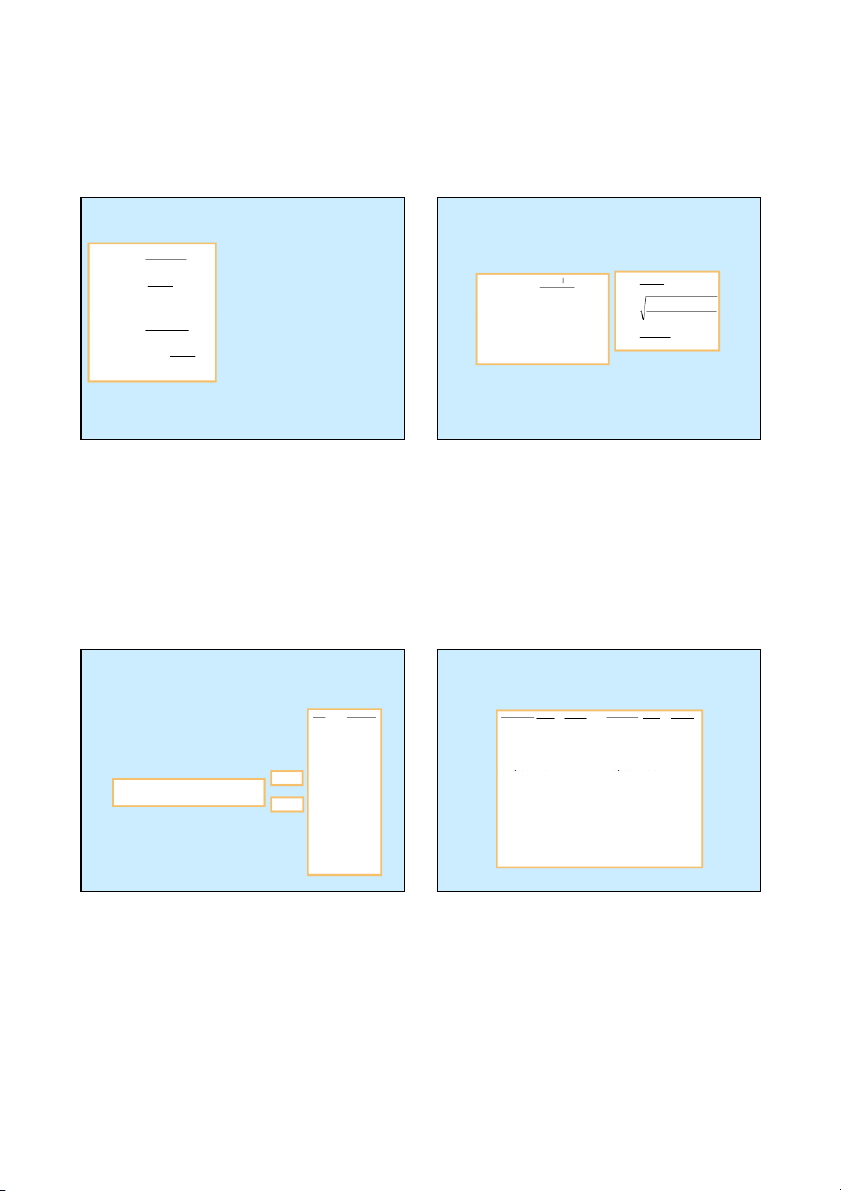
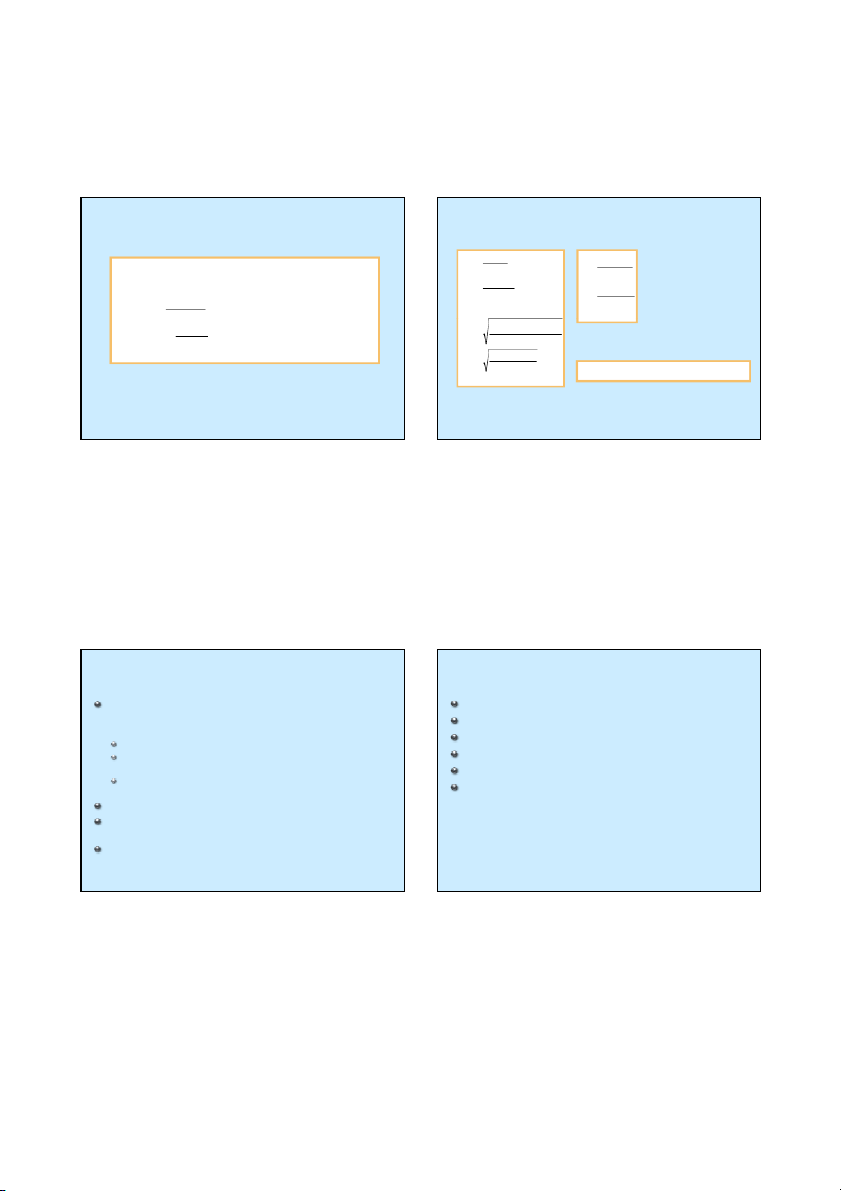
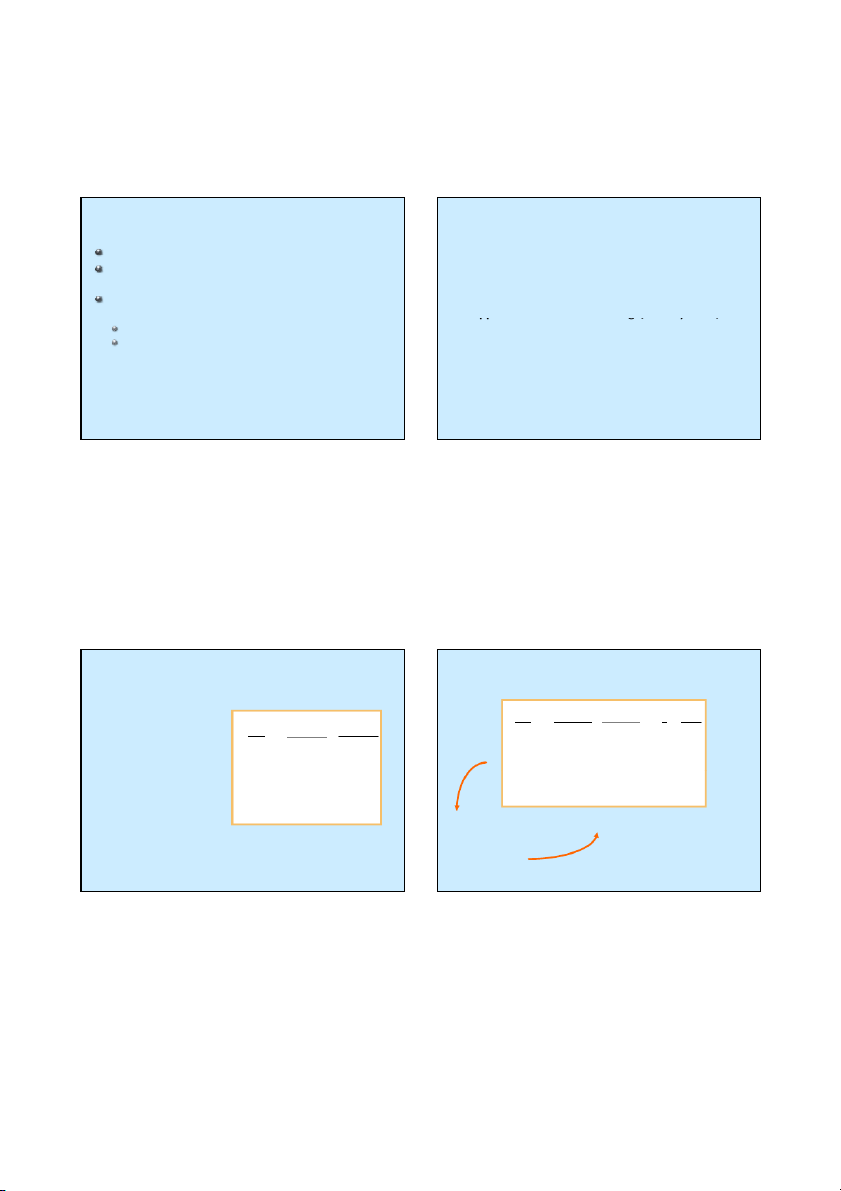
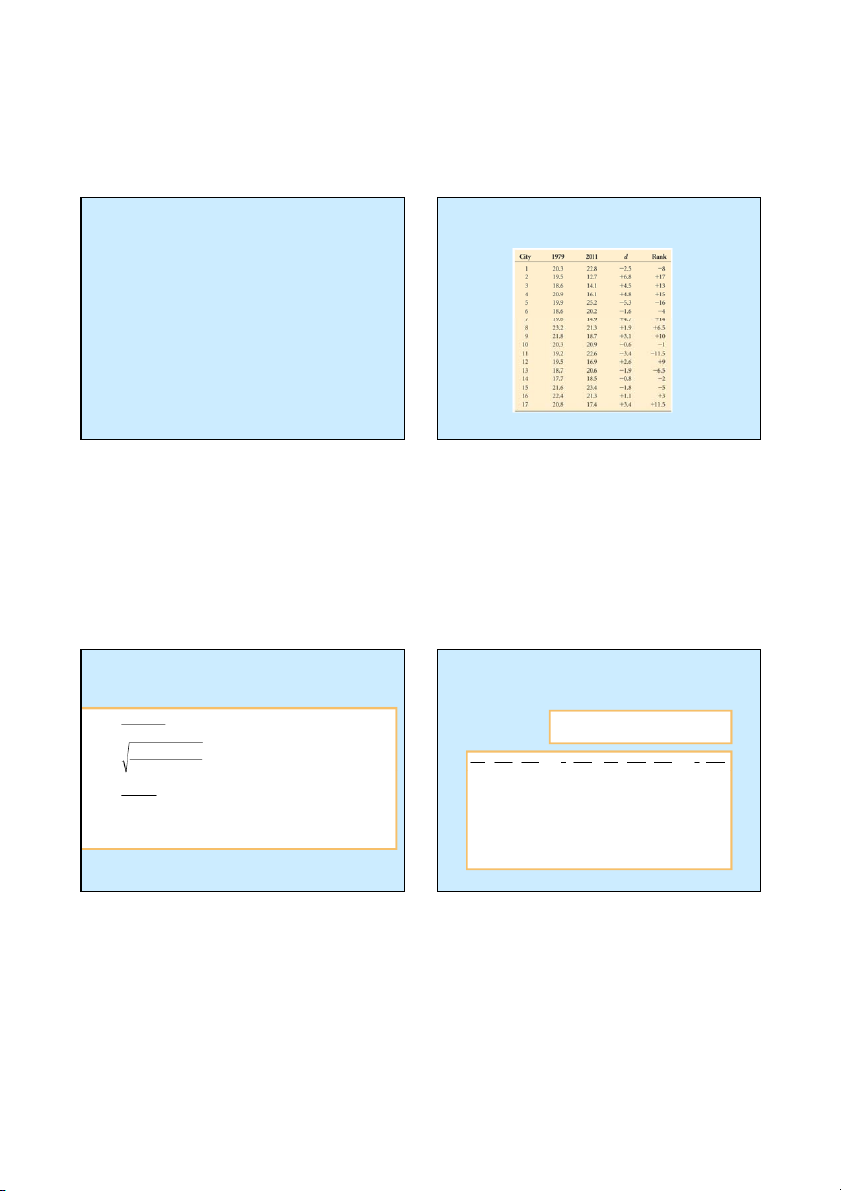
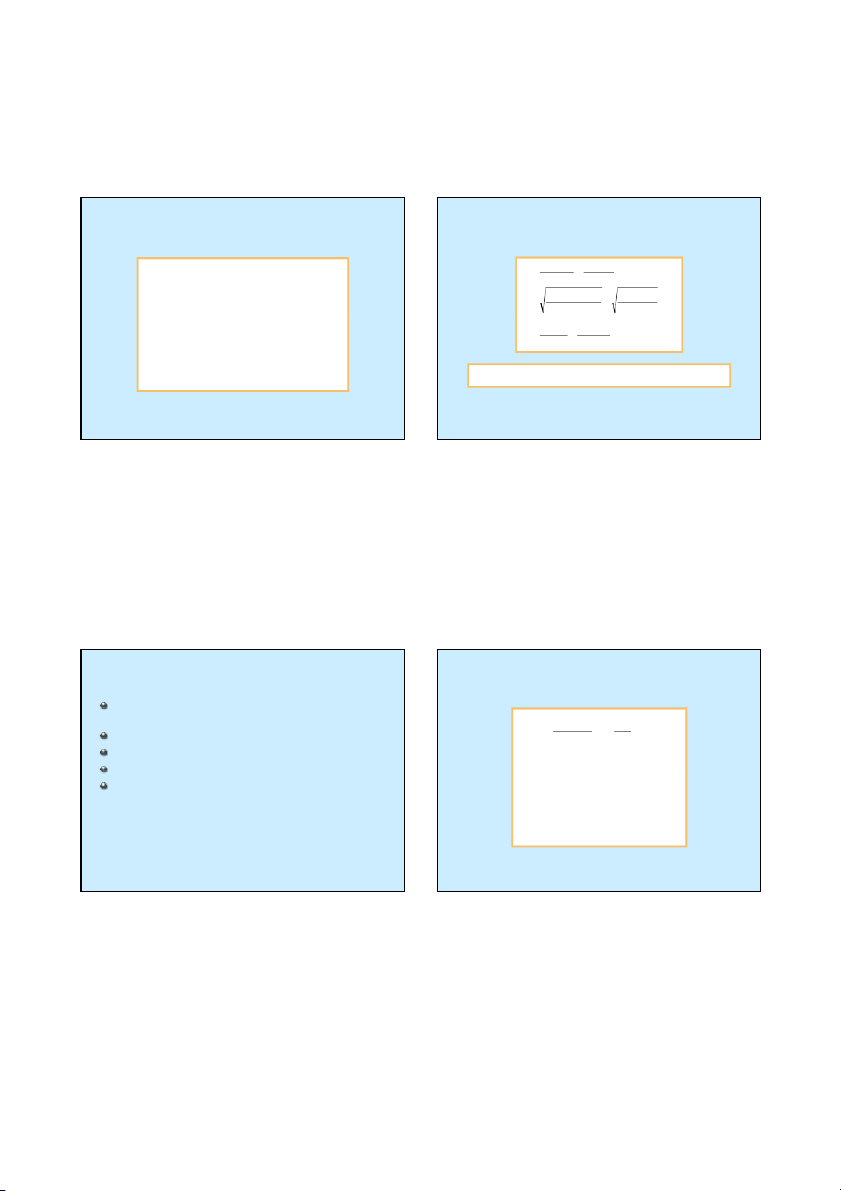
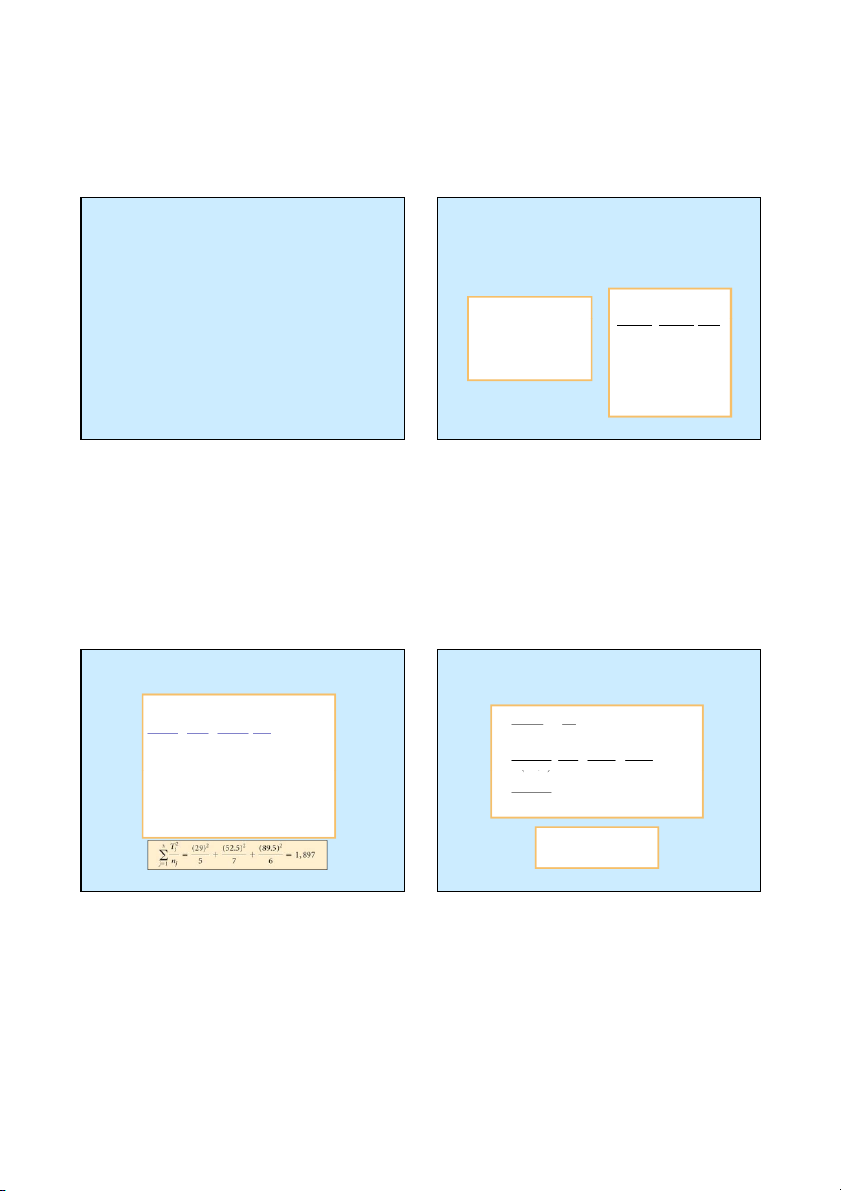
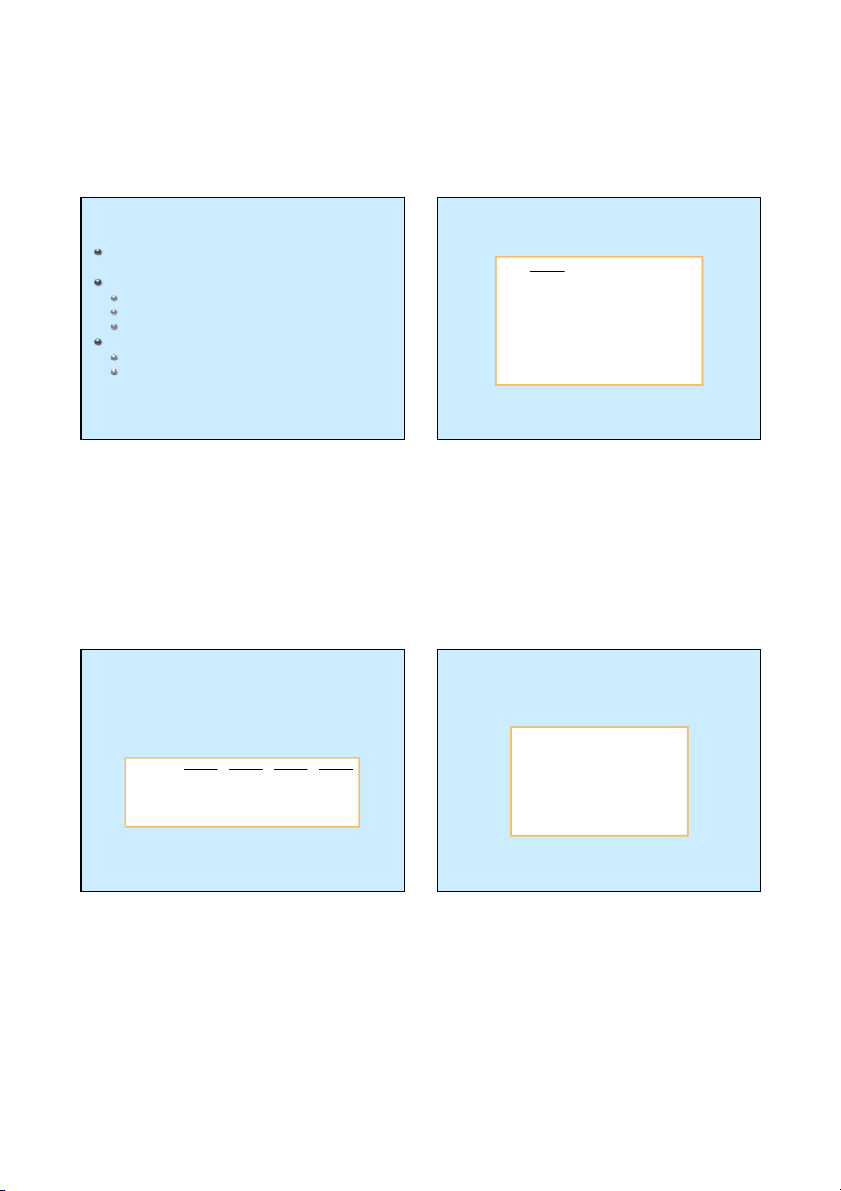
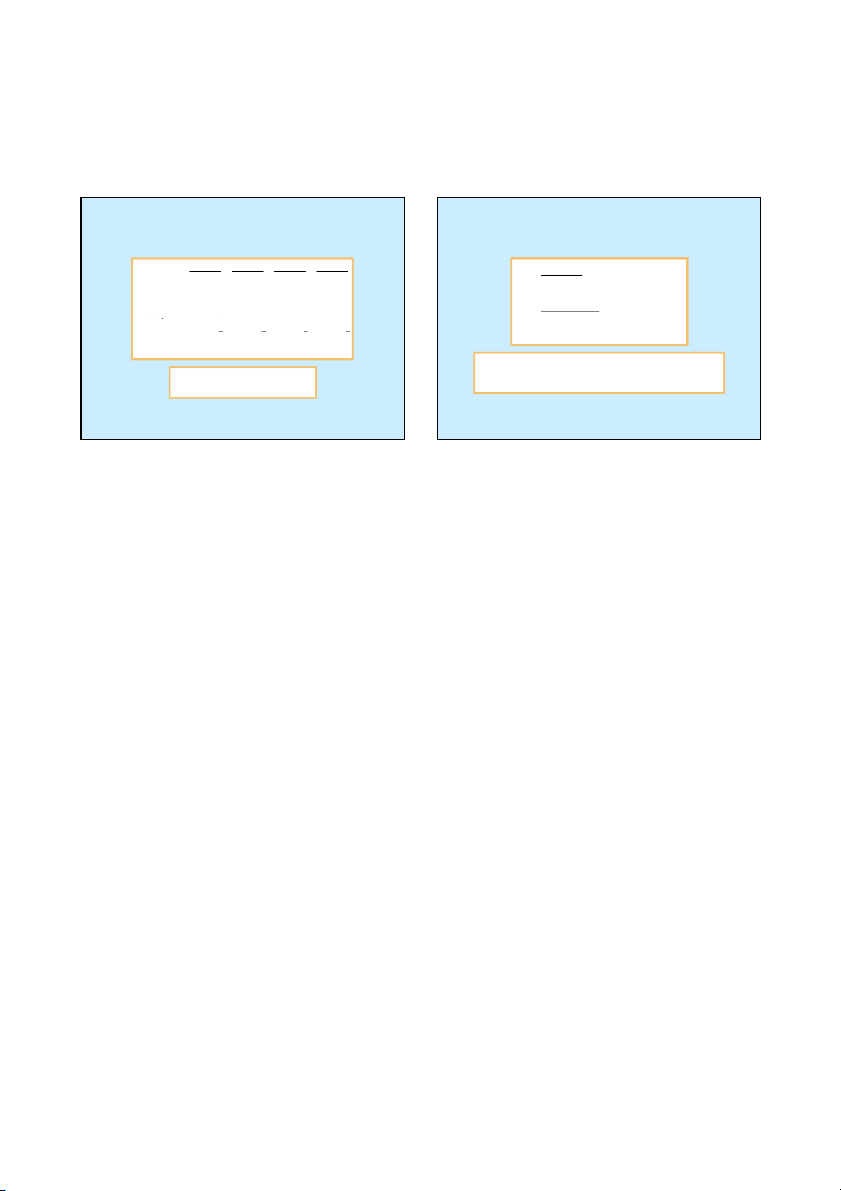
Preview text:
LearningObjectives
Parametricvs.NonparametricStatistics
ParametricStatisticsarestatisticaltechniquesbased
Recognizetheadvantagesanddisadvantagesof
onassumptionsaboutthepopulationfromwhichthe
nonparametricstatistics.
sampledataarecollected.
Understandhowtousetherunstesttotestfor
Assumptionthatdatabeinganalyzedarerandomly randomness.
selectedfromanormallydistributedpopulation.
KnowwhenandhowtousetheMann‐WhitneyU test,
Requiresquantitativemeasurementthatyieldinterval
theWilcoxonmatched‐pairssignedranktest,the
orratioleveldata.
Kruskal‐Wallistest,andtheFriedmantest.
NonparametricStatisticsarebasedonfewer
assumptionsaboutthepopulationandthe parameters.
Sometimescalled“distribution‐free”statistics.
Avarietyofnonparametricstatisticsareavailablefor
usewithnominalorordinaldata.
AdvantagesofNonparametricTechniques
DisadvantagesofNonparametricStatistics
Sometimesthereisnoparametricalternativetothe
Nonparametrictestscanbewastefulofdataif
useofnonparametricstatistics.
parametrictestsareavailableforusewiththedata.
Certainnonparametrictestcanbeusedtoanalyze
Nonparametrictestsareusuallynotaswidely nominaldata.
availableandwellknowasparametrictests.
Certainnonparametrictestcanbeusedtoanalyze
Forlargesamples,thecalculationsfo r many ordinaldata.
nonparametricstatisticscanbetedious.
Thecomputationsonnonparametricstatisticsare
usuallylesscomplicatedthanthoseforparametric
statistics,particularlyforsmallsamples.
Probabilitystatementsobtainedfrommost
nonparametrictestsareexactprobabilities.
Mann‐WhitneyU Test
Mann‐WhitneyU Test:
SampleSizeConsideration
Mann‐WhitneyUtest‐ anonparametriccounterpart
Sizeofsampleone:n1
ofthe ttestusedtocomparethemeansoftwo
Sizeofsampletwo:n independent 2 populations.
Ifbothn and are 1 n2
10,thesmallsampleprocedure
Nonparametriccounterpartofthet testfor isappropriate. independentsamples If either
eithe n or
n o n is greater than 10 the large sample 1
n2 greaterthan10,thelargesampl
Doesnotrequirenormallydistributedpopulations
procedureisappropriate.
Maybeappliedtoordinaldata Assumptions IndependentSamples
AtLeastOrdinalData
Mann‐WhitneyUTest:SmallSample
Mann‐WhitneyUTest:SmallSample Example‐Demonstration Example‐Demonstration H : = 0 µ1=µ2 .05 Compensation Rank Group H : π Drug 18.75 1 H a A DrugB 19.80 2 H 20.10 26.19
Ifthefinalp‐value<.05,rejectH . 0 20.10 3 H 19.80 23.88 20.75 4 H 21.64 5 E 22.36 25.50 21.90 6 H 18.75 21.64
W =1+2+3+4+6 + 7 + 8 = 31 1 22.36 7 H 22.96 8 H 21.90 24.85 23.45 9 E
W =5+9+10+11+12+13+14+15= 22.96 25.30 2 89 23.88 10 E 24.12 11 E 20.75 24.12 24.85 12 E 23.45 25.30 13 E 25.50 14 E 26.19 15 E
Mann‐WhitneyU Test:Small
Mann‐WhitneyU Test: SampleExample
FormulasforLargeSampleCase
SinceU <U ,U =3. 2 1
p‐value=.0011*2 (for
atwo‐tailedtest)=.022
<.05,rejectH . 0
Example– MannWhitneyUforlarge
RanksofIncomefromCombined samples
GroupsofPBSandNon‐PBSViewers PBS Non‐PBS Datavalue Rank Group Datavalue Rank Group 24,500 41,000 π 16,000 1 Non‐PBS 39,500 15 Non‐PBS 39,400 32,500 21,000 2 Non‐PBS 40,500 16 Non‐PBS 36,800 33,000 21,500 3 Non‐PBS 41,000 17 Non‐PBS 44,300 21,000 24,500 4 PBS 43,000 18 PBS 57 5 9 ,9 6 6 0 0 40 4 , 5 5 0 0 0 0 27,600 5 Non‐PBS 43,500 19.5 PBS n = 1 14 32,000 32,400 27,800 6 Non ‐PBS 43,500 19.5 Non‐PBS 61,000 16,000 32,000 7 PBS 51,900 21 Non‐PBS 34,000 21,500 32,400 8 Non‐PBS 53,000 22 PBS n = 2 13 43,500 39,500 32,500 9 Non‐PBS 55,000 23 PBS 33,000 10 Non‐PBS 57,960 24 PBS 55,000 27,600 34,000 11 PBS 61,000 25 PBS 39,000 43,500 36,800 12 PBS 61,400 26 PBS 62,500 51,900 39,000 13 PBS 62,500 27 PBS 61,400 27,800 39,400 14 PBS 53,000
PBSandNon‐PBS:CalculationofU
PBSandNon‐PBS:Conclusion
WilcoxonMatched‐PairsSignedRankTest
WilcoxonMatched‐PairsSignedRankTest
Differencesofthescoresofthetwomatchedsamples
Differencesareranked,ignoringthesign
Ranksaregiventhesignofthedifference
Positiveranksaresummed Negative v ranks are summed ranksaresumme
T isthesmallersumofranks
WilcoxonMatched‐PairsSignedRankTest:
WilcoxonMatched‐PairsSignedRankTest:
SampleSizeConsideration
SmallSampleExample
n isthenumberofmatchedpairs
Considerthesurveyb
y AmericanDemographicsthat
Ifn >15,T isapproximatelynormallydistributed,
estimatedtheaverageannualhouseholdspending
andaZ testisused.
onhealthcare.TheU.S.metropolitanaveragewas
Ifn 15,aspecial“smallsample”procedureis $1,800. followed.
SupposesixfamiliesinPittsburgh ,Pennsylvania,are
Thepaireddataarerandomlyselected.
matcheddemographicallywithsixfamiliesin
Theunderlyingdistributionsaresymmetric.
Oakland,California,andtheiramountso fhousehold
spendingonhealthcareforlastyearareobtained.
WilcoxonMatched‐PairsSignedRankTest:
WilcoxonMatched‐PairsSignedRankTest:
SmallSampleExample
SmallSampleExample Family H : =0 0 Md Pair SampleA SampleB d Rank H :M 0 a d Pair SampleA SampleB 1 1,950 1,760 190 +4 1 1,950 1,760 2 1,840 1,870 ‐30 ‐1 n=6 2 1,840 1,870 3 2,015 1,810 205 +5 3 2,015 1,810 4 1 5 , 8 5 0 8 1 6 , 6 6 0 6 8 ‐ 0 2 ‐ =0.05 4 1,580 1,660 5 1,790 1,340 450 +6 6 1,925 1,765 160 +3 5 1,790 1,340 IfT 1,rejectH 6 1,925 1,765 observed 0.
WilcoxonMatched‐PairsSignedRankTest:
WilcoxonMatched‐PairsSignedRankTest:
LargeSampleFormulas
LargeSampleFormulas
Forlargesamples,theTstatisticisapproximatelynormally
distributedandazscoreca n b
e usedastheteststatistic.
WilcoxonMatched‐PairsSignedRankTest: Example
LargeSampleFormulas City 1979 2011 d Rank City 1979 2011 d Rank 1 20 2 3 . 22 2 .8 8 2 ‐ .5 ‐ 8 10 1 20 2 .3 3 20 2 .9 9 ‐ 0.6 1 ‐ 2 19.5 12.7 6.8 17 11 19.2 22.6 ‐3.4 ‐11.5 3 18.6 14.1 4.5 13 12 19.5 16.9 2.6 9 4 20.9 16.1 4.8 15 13 18.7 20.6 ‐1.9 ‐6.5 5 19.9 25.2 ‐5.3 ‐16 14 17.7 18.5 ‐0.8 ‐2 6 18.6 20.2 ‐1.6 ‐4 15 21.6 23.4 ‐1.8 ‐5 7 19.6 14.9 4.7 14 16 22.4 21.3 1.1 3 8 23.2 21.3 1.9 6.5 17 20.8 17.4 3.4 11.5 9 21.8 18.7 3.1 10
T Calculation Conclusion
Kruskal‐WallisTest
Kruskal‐WallisK Statistic
Kruskal‐WallisTest‐ Anonparametricalternative
toone‐wayanalysisofvariance
Mayusedtoanalyzeordinaldata
Noassumedpopulationshape As A s s u s m u e m s e that the
that th C groups are independent
C groupsareindependen
Assumesrandomselectionofindividualitems
NumberofPatientsperDayperPhysician
NumberofPatientsperDayperPhysician
inThreeOrganizationalCategories
inThreeOrganizationalCategories
Supposearesearcherwantstodeterminewhetherthe H :
o Thethreepopulationsareidentical
numberofphysiciansinanofficeproducessignificant H :
a Atleastoneofthethreepopulationsisdifferent
differencesinthenumberofoffic e patientssee n b y eac h Threeor
physicianperday. Two More Sh S et k a esara d n omsam l p e f o phy i s i
c ansfrompractices Partners P artners HMO
inwhich(1)thereareonlytwopartners,(2)thereare 13 24 26 three 15 16 22
ormorepartners,or(3)theofficeisahealth 20 19 31
maintenanceorganization(HMO). 18 22 27 23 25 28 14 33 17
PatientsperDayData:Kruskal‐Wallis
PatientsperDayData:Kruskal‐Wallis
PreliminaryCalculations
CalculationsandConclusion Threeor Two More Partners Partners HMO Patients Rank
Patients Rank Patients Rank 13 1 24 12 26 14 15 3 16 4 22 9.5 20 8 19 7 31 17 18 6 22 9 5 . 27 15 23 11 25 13 28 16 14 2 33 18 17 5 T =29 T = = 1 2 52.5 T3 89.5 n = = = 1 5 n2 7 n3 6 n=n + + = + + = 1
n2 n3 5 7 6 18 FriedmanTest FriedmanTest
FriedmanTest‐ Anonparametricalternativetothe
randomizedblockdesign Assumptions
Theblocksareindependent. There
isnointeractionbetweenblocksandtreatments.
Observationswithineachblockcanberanked. Hypotheses H :
o Thetreatmentpopulationsareequal H :
a Atleastonetreatmentpopulationyieldslargervalues
thanatleastoneothertreatmentpopulation FriedmanTest: FriedmanTest:
H : The supplier equal o populationsare
H : At least one supplier s larger a
populationyield values
thanatleastoneothersupplierpopulation Supplier1 Supplier2 Supplier3 Supplier4 Monday 62 63 57 61 Tuesday 63 61 59 65 Wednesday 61 62 56 63 Thursday 62 60 57 64 Friday 64 63 58 66 FriedmanTest: FriedmanTest: Supplier1 Supplier2 Supplier3 Supplier4 Monday 3 4 1 2 Tuesday 3 2 1 4 Wednesday 2 3 1 4 Thursday 3 2 1 4 Friday 3 2 1 4 R j 14 13 5 18 R2 196 169 25 324 j