-
Thông tin
-
Quiz
Đồ thị từ Ma trận - Toán Kinh Tế | Trường Đại học Tôn Đức Thắng
Ôn tập lý thuyết đồ thị từ Ma trận học phần Toán Kinh Tế - Toán Kinh Tế | Trường Đại học Tôn Đức Thắng. Tài liệu được sưu tầm và soạn thảo dưới dạng file PDF để gửi tới các bạn sinh viên cùng tham khảo, ôn tập đầy đủ kiến thức, chuẩn bị cho các buổi học thật tốt và đạt kết quả cao trong môn học. Mời bạn đọc đón xem!
Toán Kinh Tế (C01120) 73 tài liệu
Đại học Tôn Đức Thắng 3.5 K tài liệu
Đồ thị từ Ma trận - Toán Kinh Tế | Trường Đại học Tôn Đức Thắng
Ôn tập lý thuyết đồ thị từ Ma trận học phần Toán Kinh Tế - Toán Kinh Tế | Trường Đại học Tôn Đức Thắng. Tài liệu được sưu tầm và soạn thảo dưới dạng file PDF để gửi tới các bạn sinh viên cùng tham khảo, ôn tập đầy đủ kiến thức, chuẩn bị cho các buổi học thật tốt và đạt kết quả cao trong môn học. Mời bạn đọc đón xem!
Môn: Toán Kinh Tế (C01120) 73 tài liệu
Trường: Đại học Tôn Đức Thắng 3.5 K tài liệu
Thông tin:
Tác giả:
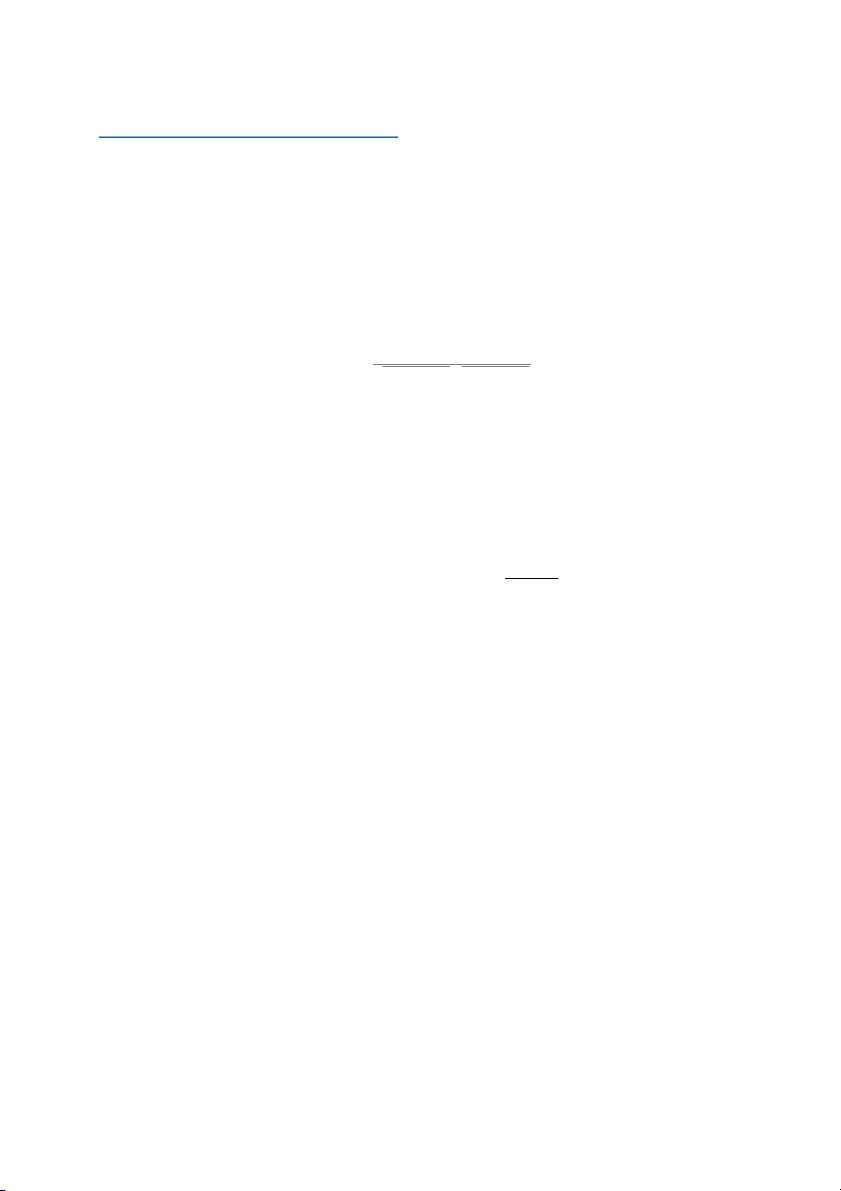
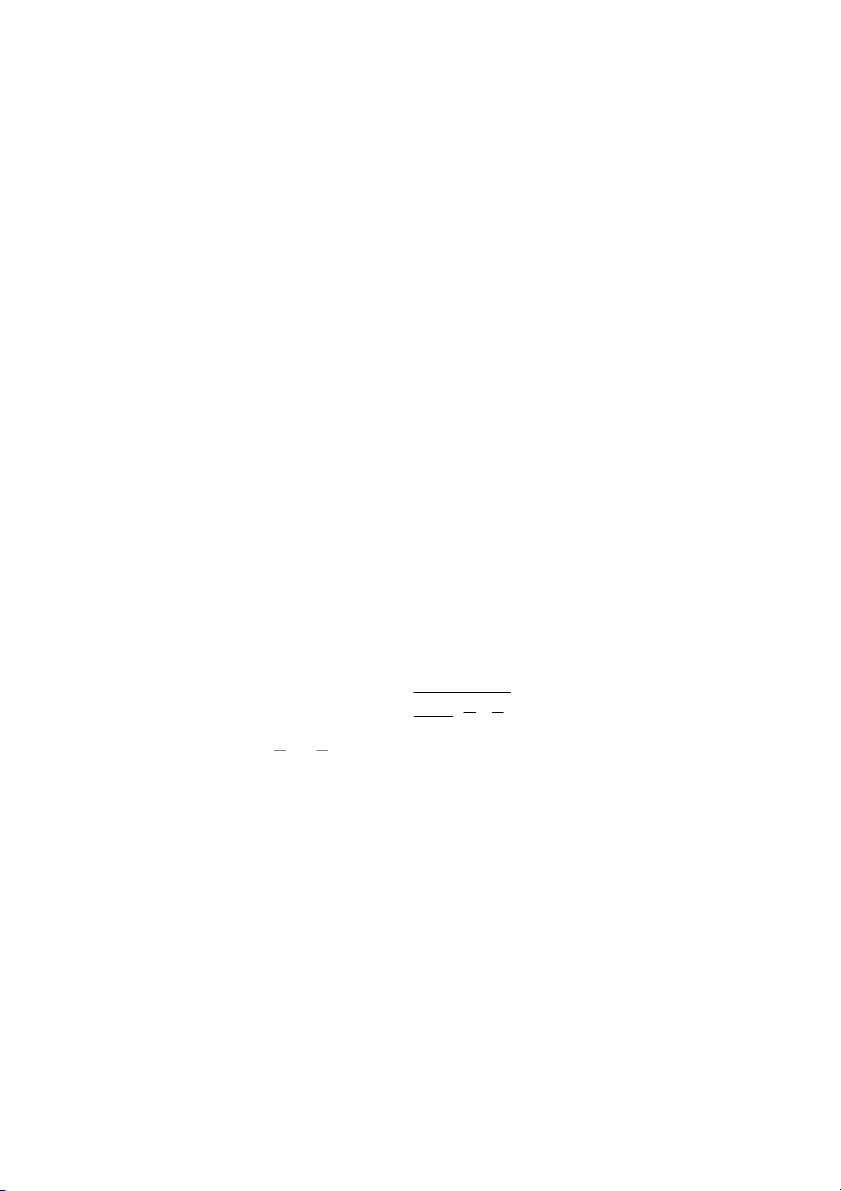
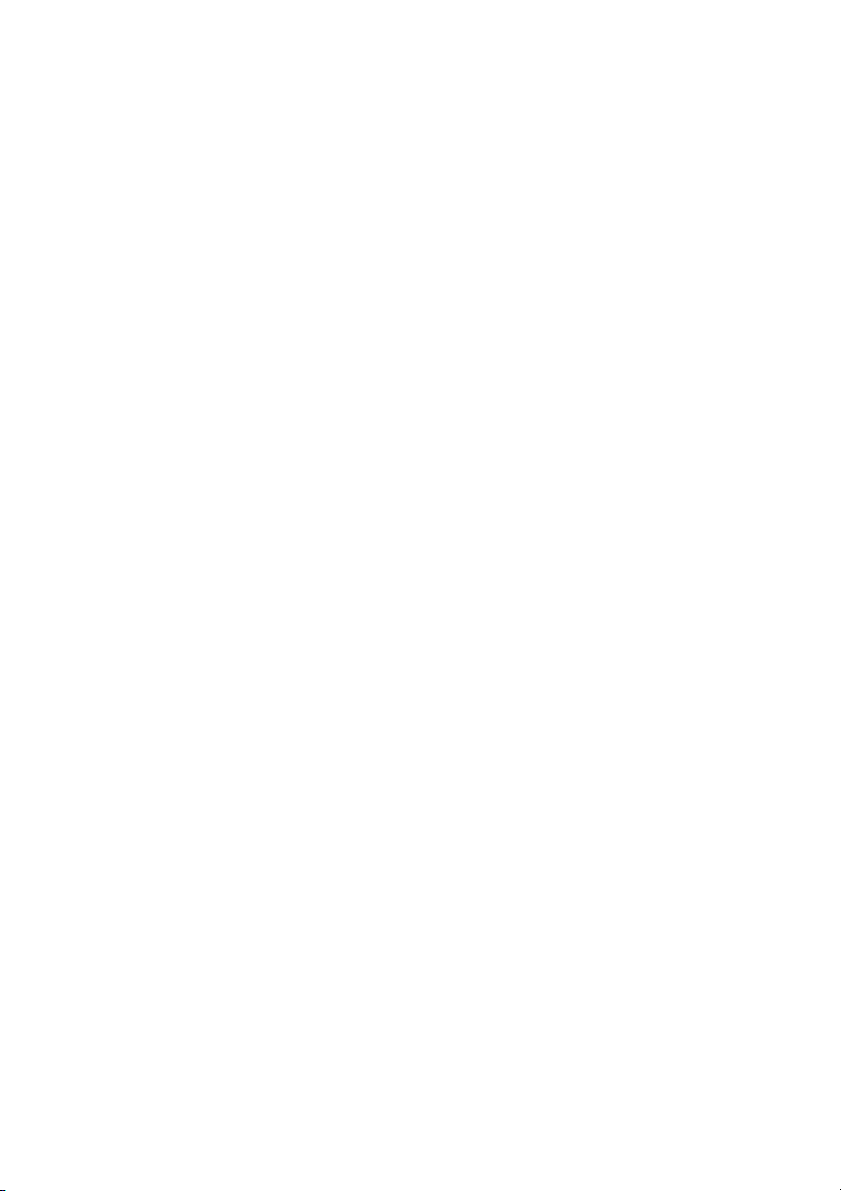
Tài liệu khác của Đại học Tôn Đức Thắng
Preview text:
Đồ thị từ Ma trận
https://arxiv.org/pdf/cond-mat/0009350.pdf
Chúng ta sẽ xem xét đồ thị G=V,E, bao gồm các nút V và các cạnh E. Trong đồ thị dựa trên mối
tương quan, chỉ số chứng khoán hoặc thị trường riêng lẻ được coi là một nút. Đồ thị thị trường
chứng khoán có thể được hình thành dựa trên ma trận khoảng cách.
Trong bài nghiên cứu này, chúng tôi sử dụng phương pháp khoảng cách đo lường sự tương
đồng giữa chuỗi thời gian dưới dạng khoảng cách dựa trên hệ số tương quan Pearson (còn
được gọi là khoảng cách Pearson): dij= 2(1-ij with
(Y Y )−(Y )(Y ) ρ (∆ t )= i j i j ij
√(Y2)−(Y 2)√(Y2)−(Y2) i i j j
where i and j are the numerical labels of stocks, where Y =ln P (t )−ln P (t −1) and P (t) is the i i i i
closure price of the stock i at the day t. The statistical average is a temporal average performed
on all the trading days of the investigated time period.
We determine the n × n matrix of correlation coefficients for daily logarithm price differences
(which almost coincides with returns). By definition, ρ can vary from -1 (completely anti- ij
correlated pair of stocks) to 1 (completely correlated pair of stocks). When ρ = 0 the two ij
stocks are uncorrelated. The matrix of correlation coefficient is a symmetric matrix with n(n−1)
ρ (∆ t )=1 in the main diagonal. Hence for each value of ∆ t , =4950 correlation i j 2
coefficients characterize each correlation coefficient matrix completely.
With this choice d (∆ t )fulfills the three axioms of a metric: ij (i)
d (∆ t )=0 if and only if i= j; ij (ii)
d (∆ t )=d ( ∆ t ) ij j i (iii)
d (∆ t ) ≤ d ( ∆t) +d ( ∆ t) ij i k kj 2.1
In this approach, an adjacency matrix is constructed by applying a threshold value in the
correlation or distance d of the network. The main idea of the threshold method is as follows. ij
It filters out the strongest correlations (or shortest distances) by putting a certain value of
threshold and discard the remaining correlations/distances. The stocks represent the vertices of
the network. We specify a certain threshold value θ ,−1≤ θ ≤1. Hence, if the correlation
coefficient is greater than or equal to θ, we add an undirected edge connecting the vertices i
and j. So, different values of θ define the networks with the same set of vertices, but different sets of edges. 2.2
Mimimum Spanning Tree (MST) is constructed by using the distances d , which represent the ij
interaction strengths (correlations) between pairs of stocks i , j=1 , .. . , n in a market for a
specific time window, such that all n nodes (stocks) are connected with exactly (n−1¿ edges
under the constraint that total distance is minimum. n=¿ V ∨¿
Algorithms due to Kruskal or Prim are generally utilized to obtain MST from a distance matrix.
The MST allows to obtain, in a direct and essentially unique way, the subdominant ultrametric
distance matrix D¿ (∆ t) and the hierarchical organization of the elements (stocks in our case) of the investigated data set.
In this work, we use the method of constructing a MST linking a set of n objects is direct and it is
known in multivariate analysis as the nearest neighbor single linkage cluster analysis. The
subdominant ultrametric distance between ¿
i and j objects, i.e. the element d of the ij D¿ (∆ t) is
the maximum value of the metric distance detected by moving in single steps from i to j
through the path connecting iand j in the MST. Phân cụm
Hierarchical clustering is one of the clustering methods for observing groups of objects based on
their distance from each other. The cluster distance between two objects can be calculated in
many ways, such as single linkage, complete linkage, and Ward’s linkage. After all pairs of cluster
distances have been computed, they are matched in a hierarchical tree structure from a pair
with the lowest cluster distance to one with the highest cluster distance. We use Ward’s linkage
to compute the distance between clusters of stocks in this work, which can be calculated as n d
(r , s )=√2nr s‖x −x‖ clust n +n r s r s
where ‖∙‖ is the distance. x and x are the centroids of the clusters r and s, respectively. nr and r s
n denote the numbers of elements in the clusters r and s, respectively. The distance between s
stocks is represented as a matrix; for example, stock 1 has a distance 0 from itself, 0.2 from stock
2, and 0.9 from stock 3. Using the Ward’s linkage, the distance cluster between stocks 1 and 2
yields 0.412, which is the minimum cluster distance of all pairs. As a consequence, the first link
is established between stocks 1 and 2. Following that, a distance cluster of 1.391 is obtained
from the centroid of the stocks 1 and 2 to the stock 3. If there are more stocks, then the
procedure is continued until all stocks have been exhausted.
We can verify the cluster tree by using inconsistency coefficients which is defined as the
difference between considered link height and the average of the heights of all prior links,
including itself, normalized by standard deviation of all heights. For example, the inconsistency
coefficient of link 2 is equal [1.391 − (mean(0.412,1.391)]/std(0.412,1.391) = 0.707. Stocks 1 and
2 are in cluster 1, while stock 3 is in cluster 2 if the hierarchical clustering is cut off due to this
level inconsistency. Conversely, all stocks would be in the same cluster if the cut off is greater than 0.707.
Then, the hierarchical tree structure with the distance can be constructed and determined the
minimum spanning tree in different economic circumstances.
Việc phát hiện các cộng đồng hoặc cụm có tầm quan trọng lớn đối với bất kỳ nghiên cứu dựa
trên biểu đồ nào và thị trường chứng khoán cũng không khác. Phân cụm biểu đồ thị trường
chứng khoán có thể giúp lấy thông tin kinh tế có ý nghĩa. Nó cũng có thể giúp tối ưu hóa danh
mục đầu tư bằng cách xác định các loại tài sản ít tương quan hơn.
Kỹ thuật phân cụm theo cấp bậc cho thấy cấu trúc đa cấp của biểu đồ bằng cách hợp nhất đệ
quy các nút hoặc cụm và được sử dụng rộng rãi để phát hiện các cụm trong biểu đồ thị trường
chứng khoán. Độ tương tự được đo bằng các thước đo khoảng cách khác nhau, chẳng hạn như
thước đo được xác định trong phương trình:
Giá trị tối thiểu của dij sẽ là 0 (khi pij = 1) và giá trị tối đa sẽ là 2 (khi pij = −1). Do đó, giá trị dij
cao hơn có nghĩa là mối tương quan tích cực ít hơn hoặc tương quan tiêu cực hơn giữa các cặp cổ phiếu.